کد مقاله | کد نشریه | سال انتشار | مقاله انگلیسی | نسخه تمام متن |
---|---|---|---|---|
445196 | 693154 | 2011 | 12 صفحه PDF | دانلود رایگان |
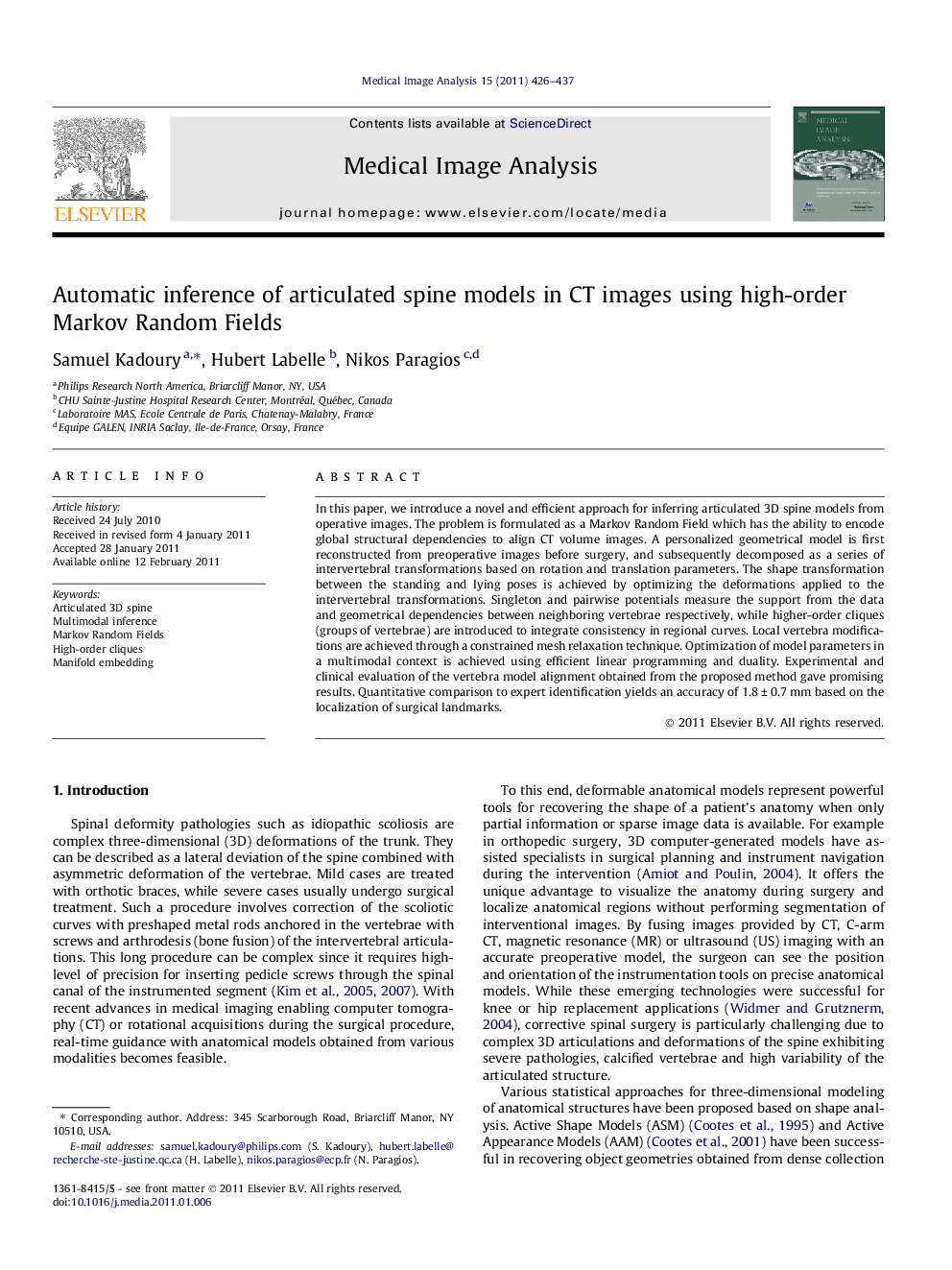
In this paper, we introduce a novel and efficient approach for inferring articulated 3D spine models from operative images. The problem is formulated as a Markov Random Field which has the ability to encode global structural dependencies to align CT volume images. A personalized geometrical model is first reconstructed from preoperative images before surgery, and subsequently decomposed as a series of intervertebral transformations based on rotation and translation parameters. The shape transformation between the standing and lying poses is achieved by optimizing the deformations applied to the intervertebral transformations. Singleton and pairwise potentials measure the support from the data and geometrical dependencies between neighboring vertebrae respectively, while higher-order cliques (groups of vertebrae) are introduced to integrate consistency in regional curves. Local vertebra modifications are achieved through a constrained mesh relaxation technique. Optimization of model parameters in a multimodal context is achieved using efficient linear programming and duality. Experimental and clinical evaluation of the vertebra model alignment obtained from the proposed method gave promising results. Quantitative comparison to expert identification yields an accuracy of 1.8 ± 0.7 mm based on the localization of surgical landmarks.
A personalized geometrical model is reconstructed from preoperative X-ray images and represented as an articulated shape based on a series of local rotations and translations. The shape transformation between the standing and lying poses is achieved by optimizing the intervertebral articulations based on image similarity with the interventional CT scan. Higher-order cliques anchored on low-dimensional manifold statistics are introduced to integrate consistency in anatomical curves. Optimization of global model parameters in a multimodal context is achieved using efficient linear programming and duality, followed by local triangular mesh relaxation of vertebra models. This generates a fused spine model with CT images, aligning surgical landmarks to intraoperative image data.Figure optionsDownload high-quality image (94 K)Download as PowerPoint slideResearch highlights
► Articulated preoperative spine model can be used to infer shape from surgical images.
► High-order Markov Random Field optimization achieves fast and accurate results for multimodal registration.
► Prior knowledge constrains discrete minimization methods with anatomically coherent shape transformations.
► Complex deformations from standing to lying poses can be reliably determined via manifold learning schemes underlying natural variations.
► Accurate landmark localization using fused preoperative model is feasible in spine surgery.
Journal: Medical Image Analysis - Volume 15, Issue 4, August 2011, Pages 426–437