کد مقاله | کد نشریه | سال انتشار | مقاله انگلیسی | نسخه تمام متن |
---|---|---|---|---|
412286 | 679623 | 2014 | 11 صفحه PDF | دانلود رایگان |
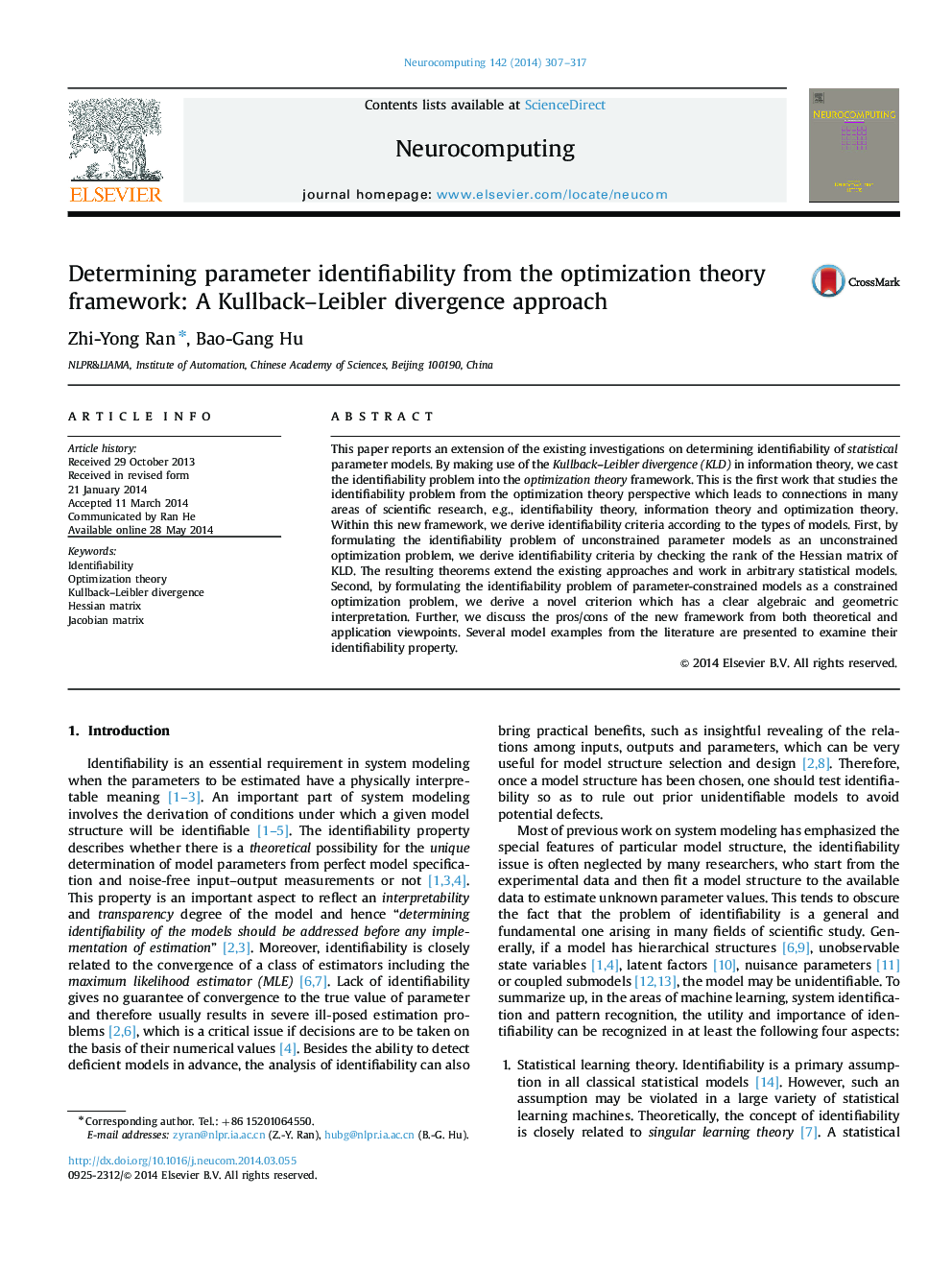
This paper reports an extension of the existing investigations on determining identifiability of statistical parameter models. By making use of the Kullback–Leibler divergence (KLD) in information theory, we cast the identifiability problem into the optimization theory framework. This is the first work that studies the identifiability problem from the optimization theory perspective which leads to connections in many areas of scientific research, e.g., identifiability theory, information theory and optimization theory. Within this new framework, we derive identifiability criteria according to the types of models. First, by formulating the identifiability problem of unconstrained parameter models as an unconstrained optimization problem, we derive identifiability criteria by checking the rank of the Hessian matrix of KLD. The resulting theorems extend the existing approaches and work in arbitrary statistical models. Second, by formulating the identifiability problem of parameter-constrained models as a constrained optimization problem, we derive a novel criterion which has a clear algebraic and geometric interpretation. Further, we discuss the pros/cons of the new framework from both theoretical and application viewpoints. Several model examples from the literature are presented to examine their identifiability property.
Journal: Neurocomputing - Volume 142, 22 October 2014, Pages 307–317