کد مقاله | کد نشریه | سال انتشار | مقاله انگلیسی | نسخه تمام متن |
---|---|---|---|---|
410859 | 679167 | 2011 | 9 صفحه PDF | دانلود رایگان |
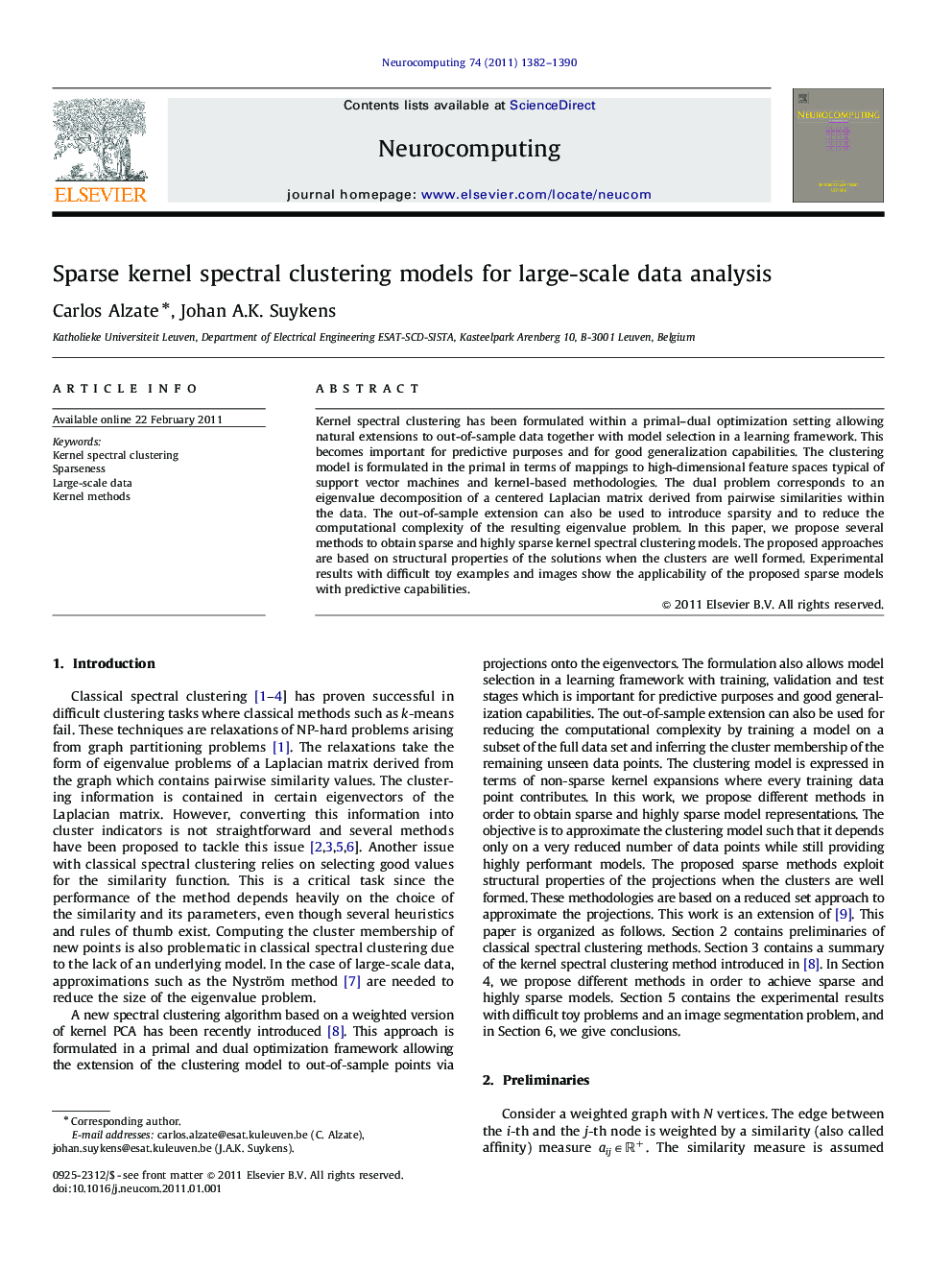
Kernel spectral clustering has been formulated within a primal–dual optimization setting allowing natural extensions to out-of-sample data together with model selection in a learning framework. This becomes important for predictive purposes and for good generalization capabilities. The clustering model is formulated in the primal in terms of mappings to high-dimensional feature spaces typical of support vector machines and kernel-based methodologies. The dual problem corresponds to an eigenvalue decomposition of a centered Laplacian matrix derived from pairwise similarities within the data. The out-of-sample extension can also be used to introduce sparsity and to reduce the computational complexity of the resulting eigenvalue problem. In this paper, we propose several methods to obtain sparse and highly sparse kernel spectral clustering models. The proposed approaches are based on structural properties of the solutions when the clusters are well formed. Experimental results with difficult toy examples and images show the applicability of the proposed sparse models with predictive capabilities.
Journal: Neurocomputing - Volume 74, Issue 9, April 2011, Pages 1382–1390