کد مقاله | کد نشریه | سال انتشار | مقاله انگلیسی | نسخه تمام متن |
---|---|---|---|---|
412506 | 679647 | 2012 | 7 صفحه PDF | دانلود رایگان |
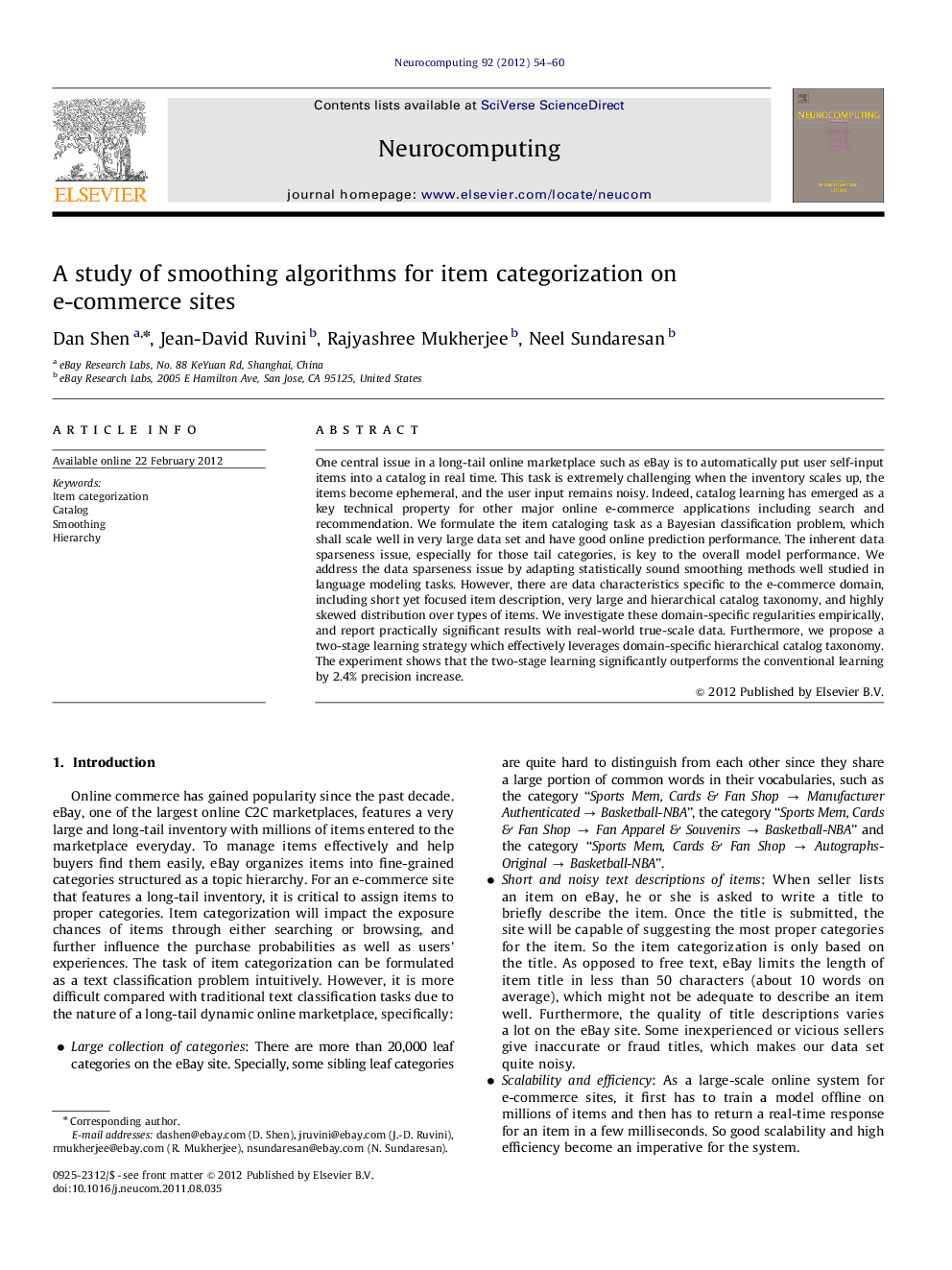
One central issue in a long-tail online marketplace such as eBay is to automatically put user self-input items into a catalog in real time. This task is extremely challenging when the inventory scales up, the items become ephemeral, and the user input remains noisy. Indeed, catalog learning has emerged as a key technical property for other major online e-commerce applications including search and recommendation. We formulate the item cataloging task as a Bayesian classification problem, which shall scale well in very large data set and have good online prediction performance. The inherent data sparseness issue, especially for those tail categories, is key to the overall model performance. We address the data sparseness issue by adapting statistically sound smoothing methods well studied in language modeling tasks. However, there are data characteristics specific to the e-commerce domain, including short yet focused item description, very large and hierarchical catalog taxonomy, and highly skewed distribution over types of items. We investigate these domain-specific regularities empirically, and report practically significant results with real-world true-scale data. Furthermore, we propose a two-stage learning strategy which effectively leverages domain-specific hierarchical catalog taxonomy. The experiment shows that the two-stage learning significantly outperforms the conventional learning by 2.4% precision increase.
Journal: Neurocomputing - Volume 92, 1 September 2012, Pages 54–60