کد مقاله | کد نشریه | سال انتشار | مقاله انگلیسی | نسخه تمام متن |
---|---|---|---|---|
4334925 | 1614623 | 2015 | 22 صفحه PDF | دانلود رایگان |
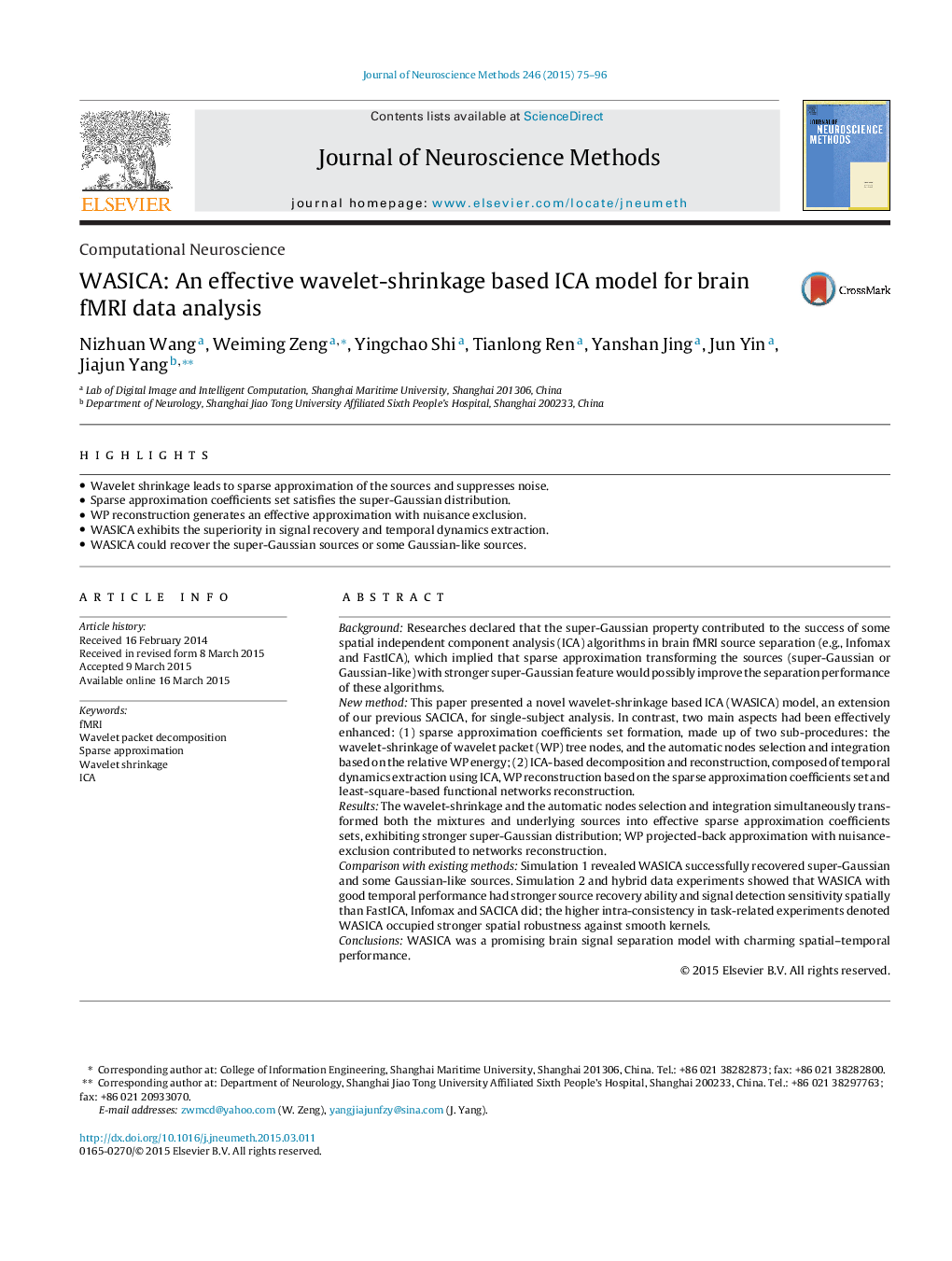
• Wavelet shrinkage leads to sparse approximation of the sources and suppresses noise.
• Sparse approximation coefficients set satisfies the super-Gaussian distribution.
• WP reconstruction generates an effective approximation with nuisance exclusion.
• WASICA exhibits the superiority in signal recovery and temporal dynamics extraction.
• WASICA could recover the super-Gaussian sources or some Gaussian-like sources.
BackgroundResearches declared that the super-Gaussian property contributed to the success of some spatial independent component analysis (ICA) algorithms in brain fMRI source separation (e.g., Infomax and FastICA), which implied that sparse approximation transforming the sources (super-Gaussian or Gaussian-like) with stronger super-Gaussian feature would possibly improve the separation performance of these algorithms.New methodThis paper presented a novel wavelet-shrinkage based ICA (WASICA) model, an extension of our previous SACICA, for single-subject analysis. In contrast, two main aspects had been effectively enhanced: (1) sparse approximation coefficients set formation, made up of two sub-procedures: the wavelet-shrinkage of wavelet packet (WP) tree nodes, and the automatic nodes selection and integration based on the relative WP energy; (2) ICA-based decomposition and reconstruction, composed of temporal dynamics extraction using ICA, WP reconstruction based on the sparse approximation coefficients set and least-square-based functional networks reconstruction.ResultsThe wavelet-shrinkage and the automatic nodes selection and integration simultaneously transformed both the mixtures and underlying sources into effective sparse approximation coefficients sets, exhibiting stronger super-Gaussian distribution; WP projected-back approximation with nuisance-exclusion contributed to networks reconstruction.Comparison with existing methodsSimulation 1 revealed WASICA successfully recovered super-Gaussian and some Gaussian-like sources. Simulation 2 and hybrid data experiments showed that WASICA with good temporal performance had stronger source recovery ability and signal detection sensitivity spatially than FastICA, Infomax and SACICA did; the higher intra-consistency in task-related experiments denoted WASICA occupied stronger spatial robustness against smooth kernels.ConclusionsWASICA was a promising brain signal separation model with charming spatial–temporal performance.
Journal: Journal of Neuroscience Methods - Volume 246, 15 May 2015, Pages 75–96