کد مقاله | کد نشریه | سال انتشار | مقاله انگلیسی | نسخه تمام متن |
---|---|---|---|---|
413351 | 680437 | 2015 | 12 صفحه PDF | دانلود رایگان |
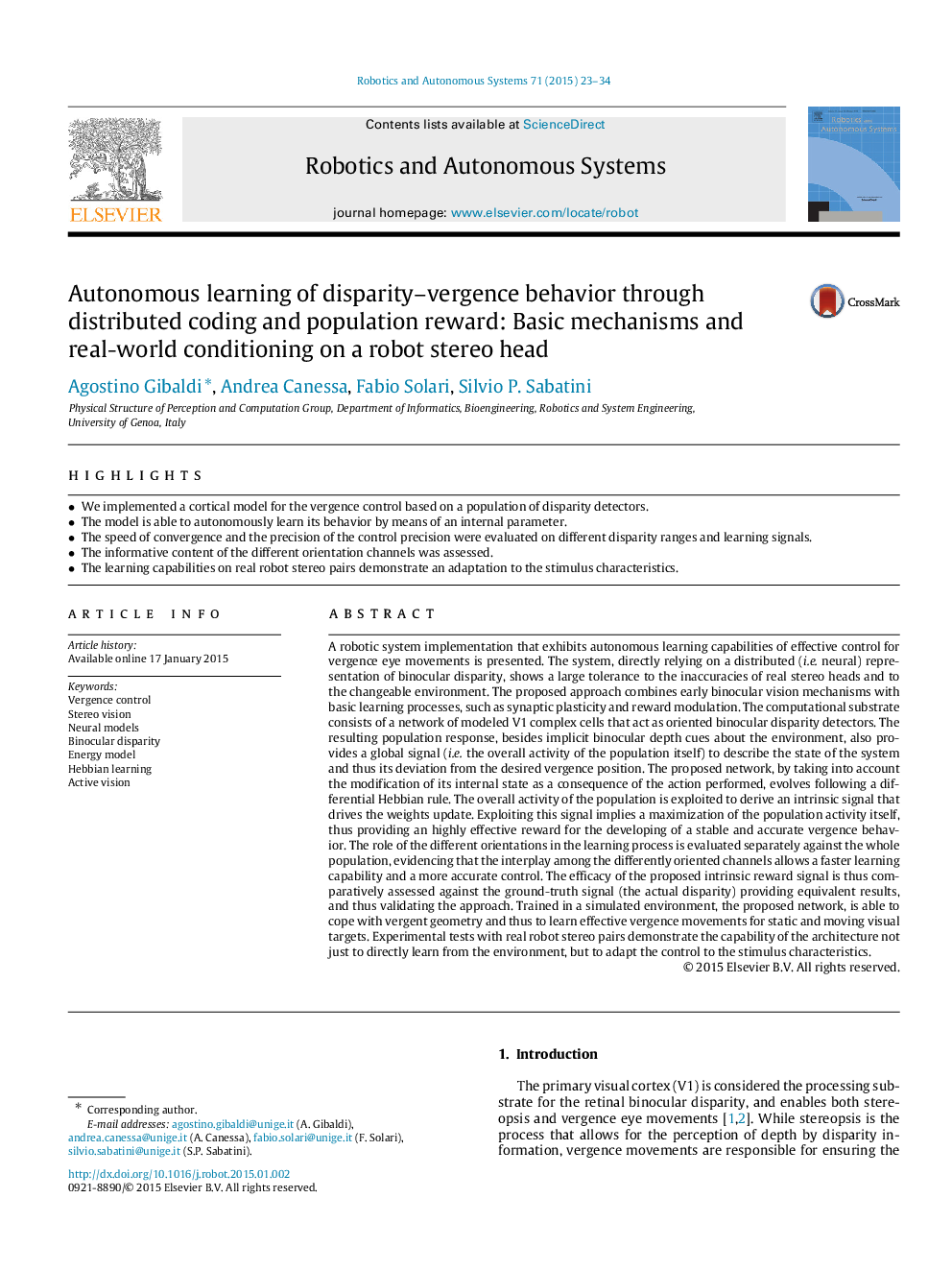
• We implemented a cortical model for the vergence control based on a population of disparity detectors.
• The model is able to autonomously learn its behavior by means of an internal parameter.
• The speed of convergence and the precision of the control precision were evaluated on different disparity ranges and learning signals.
• The informative content of the different orientation channels was assessed.
• The learning capabilities on real robot stereo pairs demonstrate an adaptation to the stimulus characteristics.
A robotic system implementation that exhibits autonomous learning capabilities of effective control for vergence eye movements is presented. The system, directly relying on a distributed (i.e. neural) representation of binocular disparity, shows a large tolerance to the inaccuracies of real stereo heads and to the changeable environment. The proposed approach combines early binocular vision mechanisms with basic learning processes, such as synaptic plasticity and reward modulation. The computational substrate consists of a network of modeled V1 complex cells that act as oriented binocular disparity detectors. The resulting population response, besides implicit binocular depth cues about the environment, also provides a global signal (i.e. the overall activity of the population itself) to describe the state of the system and thus its deviation from the desired vergence position. The proposed network, by taking into account the modification of its internal state as a consequence of the action performed, evolves following a differential Hebbian rule. The overall activity of the population is exploited to derive an intrinsic signal that drives the weights update. Exploiting this signal implies a maximization of the population activity itself, thus providing an highly effective reward for the developing of a stable and accurate vergence behavior. The role of the different orientations in the learning process is evaluated separately against the whole population, evidencing that the interplay among the differently oriented channels allows a faster learning capability and a more accurate control. The efficacy of the proposed intrinsic reward signal is thus comparatively assessed against the ground-truth signal (the actual disparity) providing equivalent results, and thus validating the approach. Trained in a simulated environment, the proposed network, is able to cope with vergent geometry and thus to learn effective vergence movements for static and moving visual targets. Experimental tests with real robot stereo pairs demonstrate the capability of the architecture not just to directly learn from the environment, but to adapt the control to the stimulus characteristics.
Journal: Robotics and Autonomous Systems - Volume 71, September 2015, Pages 23–34