کد مقاله | کد نشریه | سال انتشار | مقاله انگلیسی | نسخه تمام متن |
---|---|---|---|---|
416854 | 681409 | 2012 | 23 صفحه PDF | دانلود رایگان |
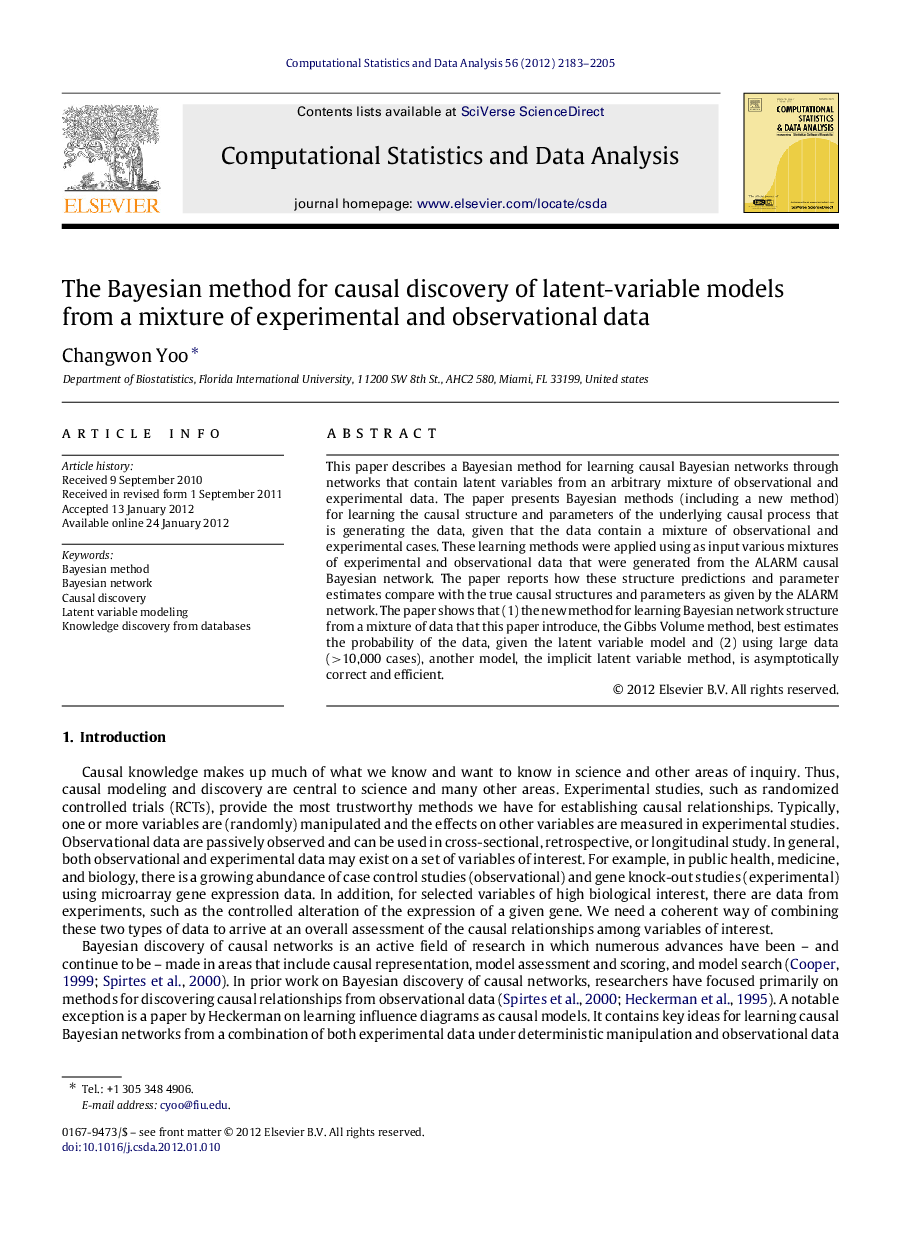
This paper describes a Bayesian method for learning causal Bayesian networks through networks that contain latent variables from an arbitrary mixture of observational and experimental data. The paper presents Bayesian methods (including a new method) for learning the causal structure and parameters of the underlying causal process that is generating the data, given that the data contain a mixture of observational and experimental cases. These learning methods were applied using as input various mixtures of experimental and observational data that were generated from the ALARM causal Bayesian network. The paper reports how these structure predictions and parameter estimates compare with the true causal structures and parameters as given by the ALARM network. The paper shows that (1) the new method for learning Bayesian network structure from a mixture of data that this paper introduce, the Gibbs Volume method, best estimates the probability of the data, given the latent variable model and (2) using large data (>10,000 cases), another model, the implicit latent variable method, is asymptotically correct and efficient.
Journal: Computational Statistics & Data Analysis - Volume 56, Issue 7, July 2012, Pages 2183–2205