کد مقاله | کد نشریه | سال انتشار | مقاله انگلیسی | نسخه تمام متن |
---|---|---|---|---|
443946 | 692824 | 2012 | 12 صفحه PDF | دانلود رایگان |
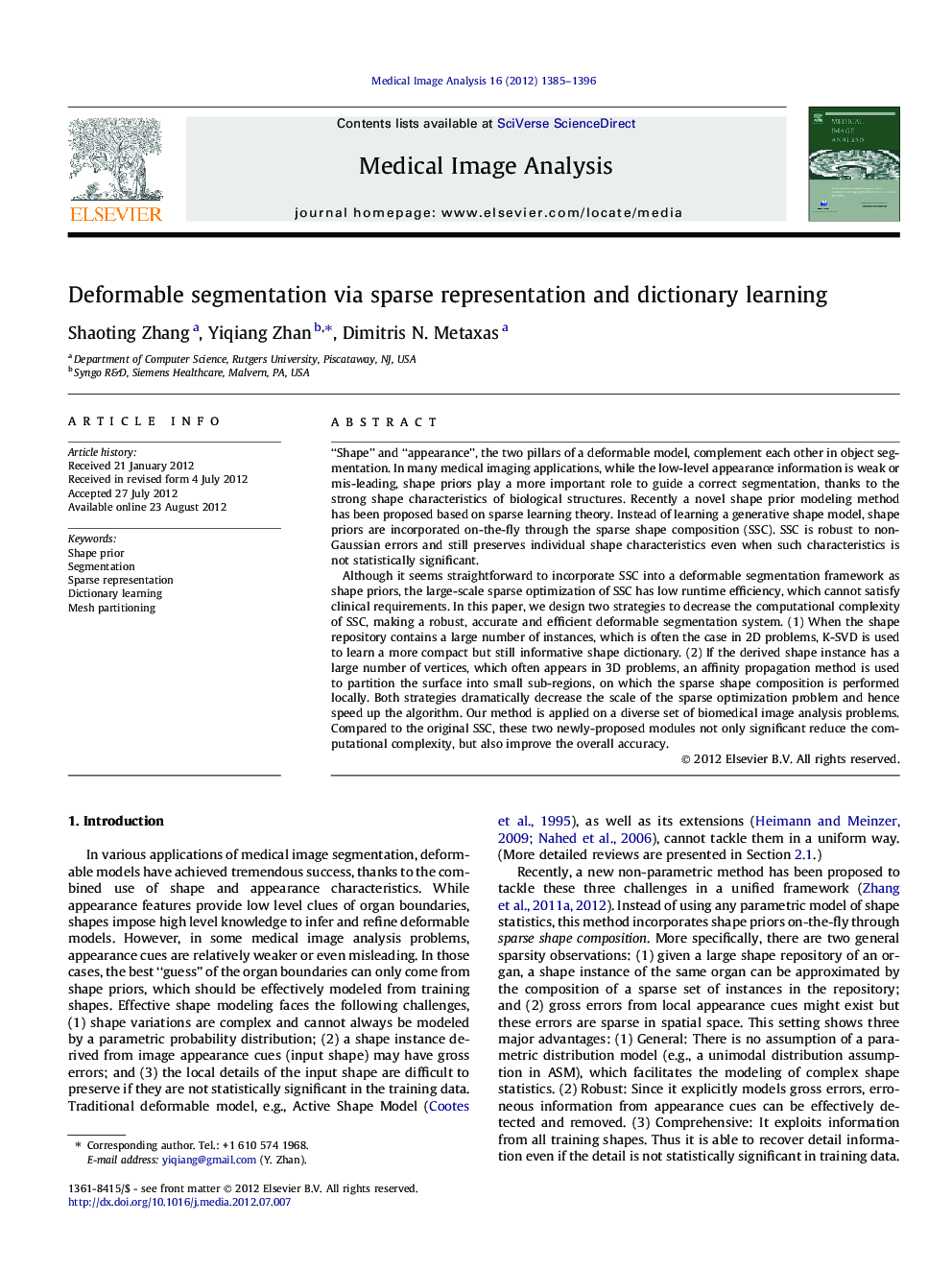
“Shape” and “appearance”, the two pillars of a deformable model, complement each other in object segmentation. In many medical imaging applications, while the low-level appearance information is weak or mis-leading, shape priors play a more important role to guide a correct segmentation, thanks to the strong shape characteristics of biological structures. Recently a novel shape prior modeling method has been proposed based on sparse learning theory. Instead of learning a generative shape model, shape priors are incorporated on-the-fly through the sparse shape composition (SSC). SSC is robust to non-Gaussian errors and still preserves individual shape characteristics even when such characteristics is not statistically significant.Although it seems straightforward to incorporate SSC into a deformable segmentation framework as shape priors, the large-scale sparse optimization of SSC has low runtime efficiency, which cannot satisfy clinical requirements. In this paper, we design two strategies to decrease the computational complexity of SSC, making a robust, accurate and efficient deformable segmentation system. (1) When the shape repository contains a large number of instances, which is often the case in 2D problems, K-SVD is used to learn a more compact but still informative shape dictionary. (2) If the derived shape instance has a large number of vertices, which often appears in 3D problems, an affinity propagation method is used to partition the surface into small sub-regions, on which the sparse shape composition is performed locally. Both strategies dramatically decrease the scale of the sparse optimization problem and hence speed up the algorithm. Our method is applied on a diverse set of biomedical image analysis problems. Compared to the original SSC, these two newly-proposed modules not only significant reduce the computational complexity, but also improve the overall accuracy.
Figure optionsDownload high-quality image (184 K)Download as PowerPoint slideHighlights
► Sparse shape composition is embedded into the deformable segmentation framework.
► K-SVD based dictionary learning is employed to handle large-scale training data.
► Affinity propagation is used to partition and model local priors of complex shapes.
► Our approach is evaluated in three very diverse medical applications.
Journal: Medical Image Analysis - Volume 16, Issue 7, October 2012, Pages 1385–1396