کد مقاله | کد نشریه | سال انتشار | مقاله انگلیسی | نسخه تمام متن |
---|---|---|---|---|
415080 | 681168 | 2011 | 10 صفحه PDF | دانلود رایگان |
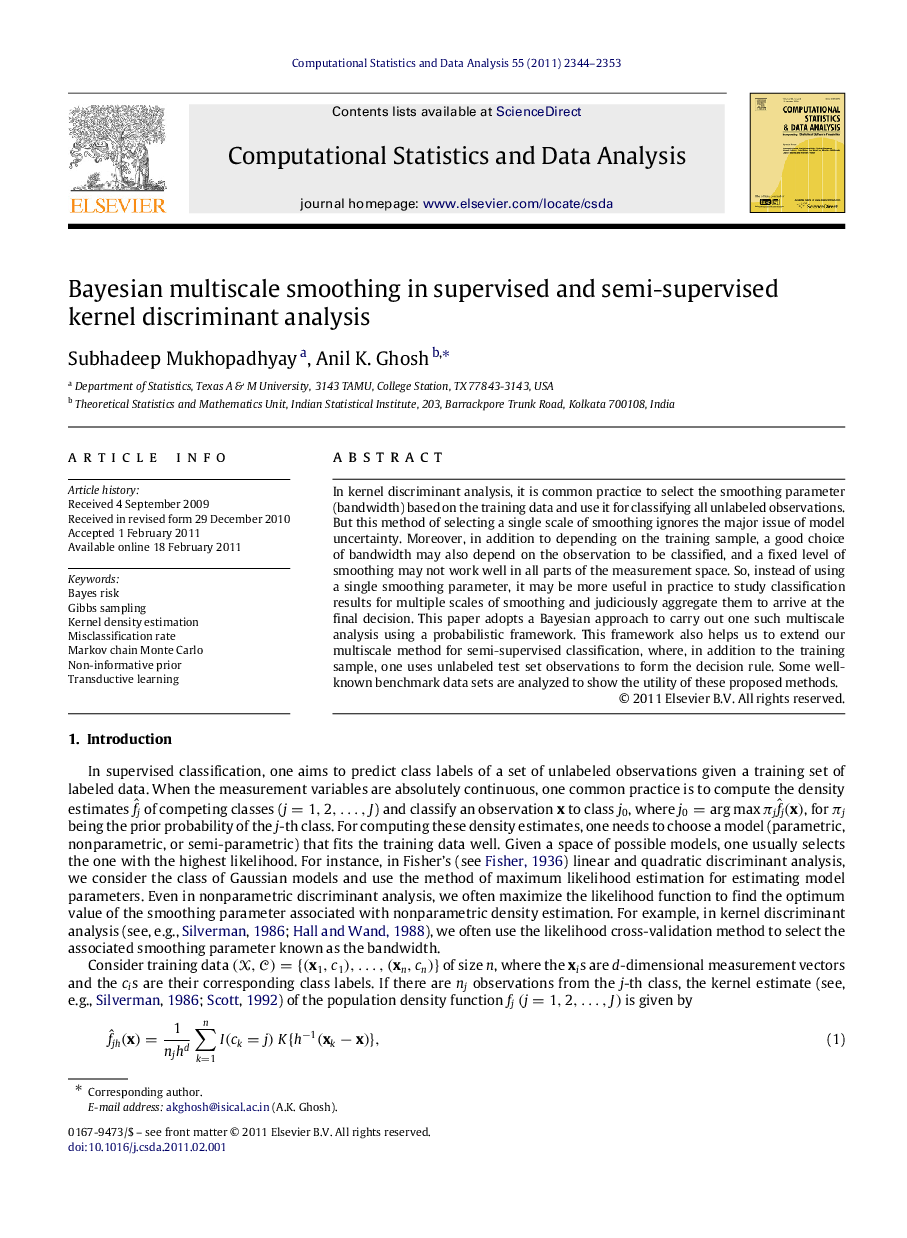
In kernel discriminant analysis, it is common practice to select the smoothing parameter (bandwidth) based on the training data and use it for classifying all unlabeled observations. But this method of selecting a single scale of smoothing ignores the major issue of model uncertainty. Moreover, in addition to depending on the training sample, a good choice of bandwidth may also depend on the observation to be classified, and a fixed level of smoothing may not work well in all parts of the measurement space. So, instead of using a single smoothing parameter, it may be more useful in practice to study classification results for multiple scales of smoothing and judiciously aggregate them to arrive at the final decision. This paper adopts a Bayesian approach to carry out one such multiscale analysis using a probabilistic framework. This framework also helps us to extend our multiscale method for semi-supervised classification, where, in addition to the training sample, one uses unlabeled test set observations to form the decision rule. Some well-known benchmark data sets are analyzed to show the utility of these proposed methods.
Journal: Computational Statistics & Data Analysis - Volume 55, Issue 7, 1 July 2011, Pages 2344–2353