کد مقاله | کد نشریه | سال انتشار | مقاله انگلیسی | نسخه تمام متن |
---|---|---|---|---|
444078 | 692879 | 2012 | 11 صفحه PDF | دانلود رایگان |
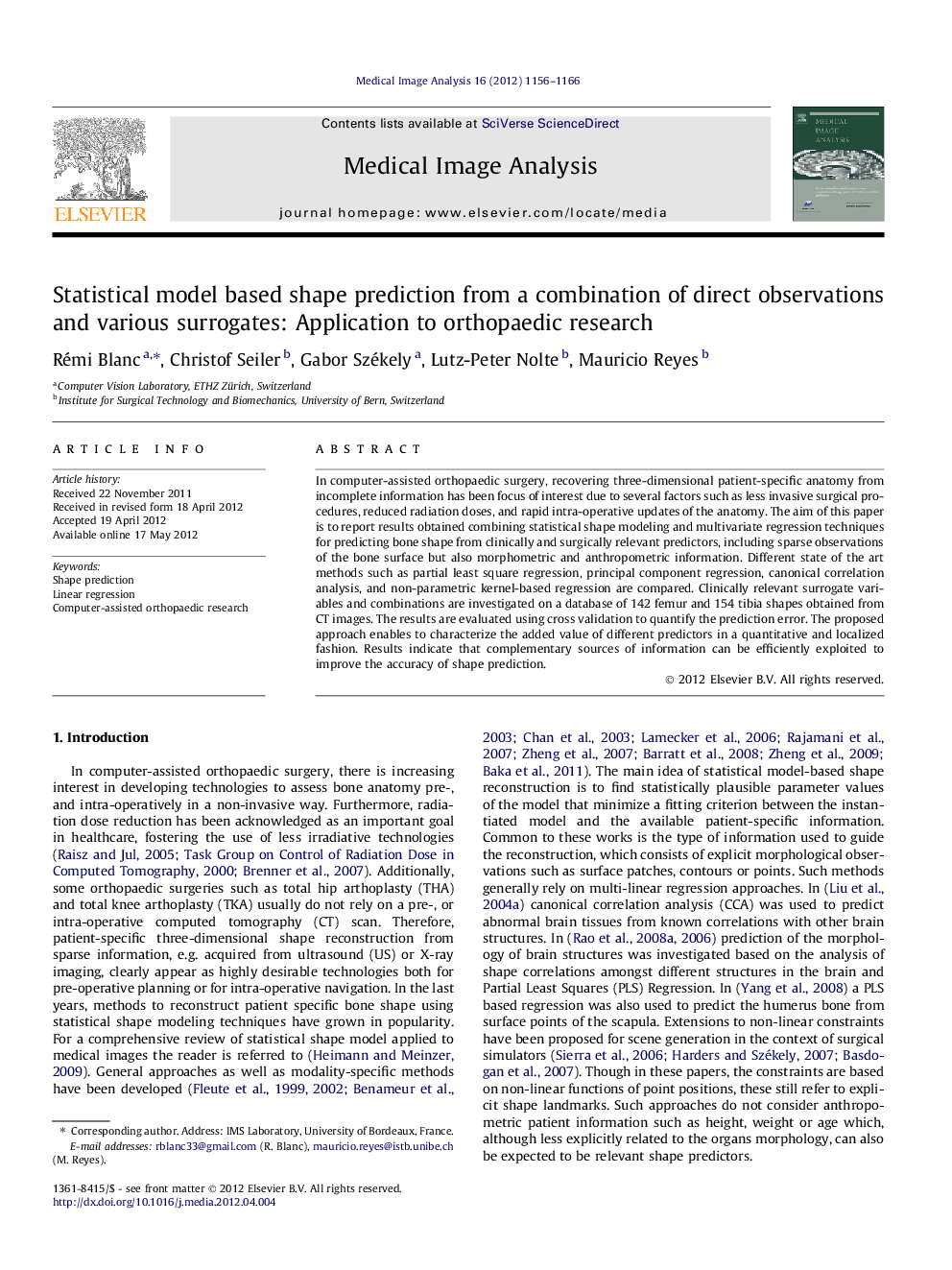
In computer-assisted orthopaedic surgery, recovering three-dimensional patient-specific anatomy from incomplete information has been focus of interest due to several factors such as less invasive surgical procedures, reduced radiation doses, and rapid intra-operative updates of the anatomy. The aim of this paper is to report results obtained combining statistical shape modeling and multivariate regression techniques for predicting bone shape from clinically and surgically relevant predictors, including sparse observations of the bone surface but also morphometric and anthropometric information. Different state of the art methods such as partial least square regression, principal component regression, canonical correlation analysis, and non-parametric kernel-based regression are compared. Clinically relevant surrogate variables and combinations are investigated on a database of 142 femur and 154 tibia shapes obtained from CT images. The results are evaluated using cross validation to quantify the prediction error. The proposed approach enables to characterize the added value of different predictors in a quantitative and localized fashion. Results indicate that complementary sources of information can be efficiently exploited to improve the accuracy of shape prediction.
Figure optionsDownload high-quality image (90 K)Download as PowerPoint slideHighlights
► In this study, we investigate shape prediction from heterogeneous predictors.
► We consider surface-based, but also anthropometric and morphometric predictors.
► The locality of the information provided by various predictors is assessed.
► Complementary information is shown to improve shape prediction accuracy.
Journal: Medical Image Analysis - Volume 16, Issue 6, August 2012, Pages 1156–1166