کد مقاله | کد نشریه | سال انتشار | مقاله انگلیسی | نسخه تمام متن |
---|---|---|---|---|
417681 | 681560 | 2011 | 17 صفحه PDF | دانلود رایگان |
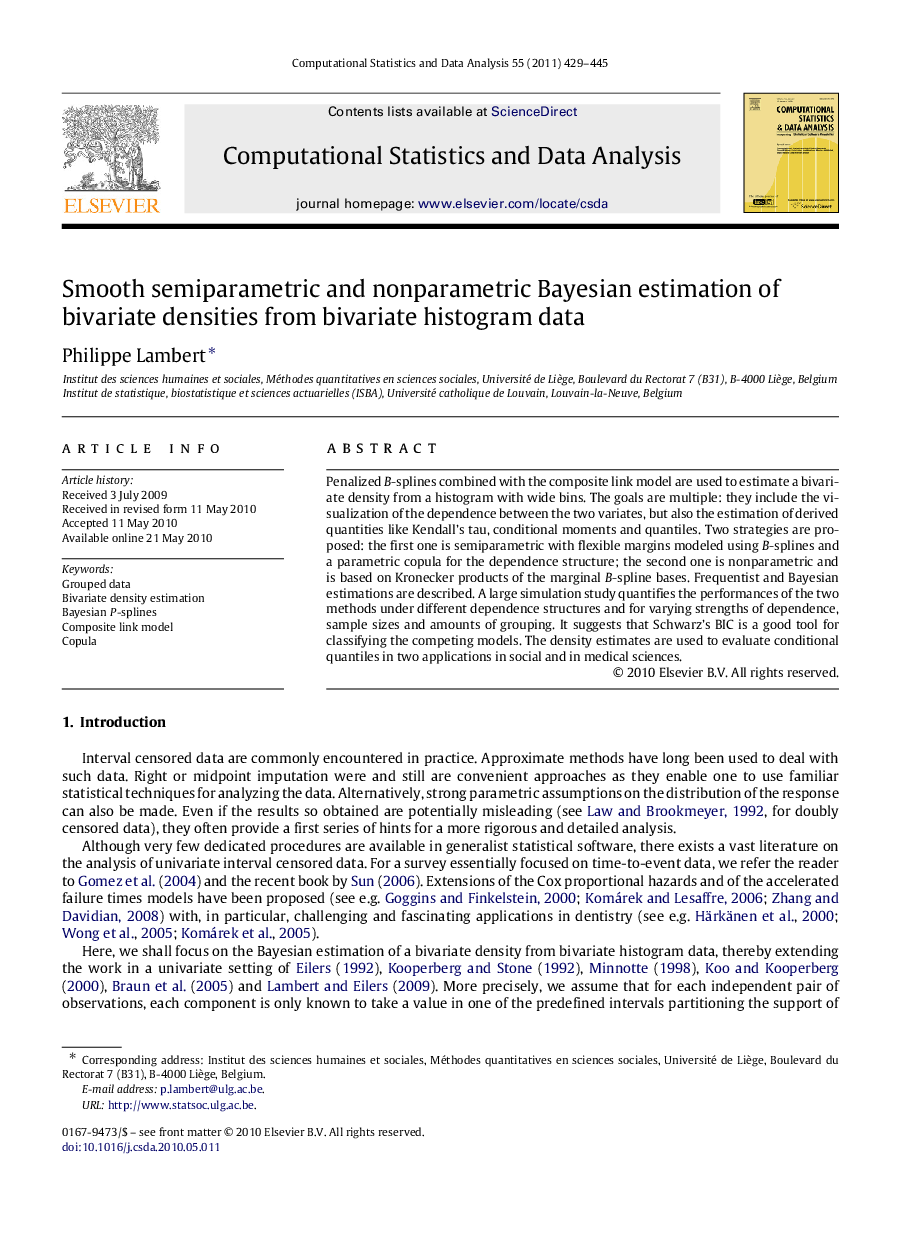
Penalized BB-splines combined with the composite link model are used to estimate a bivariate density from a histogram with wide bins. The goals are multiple: they include the visualization of the dependence between the two variates, but also the estimation of derived quantities like Kendall’s tau, conditional moments and quantiles. Two strategies are proposed: the first one is semiparametric with flexible margins modeled using BB-splines and a parametric copula for the dependence structure; the second one is nonparametric and is based on Kronecker products of the marginal BB-spline bases. Frequentist and Bayesian estimations are described. A large simulation study quantifies the performances of the two methods under different dependence structures and for varying strengths of dependence, sample sizes and amounts of grouping. It suggests that Schwarz’s BIC is a good tool for classifying the competing models. The density estimates are used to evaluate conditional quantiles in two applications in social and in medical sciences.
Journal: Computational Statistics & Data Analysis - Volume 55, Issue 1, 1 January 2011, Pages 429–445