کد مقاله | کد نشریه | سال انتشار | مقاله انگلیسی | نسخه تمام متن |
---|---|---|---|---|
417779 | 681579 | 2010 | 12 صفحه PDF | دانلود رایگان |
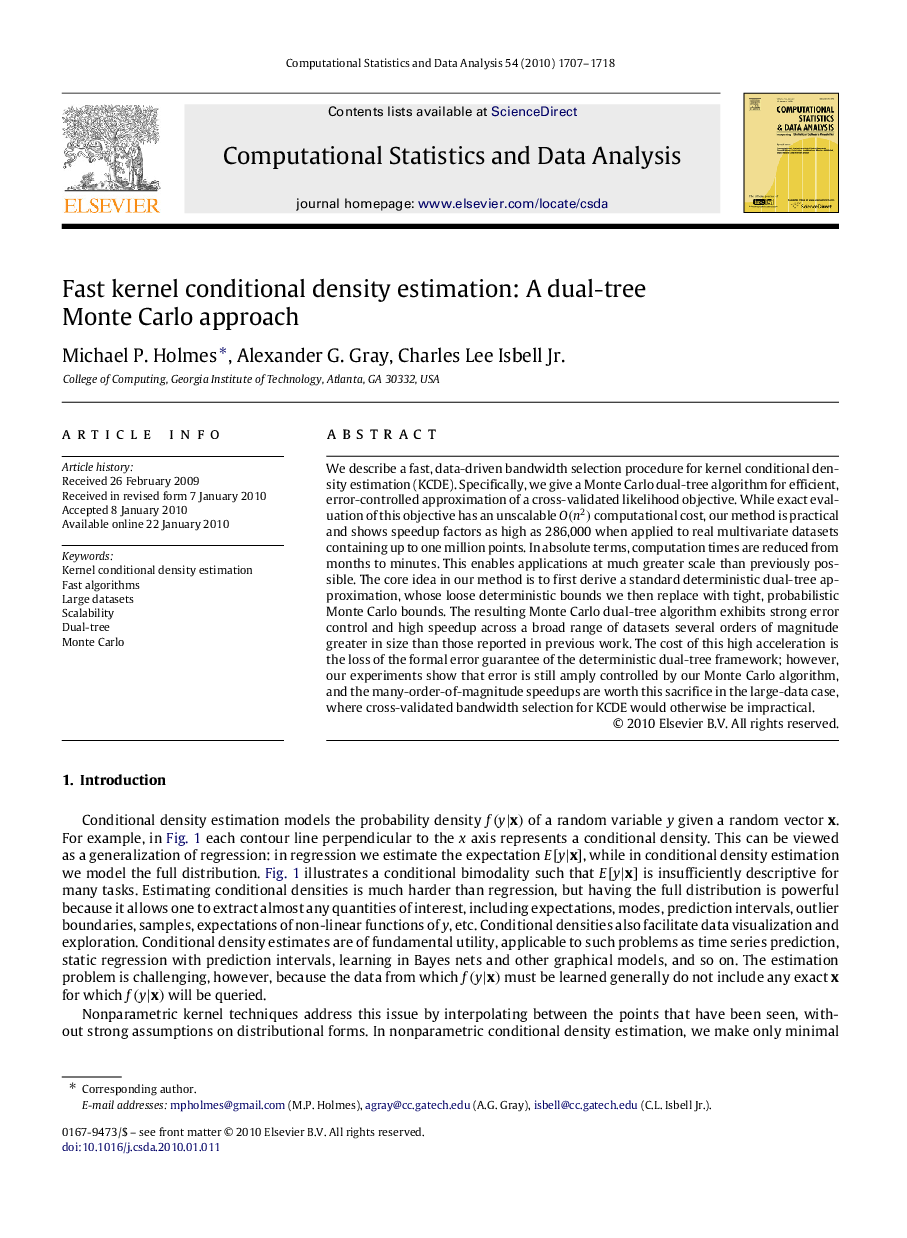
We describe a fast, data-driven bandwidth selection procedure for kernel conditional density estimation (KCDE). Specifically, we give a Monte Carlo dual-tree algorithm for efficient, error-controlled approximation of a cross-validated likelihood objective. While exact evaluation of this objective has an unscalable O(n2)O(n2) computational cost, our method is practical and shows speedup factors as high as 286,000 when applied to real multivariate datasets containing up to one million points. In absolute terms, computation times are reduced from months to minutes. This enables applications at much greater scale than previously possible. The core idea in our method is to first derive a standard deterministic dual-tree approximation, whose loose deterministic bounds we then replace with tight, probabilistic Monte Carlo bounds. The resulting Monte Carlo dual-tree algorithm exhibits strong error control and high speedup across a broad range of datasets several orders of magnitude greater in size than those reported in previous work. The cost of this high acceleration is the loss of the formal error guarantee of the deterministic dual-tree framework; however, our experiments show that error is still amply controlled by our Monte Carlo algorithm, and the many-order-of-magnitude speedups are worth this sacrifice in the large-data case, where cross-validated bandwidth selection for KCDE would otherwise be impractical.
Journal: Computational Statistics & Data Analysis - Volume 54, Issue 7, 1 July 2010, Pages 1707–1718