کد مقاله | کد نشریه | سال انتشار | مقاله انگلیسی | نسخه تمام متن |
---|---|---|---|---|
4493811 | 1318672 | 2009 | 11 صفحه PDF | دانلود رایگان |
عنوان انگلیسی مقاله ISI
Efficient data assimilation method based on chaos theory and Kalman filter with an application in Singapore Regional Model
دانلود مقاله + سفارش ترجمه
دانلود مقاله ISI انگلیسی
رایگان برای ایرانیان
کلمات کلیدی
موضوعات مرتبط
علوم زیستی و بیوفناوری
علوم کشاورزی و بیولوژیک
علوم کشاورزی و بیولوژیک (عمومی)
پیش نمایش صفحه اول مقاله
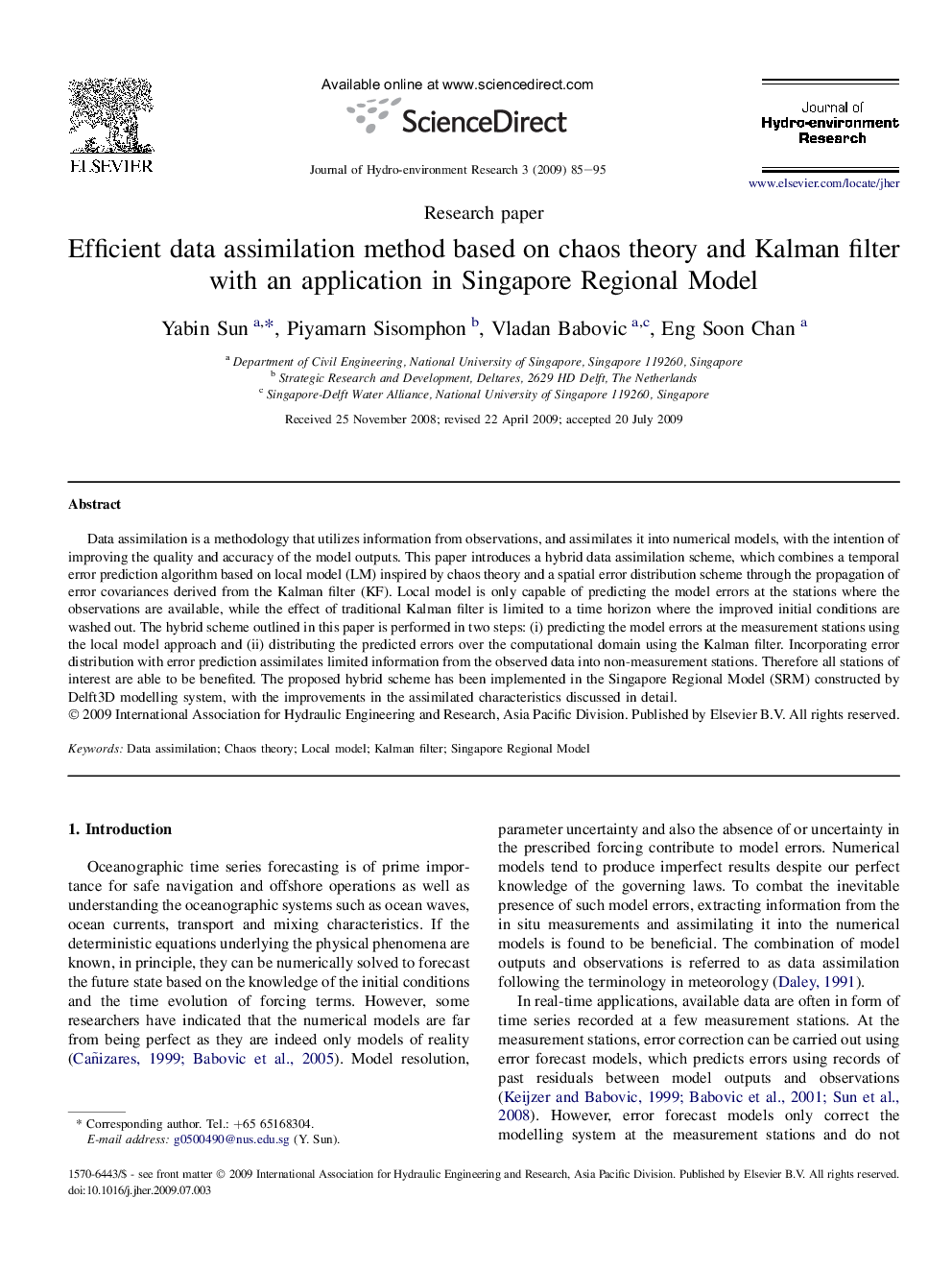
چکیده انگلیسی
Data assimilation is a methodology that utilizes information from observations, and assimilates it into numerical models, with the intention of improving the quality and accuracy of the model outputs. This paper introduces a hybrid data assimilation scheme, which combines a temporal error prediction algorithm based on local model (LM) inspired by chaos theory and a spatial error distribution scheme through the propagation of error covariances derived from the Kalman filter (KF). Local model is only capable of predicting the model errors at the stations where the observations are available, while the effect of traditional Kalman filter is limited to a time horizon where the improved initial conditions are washed out. The hybrid scheme outlined in this paper is performed in two steps: (i) predicting the model errors at the measurement stations using the local model approach and (ii) distributing the predicted errors over the computational domain using the Kalman filter. Incorporating error distribution with error prediction assimilates limited information from the observed data into non-measurement stations. Therefore all stations of interest are able to be benefited. The proposed hybrid scheme has been implemented in the Singapore Regional Model (SRM) constructed by Delft3D modelling system, with the improvements in the assimilated characteristics discussed in detail.
ناشر
Database: Elsevier - ScienceDirect (ساینس دایرکت)
Journal: Journal of Hydro-environment Research - Volume 3, Issue 2, October 2009, Pages 85-95
Journal: Journal of Hydro-environment Research - Volume 3, Issue 2, October 2009, Pages 85-95
نویسندگان
Yabin Sun, Piyamarn Sisomphon, Vladan Babovic, Eng Soon Chan,