کد مقاله | کد نشریه | سال انتشار | مقاله انگلیسی | نسخه تمام متن |
---|---|---|---|---|
411645 | 679578 | 2016 | 9 صفحه PDF | دانلود رایگان |
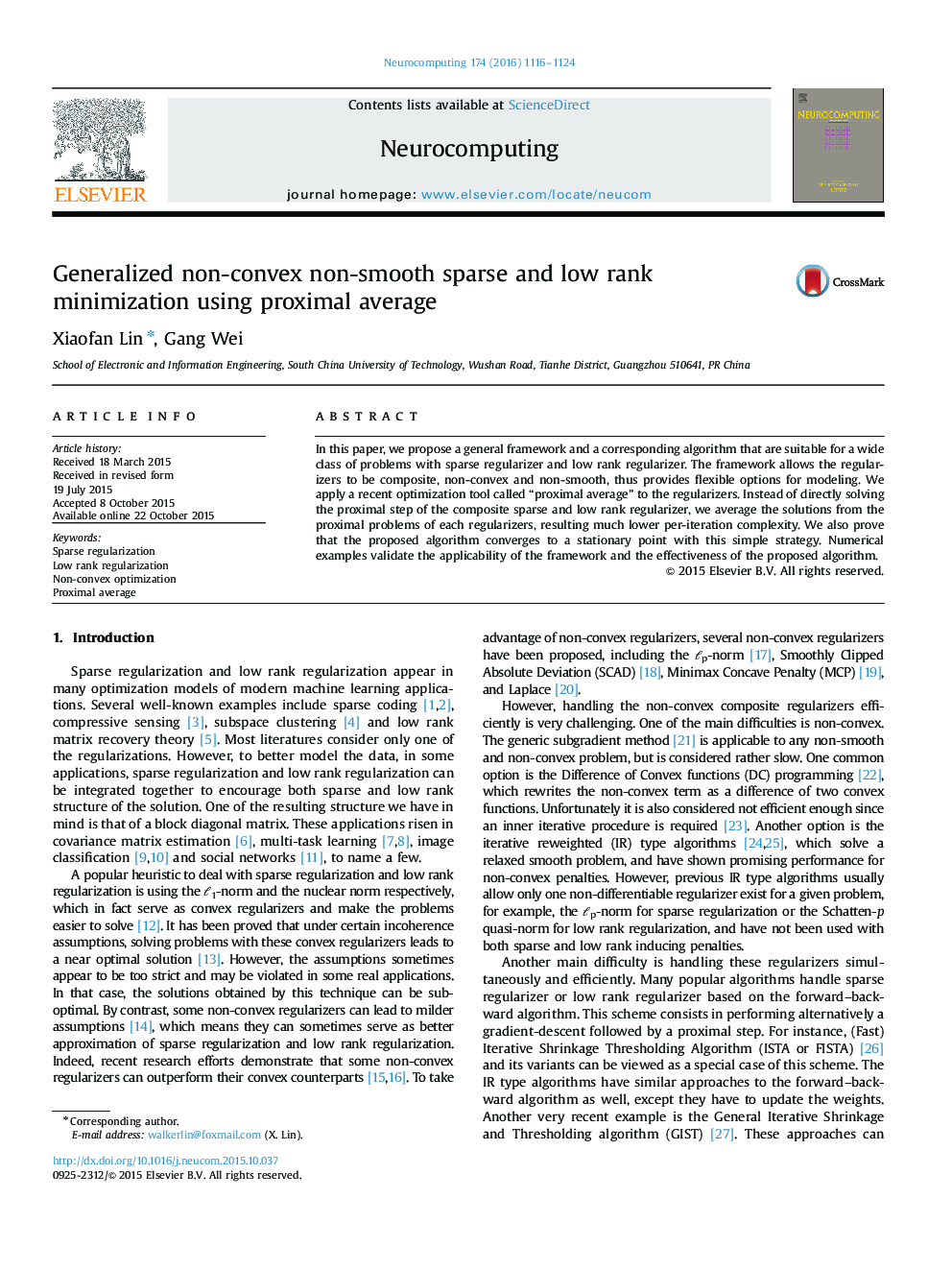
• A general framework for problems with sparse and low rank regularizer.
• Provide an corresponding algorithm to solve the general framework.
• Provide theoretical justification and convergence analysis.
In this paper, we propose a general framework and a corresponding algorithm that are suitable for a wide class of problems with sparse regularizer and low rank regularizer. The framework allows the regularizers to be composite, non-convex and non-smooth, thus provides flexible options for modeling. We apply a recent optimization tool called “proximal average” to the regularizers. Instead of directly solving the proximal step of the composite sparse and low rank regularizer, we average the solutions from the proximal problems of each regularizers, resulting much lower per-iteration complexity. We also prove that the proposed algorithm converges to a stationary point with this simple strategy. Numerical examples validate the applicability of the framework and the effectiveness of the proposed algorithm.
Journal: Neurocomputing - Volume 174, Part B, 22 January 2016, Pages 1116–1124