کد مقاله | کد نشریه | سال انتشار | مقاله انگلیسی | نسخه تمام متن |
---|---|---|---|---|
412515 | 679647 | 2012 | 11 صفحه PDF | دانلود رایگان |
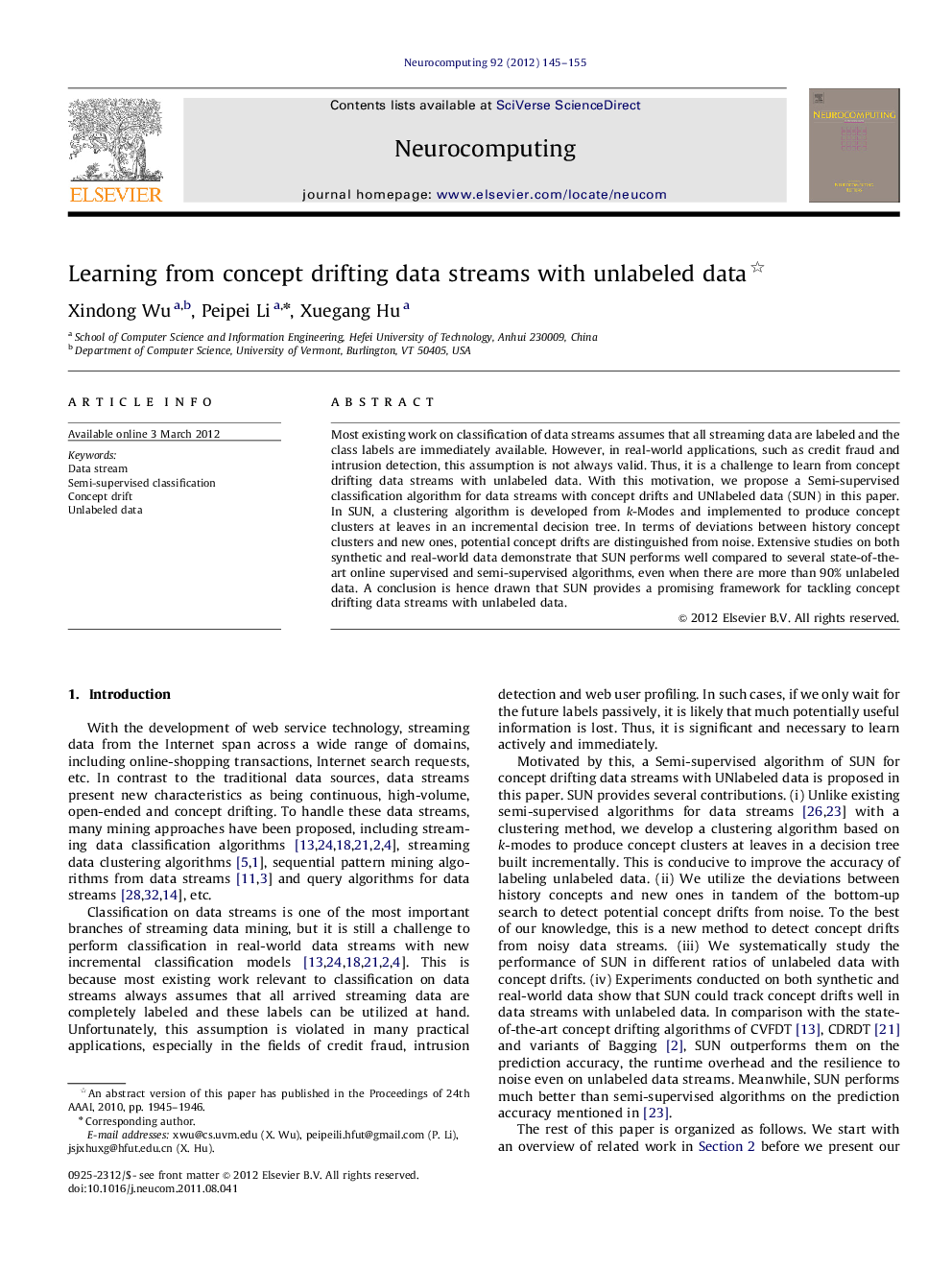
Most existing work on classification of data streams assumes that all streaming data are labeled and the class labels are immediately available. However, in real-world applications, such as credit fraud and intrusion detection, this assumption is not always valid. Thus, it is a challenge to learn from concept drifting data streams with unlabeled data. With this motivation, we propose a Semi-supervised classification algorithm for data streams with concept drifts and UNlabeled data (SUN) in this paper. In SUN, a clustering algorithm is developed from k-Modes and implemented to produce concept clusters at leaves in an incremental decision tree. In terms of deviations between history concept clusters and new ones, potential concept drifts are distinguished from noise. Extensive studies on both synthetic and real-world data demonstrate that SUN performs well compared to several state-of-the-art online supervised and semi-supervised algorithms, even when there are more than 90% unlabeled data. A conclusion is hence drawn that SUN provides a promising framework for tackling concept drifting data streams with unlabeled data.
Journal: Neurocomputing - Volume 92, 1 September 2012, Pages 145–155