کد مقاله | کد نشریه | سال انتشار | مقاله انگلیسی | نسخه تمام متن |
---|---|---|---|---|
425262 | 685710 | 2014 | 20 صفحه PDF | دانلود رایگان |
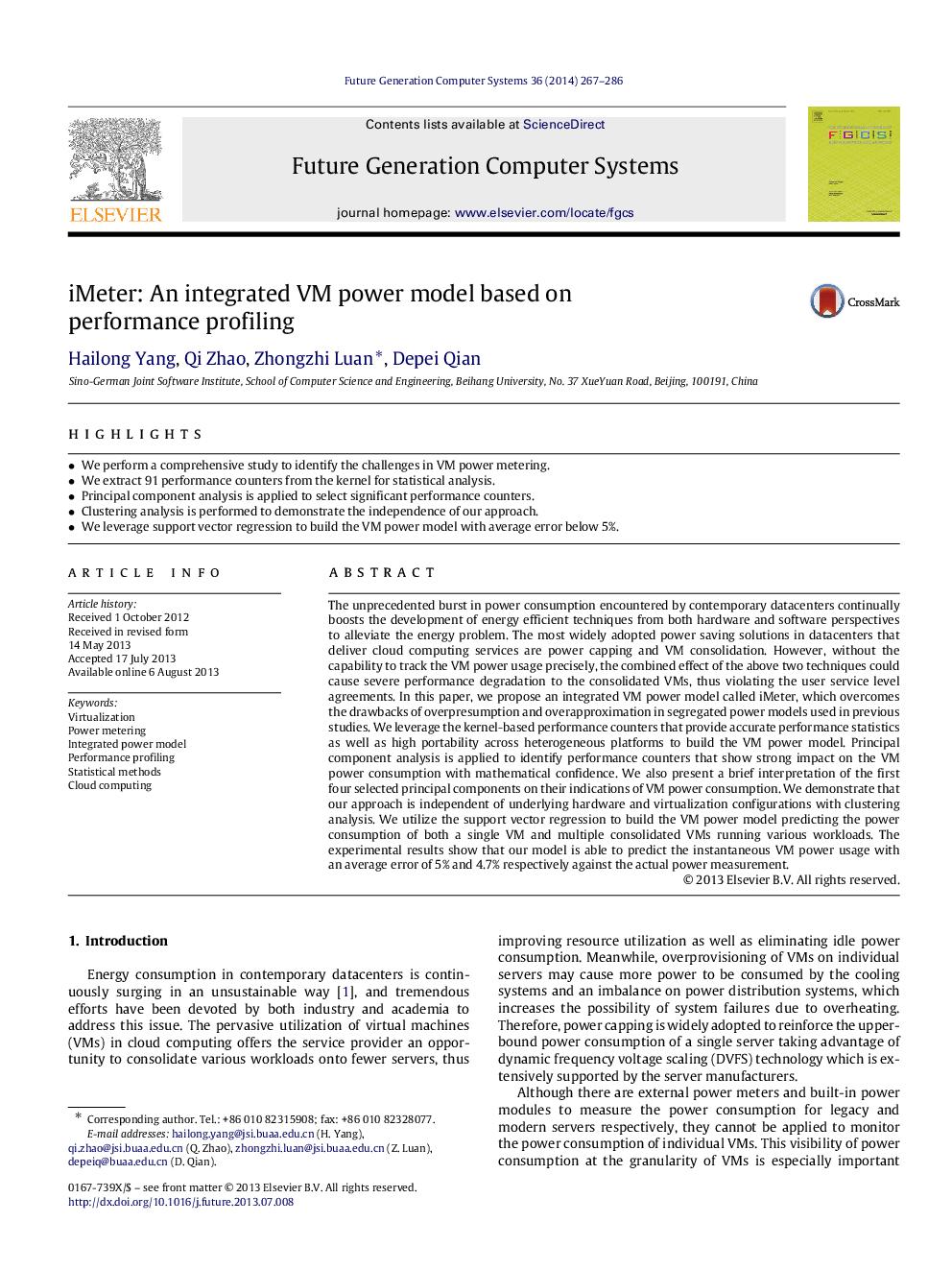
• We perform a comprehensive study to identify the challenges in VM power metering.
• We extract 91 performance counters from the kernel for statistical analysis.
• Principal component analysis is applied to select significant performance counters.
• Clustering analysis is performed to demonstrate the independence of our approach.
• We leverage support vector regression to build the VM power model with average error below 5%.
The unprecedented burst in power consumption encountered by contemporary datacenters continually boosts the development of energy efficient techniques from both hardware and software perspectives to alleviate the energy problem. The most widely adopted power saving solutions in datacenters that deliver cloud computing services are power capping and VM consolidation. However, without the capability to track the VM power usage precisely, the combined effect of the above two techniques could cause severe performance degradation to the consolidated VMs, thus violating the user service level agreements. In this paper, we propose an integrated VM power model called iMeter, which overcomes the drawbacks of overpresumption and overapproximation in segregated power models used in previous studies. We leverage the kernel-based performance counters that provide accurate performance statistics as well as high portability across heterogeneous platforms to build the VM power model. Principal component analysis is applied to identify performance counters that show strong impact on the VM power consumption with mathematical confidence. We also present a brief interpretation of the first four selected principal components on their indications of VM power consumption. We demonstrate that our approach is independent of underlying hardware and virtualization configurations with clustering analysis. We utilize the support vector regression to build the VM power model predicting the power consumption of both a single VM and multiple consolidated VMs running various workloads. The experimental results show that our model is able to predict the instantaneous VM power usage with an average error of 5% and 4.7% respectively against the actual power measurement.
Journal: Future Generation Computer Systems - Volume 36, July 2014, Pages 267–286