کد مقاله | کد نشریه | سال انتشار | مقاله انگلیسی | نسخه تمام متن |
---|---|---|---|---|
4375093 | 1303241 | 2012 | 11 صفحه PDF | دانلود رایگان |
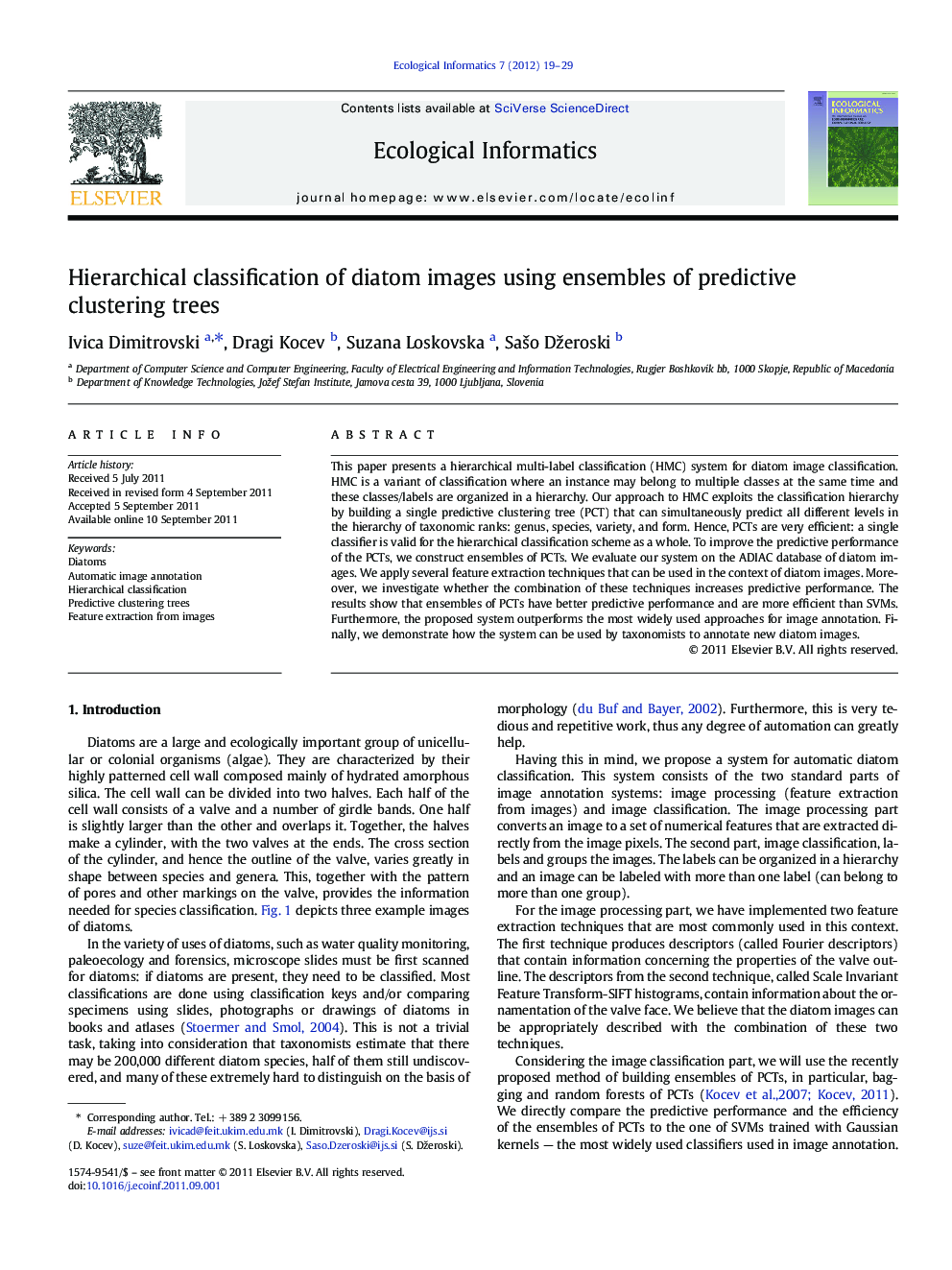
This paper presents a hierarchical multi-label classification (HMC) system for diatom image classification. HMC is a variant of classification where an instance may belong to multiple classes at the same time and these classes/labels are organized in a hierarchy. Our approach to HMC exploits the classification hierarchy by building a single predictive clustering tree (PCT) that can simultaneously predict all different levels in the hierarchy of taxonomic ranks: genus, species, variety, and form. Hence, PCTs are very efficient: a single classifier is valid for the hierarchical classification scheme as a whole. To improve the predictive performance of the PCTs, we construct ensembles of PCTs. We evaluate our system on the ADIAC database of diatom images. We apply several feature extraction techniques that can be used in the context of diatom images. Moreover, we investigate whether the combination of these techniques increases predictive performance. The results show that ensembles of PCTs have better predictive performance and are more efficient than SVMs. Furthermore, the proposed system outperforms the most widely used approaches for image annotation. Finally, we demonstrate how the system can be used by taxonomists to annotate new diatom images.
► Hierarchical multi-label classification system for diatom image classification.
► State-of-the-art classification methods coupled with Fourier and SIFT image features.
► Bagging and random forests that exploit the connections in the taxonomic rank.
► Easy applicable and efficient system with state-of-the-art performance.
Journal: Ecological Informatics - Volume 7, Issue 1, January 2012, Pages 19–29