کد مقاله | کد نشریه | سال انتشار | مقاله انگلیسی | نسخه تمام متن |
---|---|---|---|---|
443996 | 692841 | 2016 | 12 صفحه PDF | دانلود رایگان |
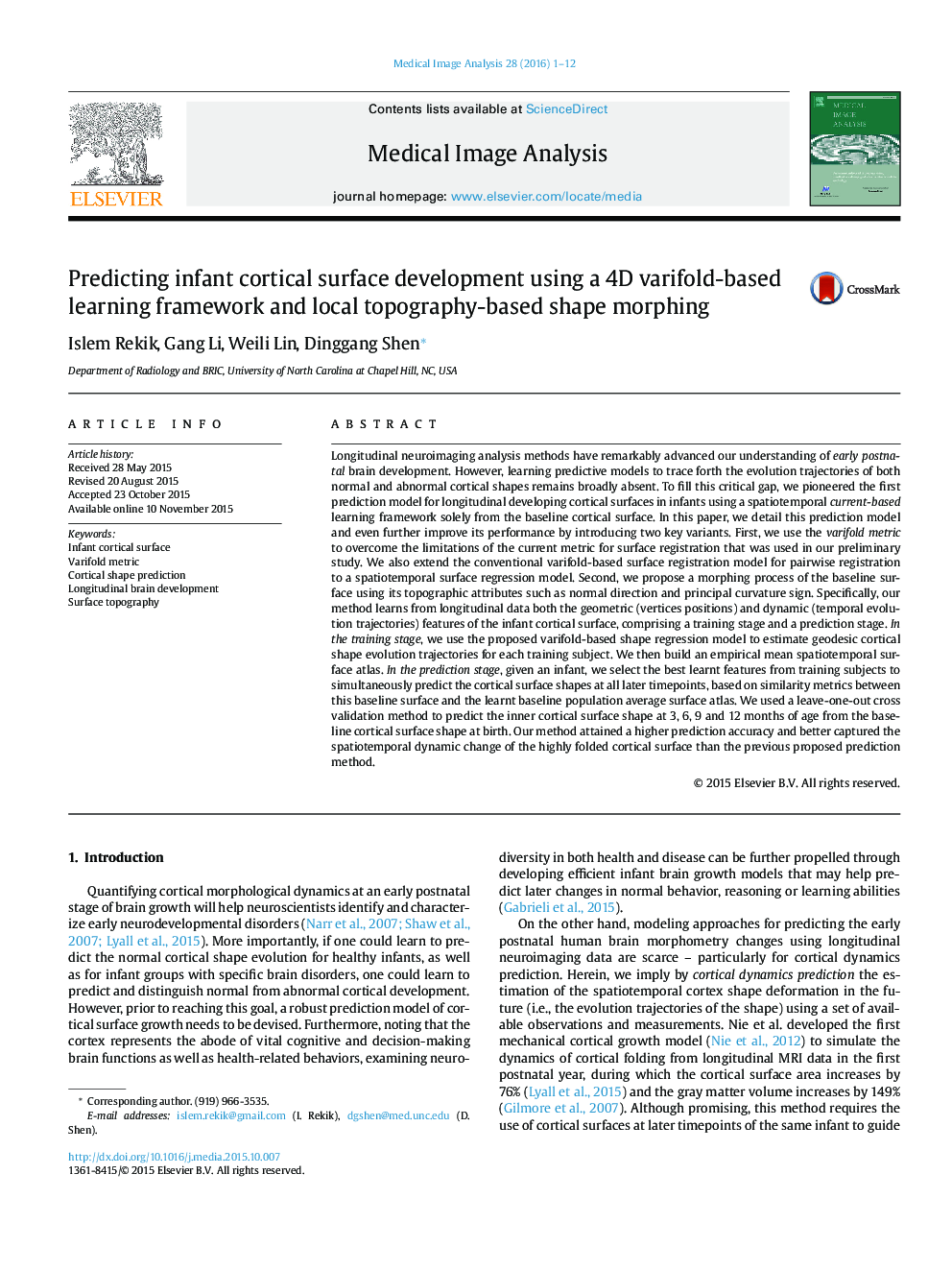
• Topography-based cortical shape evolution prediction model.
• Cortical growth prediction in the first year of life solely from baseline shape.
• 4D varifold-based shape evolution learning framework.
Longitudinal neuroimaging analysis methods have remarkably advanced our understanding of early postnatal brain development. However, learning predictive models to trace forth the evolution trajectories of both normal and abnormal cortical shapes remains broadly absent. To fill this critical gap, we pioneered the first prediction model for longitudinal developing cortical surfaces in infants using a spatiotemporal current-based learning framework solely from the baseline cortical surface. In this paper, we detail this prediction model and even further improve its performance by introducing two key variants. First, we use the varifold metric to overcome the limitations of the current metric for surface registration that was used in our preliminary study. We also extend the conventional varifold-based surface registration model for pairwise registration to a spatiotemporal surface regression model. Second, we propose a morphing process of the baseline surface using its topographic attributes such as normal direction and principal curvature sign. Specifically, our method learns from longitudinal data both the geometric (vertices positions) and dynamic (temporal evolution trajectories) features of the infant cortical surface, comprising a training stage and a prediction stage. In the training stage, we use the proposed varifold-based shape regression model to estimate geodesic cortical shape evolution trajectories for each training subject. We then build an empirical mean spatiotemporal surface atlas. In the prediction stage, given an infant, we select the best learnt features from training subjects to simultaneously predict the cortical surface shapes at all later timepoints, based on similarity metrics between this baseline surface and the learnt baseline population average surface atlas. We used a leave-one-out cross validation method to predict the inner cortical surface shape at 3, 6, 9 and 12 months of age from the baseline cortical surface shape at birth. Our method attained a higher prediction accuracy and better captured the spatiotemporal dynamic change of the highly folded cortical surface than the previous proposed prediction method.
Figure optionsDownload high-quality image (239 K)Download as PowerPoint slide
Journal: Medical Image Analysis - Volume 28, February 2016, Pages 1–12