کد مقاله | کد نشریه | سال انتشار | مقاله انگلیسی | نسخه تمام متن |
---|---|---|---|---|
417709 | 681560 | 2011 | 13 صفحه PDF | دانلود رایگان |
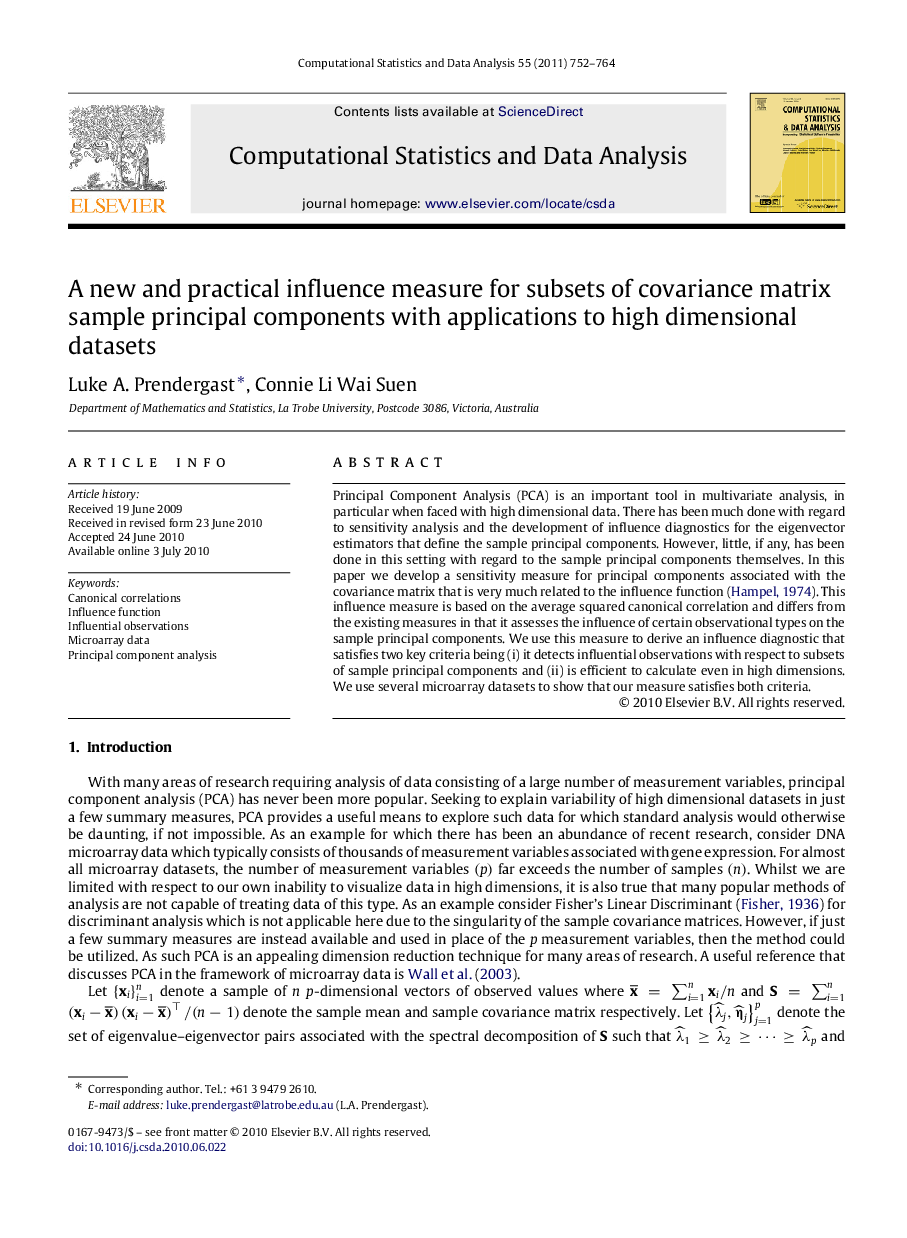
Principal Component Analysis (PCA) is an important tool in multivariate analysis, in particular when faced with high dimensional data. There has been much done with regard to sensitivity analysis and the development of influence diagnostics for the eigenvector estimators that define the sample principal components. However, little, if any, has been done in this setting with regard to the sample principal components themselves. In this paper we develop a sensitivity measure for principal components associated with the covariance matrix that is very much related to the influence function (Hampel, 1974). This influence measure is based on the average squared canonical correlation and differs from the existing measures in that it assesses the influence of certain observational types on the sample principal components. We use this measure to derive an influence diagnostic that satisfies two key criteria being (i) it detects influential observations with respect to subsets of sample principal components and (ii) is efficient to calculate even in high dimensions. We use several microarray datasets to show that our measure satisfies both criteria.
Journal: Computational Statistics & Data Analysis - Volume 55, Issue 1, 1 January 2011, Pages 752–764