کد مقاله | کد نشریه | سال انتشار | مقاله انگلیسی | نسخه تمام متن |
---|---|---|---|---|
412960 | 679708 | 2009 | 7 صفحه PDF | دانلود رایگان |
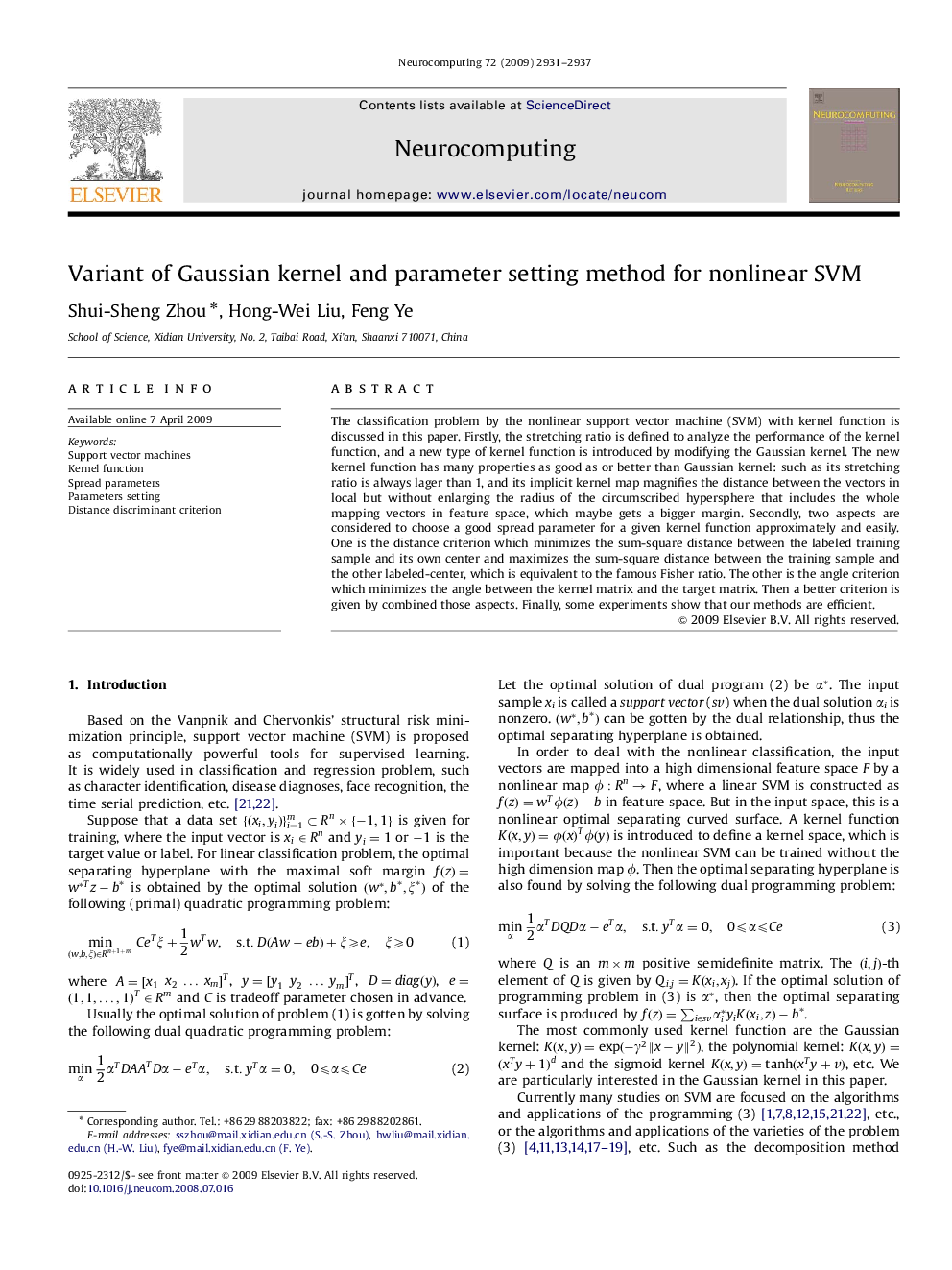
The classification problem by the nonlinear support vector machine (SVM) with kernel function is discussed in this paper. Firstly, the stretching ratio is defined to analyze the performance of the kernel function, and a new type of kernel function is introduced by modifying the Gaussian kernel. The new kernel function has many properties as good as or better than Gaussian kernel: such as its stretching ratio is always lager than 1, and its implicit kernel map magnifies the distance between the vectors in local but without enlarging the radius of the circumscribed hypersphere that includes the whole mapping vectors in feature space, which maybe gets a bigger margin. Secondly, two aspects are considered to choose a good spread parameter for a given kernel function approximately and easily. One is the distance criterion which minimizes the sum-square distance between the labeled training sample and its own center and maximizes the sum-square distance between the training sample and the other labeled-center, which is equivalent to the famous Fisher ratio. The other is the angle criterion which minimizes the angle between the kernel matrix and the target matrix. Then a better criterion is given by combined those aspects. Finally, some experiments show that our methods are efficient.
Journal: Neurocomputing - Volume 72, Issues 13–15, August 2009, Pages 2931–2937