کد مقاله | کد نشریه | سال انتشار | مقاله انگلیسی | نسخه تمام متن |
---|---|---|---|---|
410541 | 679149 | 2009 | 8 صفحه PDF | دانلود رایگان |
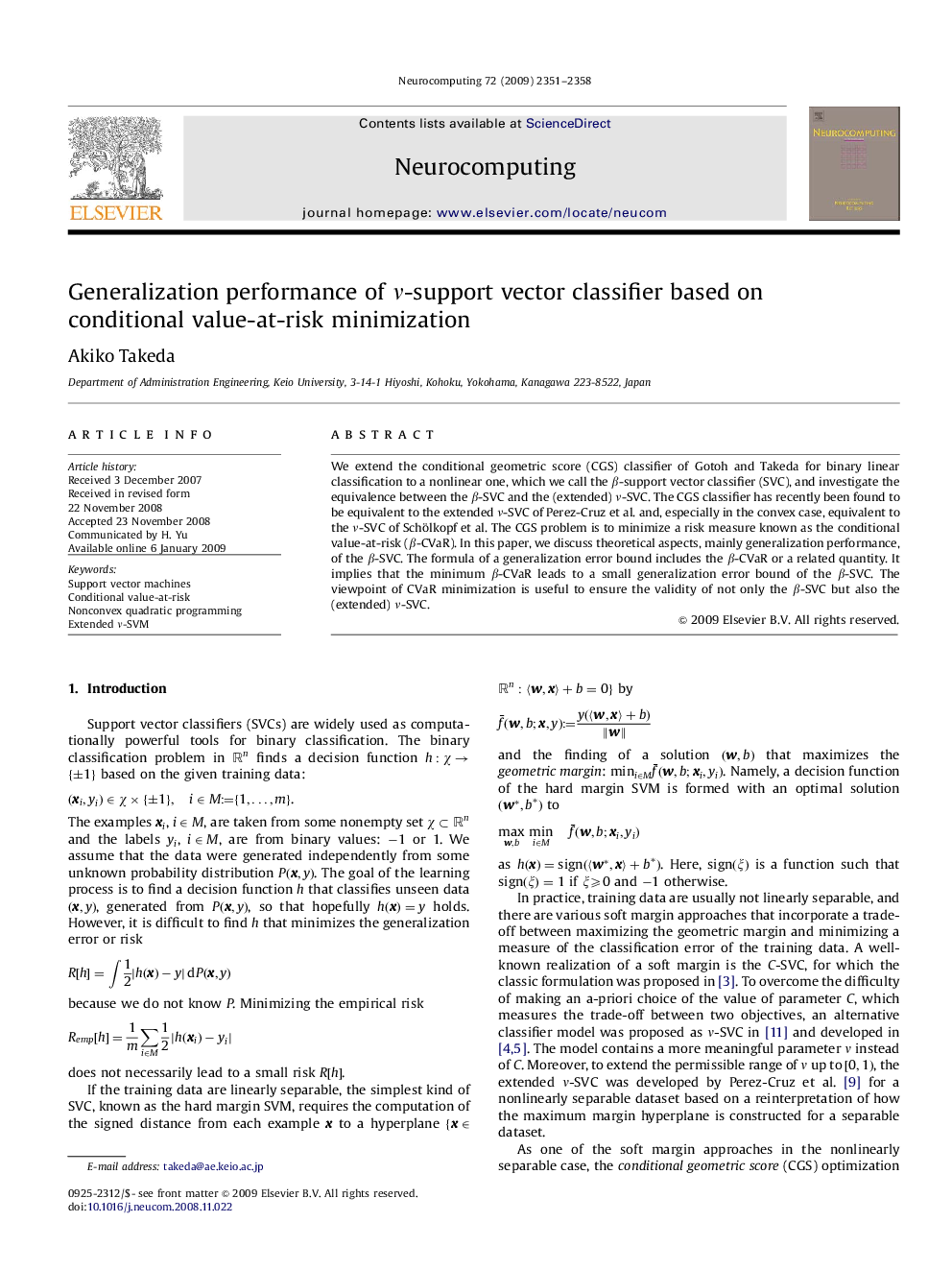
We extend the conditional geometric score (CGS) classifier of Gotoh and Takeda for binary linear classification to a nonlinear one, which we call the ββ-support vector classifier (SVC), and investigate the equivalence between the ββ-SVC and the (extended) νν-SVC. The CGS classifier has recently been found to be equivalent to the extended νν-SVC of Perez-Cruz et al. and, especially in the convex case, equivalent to the νν-SVC of Schölkopf et al. The CGS problem is to minimize a risk measure known as the conditional value-at-risk (ββ-CVaR). In this paper, we discuss theoretical aspects, mainly generalization performance, of the ββ-SVC. The formula of a generalization error bound includes the ββ-CVaR or a related quantity. It implies that the minimum ββ-CVaR leads to a small generalization error bound of the ββ-SVC. The viewpoint of CVaR minimization is useful to ensure the validity of not only the ββ-SVC but also the (extended) νν-SVC.
Journal: Neurocomputing - Volume 72, Issues 10–12, June 2009, Pages 2351–2358