کد مقاله | کد نشریه | سال انتشار | مقاله انگلیسی | نسخه تمام متن |
---|---|---|---|---|
409829 | 679093 | 2012 | 12 صفحه PDF | دانلود رایگان |
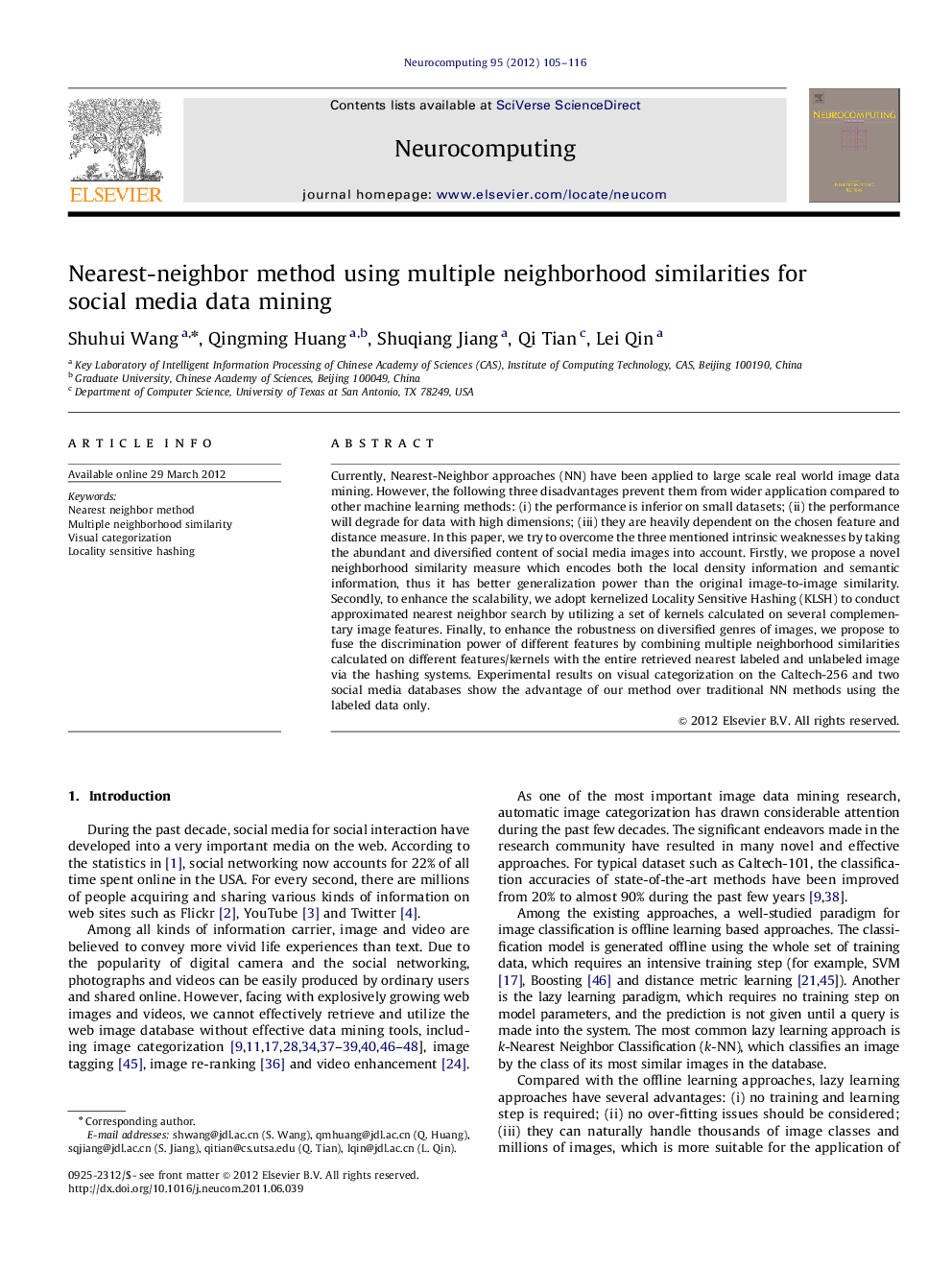
Currently, Nearest-Neighbor approaches (NN) have been applied to large scale real world image data mining. However, the following three disadvantages prevent them from wider application compared to other machine learning methods: (i) the performance is inferior on small datasets; (ii) the performance will degrade for data with high dimensions; (iii) they are heavily dependent on the chosen feature and distance measure. In this paper, we try to overcome the three mentioned intrinsic weaknesses by taking the abundant and diversified content of social media images into account. Firstly, we propose a novel neighborhood similarity measure which encodes both the local density information and semantic information, thus it has better generalization power than the original image-to-image similarity. Secondly, to enhance the scalability, we adopt kernelized Locality Sensitive Hashing (KLSH) to conduct approximated nearest neighbor search by utilizing a set of kernels calculated on several complementary image features. Finally, to enhance the robustness on diversified genres of images, we propose to fuse the discrimination power of different features by combining multiple neighborhood similarities calculated on different features/kernels with the entire retrieved nearest labeled and unlabeled image via the hashing systems. Experimental results on visual categorization on the Caltech-256 and two social media databases show the advantage of our method over traditional NN methods using the labeled data only.
Journal: Neurocomputing - Volume 95, 15 October 2012, Pages 105–116