کد مقاله | کد نشریه | سال انتشار | مقاله انگلیسی | نسخه تمام متن |
---|---|---|---|---|
410250 | 679132 | 2013 | 12 صفحه PDF | دانلود رایگان |
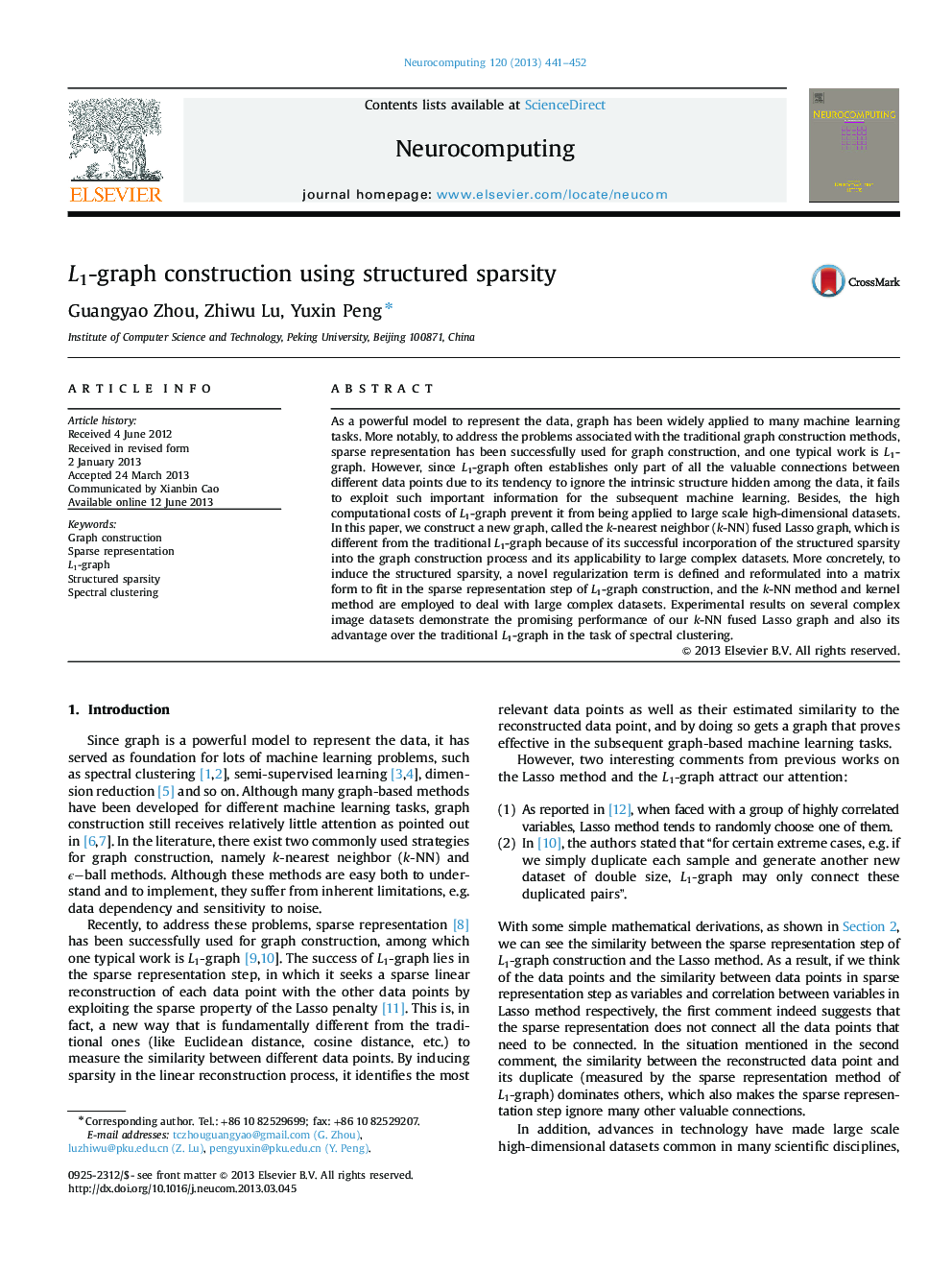
As a powerful model to represent the data, graph has been widely applied to many machine learning tasks. More notably, to address the problems associated with the traditional graph construction methods, sparse representation has been successfully used for graph construction, and one typical work is L1-graph. However, since L1-graph often establishes only part of all the valuable connections between different data points due to its tendency to ignore the intrinsic structure hidden among the data, it fails to exploit such important information for the subsequent machine learning. Besides, the high computational costs of L1-graph prevent it from being applied to large scale high-dimensional datasets. In this paper, we construct a new graph, called the k-nearest neighbor (k-NN) fused Lasso graph, which is different from the traditional L1-graph because of its successful incorporation of the structured sparsity into the graph construction process and its applicability to large complex datasets. More concretely, to induce the structured sparsity, a novel regularization term is defined and reformulated into a matrix form to fit in the sparse representation step of L1-graph construction, and the k-NN method and kernel method are employed to deal with large complex datasets. Experimental results on several complex image datasets demonstrate the promising performance of our k-NN fused Lasso graph and also its advantage over the traditional L1-graph in the task of spectral clustering.
Journal: Neurocomputing - Volume 120, 23 November 2013, Pages 441–452