کد مقاله | کد نشریه | سال انتشار | مقاله انگلیسی | نسخه تمام متن |
---|---|---|---|---|
444862 | 693060 | 2006 | 13 صفحه PDF | دانلود رایگان |
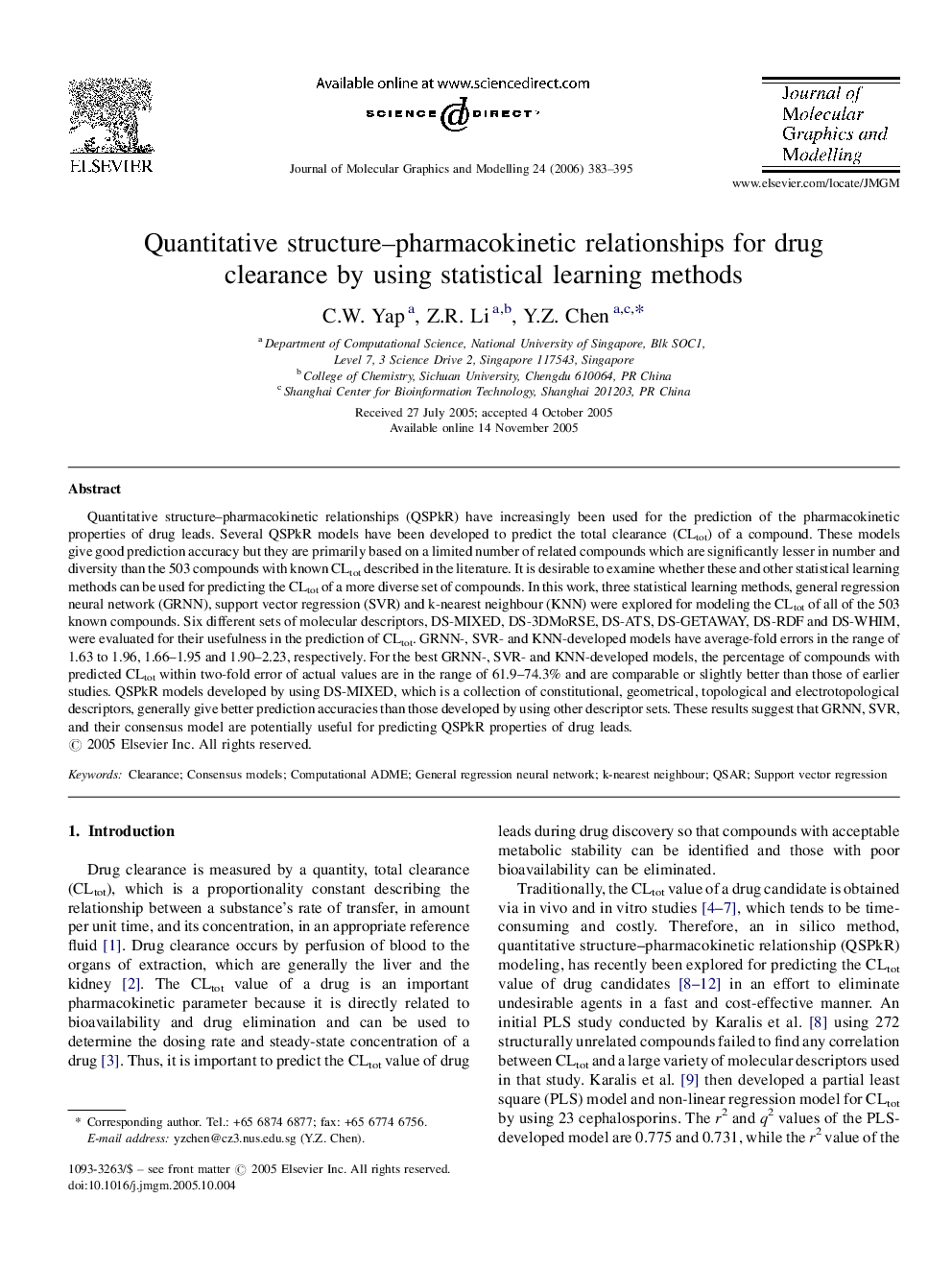
Quantitative structure–pharmacokinetic relationships (QSPkR) have increasingly been used for the prediction of the pharmacokinetic properties of drug leads. Several QSPkR models have been developed to predict the total clearance (CLtot) of a compound. These models give good prediction accuracy but they are primarily based on a limited number of related compounds which are significantly lesser in number and diversity than the 503 compounds with known CLtot described in the literature. It is desirable to examine whether these and other statistical learning methods can be used for predicting the CLtot of a more diverse set of compounds. In this work, three statistical learning methods, general regression neural network (GRNN), support vector regression (SVR) and k-nearest neighbour (KNN) were explored for modeling the CLtot of all of the 503 known compounds. Six different sets of molecular descriptors, DS-MIXED, DS-3DMoRSE, DS-ATS, DS-GETAWAY, DS-RDF and DS-WHIM, were evaluated for their usefulness in the prediction of CLtot. GRNN-, SVR- and KNN-developed models have average-fold errors in the range of 1.63 to 1.96, 1.66–1.95 and 1.90–2.23, respectively. For the best GRNN-, SVR- and KNN-developed models, the percentage of compounds with predicted CLtot within two-fold error of actual values are in the range of 61.9–74.3% and are comparable or slightly better than those of earlier studies. QSPkR models developed by using DS-MIXED, which is a collection of constitutional, geometrical, topological and electrotopological descriptors, generally give better prediction accuracies than those developed by using other descriptor sets. These results suggest that GRNN, SVR, and their consensus model are potentially useful for predicting QSPkR properties of drug leads.
Journal: Journal of Molecular Graphics and Modelling - Volume 24, Issue 5, March 2006, Pages 383–395