کد مقاله | کد نشریه | سال انتشار | مقاله انگلیسی | نسخه تمام متن |
---|---|---|---|---|
443696 | 692752 | 2012 | 18 صفحه PDF | دانلود رایگان |
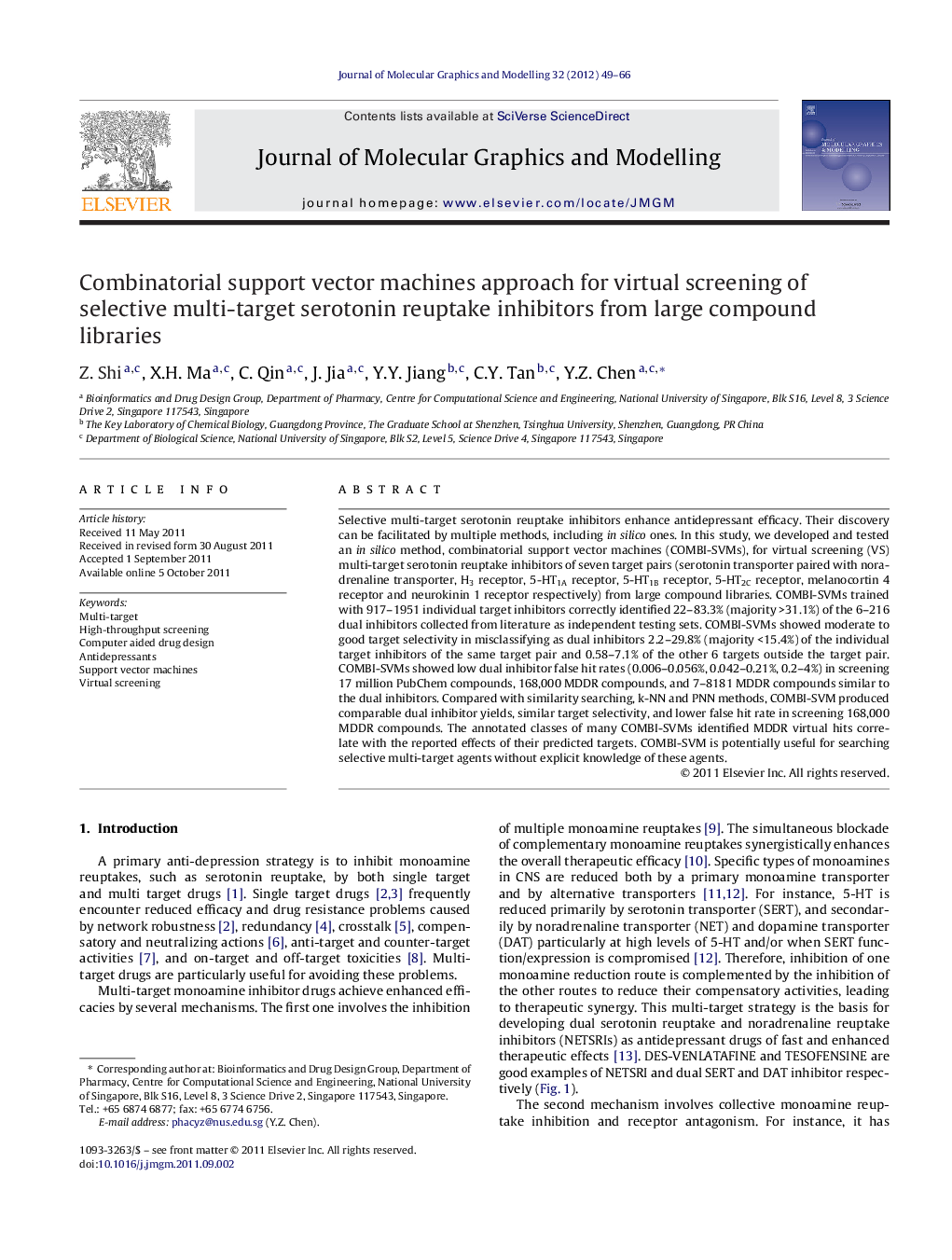
Selective multi-target serotonin reuptake inhibitors enhance antidepressant efficacy. Their discovery can be facilitated by multiple methods, including in silico ones. In this study, we developed and tested an in silico method, combinatorial support vector machines (COMBI-SVMs), for virtual screening (VS) multi-target serotonin reuptake inhibitors of seven target pairs (serotonin transporter paired with noradrenaline transporter, H3 receptor, 5-HT1A receptor, 5-HT1B receptor, 5-HT2C receptor, melanocortin 4 receptor and neurokinin 1 receptor respectively) from large compound libraries. COMBI-SVMs trained with 917–1951 individual target inhibitors correctly identified 22–83.3% (majority >31.1%) of the 6–216 dual inhibitors collected from literature as independent testing sets. COMBI-SVMs showed moderate to good target selectivity in misclassifying as dual inhibitors 2.2–29.8% (majority <15.4%) of the individual target inhibitors of the same target pair and 0.58–7.1% of the other 6 targets outside the target pair. COMBI-SVMs showed low dual inhibitor false hit rates (0.006–0.056%, 0.042–0.21%, 0.2–4%) in screening 17 million PubChem compounds, 168,000 MDDR compounds, and 7–8181 MDDR compounds similar to the dual inhibitors. Compared with similarity searching, k-NN and PNN methods, COMBI-SVM produced comparable dual inhibitor yields, similar target selectivity, and lower false hit rate in screening 168,000 MDDR compounds. The annotated classes of many COMBI-SVMs identified MDDR virtual hits correlate with the reported effects of their predicted targets. COMBI-SVM is potentially useful for searching selective multi-target agents without explicit knowledge of these agents.
The COMBI-SVMs diagram. Individual SVM models are built after 5-fold cross-validation where the training set is randomly divided into 5 sub sets and in turns 4 sets are used for training and 1 set for testing to choose the best parameters for model construction. Virtual hits simultaneously selected by all individual SVM models are considered as multi-target virtual hits. INBs = inhibitors; Non-INBs = non-inhibitors.Figure optionsDownload high-quality image (132 K)Download as PowerPoint slideHighlights
► Multi-target serotonin reuptake inhibitor virtual screening tools were developed.
► They were designed as tools for searching large compound libraries.
► They were found to achieve good yield, target selectivity, and low false-hit rate.
► They are based on combinatorial support vector machines approach.
► Their performance was compared with other virtual screening methods.
Journal: Journal of Molecular Graphics and Modelling - Volume 32, February 2012, Pages 49–66