کد مقاله | کد نشریه | سال انتشار | مقاله انگلیسی | نسخه تمام متن |
---|---|---|---|---|
410856 | 679167 | 2011 | 8 صفحه PDF | دانلود رایگان |
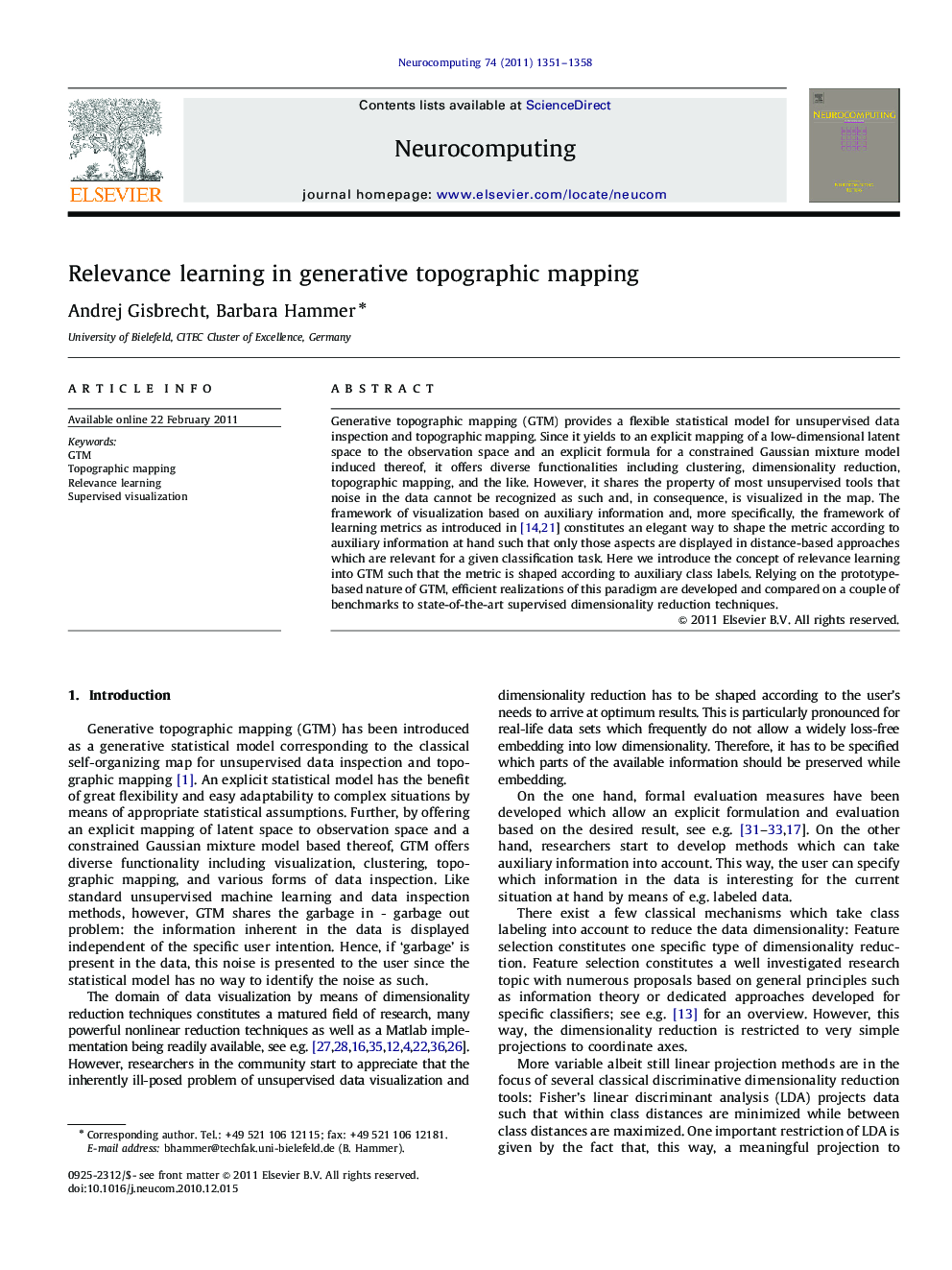
Generative topographic mapping (GTM) provides a flexible statistical model for unsupervised data inspection and topographic mapping. Since it yields to an explicit mapping of a low-dimensional latent space to the observation space and an explicit formula for a constrained Gaussian mixture model induced thereof, it offers diverse functionalities including clustering, dimensionality reduction, topographic mapping, and the like. However, it shares the property of most unsupervised tools that noise in the data cannot be recognized as such and, in consequence, is visualized in the map. The framework of visualization based on auxiliary information and, more specifically, the framework of learning metrics as introduced in [15] and [22] constitutes an elegant way to shape the metric according to auxiliary information at hand such that only those aspects are displayed in distance-based approaches which are relevant for a given classification task. Here we introduce the concept of relevance learning into GTM such that the metric is shaped according to auxiliary class labels. Relying on the prototype-based nature of GTM, efficient realizations of this paradigm are developed and compared on a couple of benchmarks to state-of-the-art supervised dimensionality reduction techniques.
Journal: Neurocomputing - Volume 74, Issue 9, April 2011, Pages 1351–1358