کد مقاله | کد نشریه | سال انتشار | مقاله انگلیسی | نسخه تمام متن |
---|---|---|---|---|
4517852 | 1624983 | 2016 | 10 صفحه PDF | دانلود رایگان |
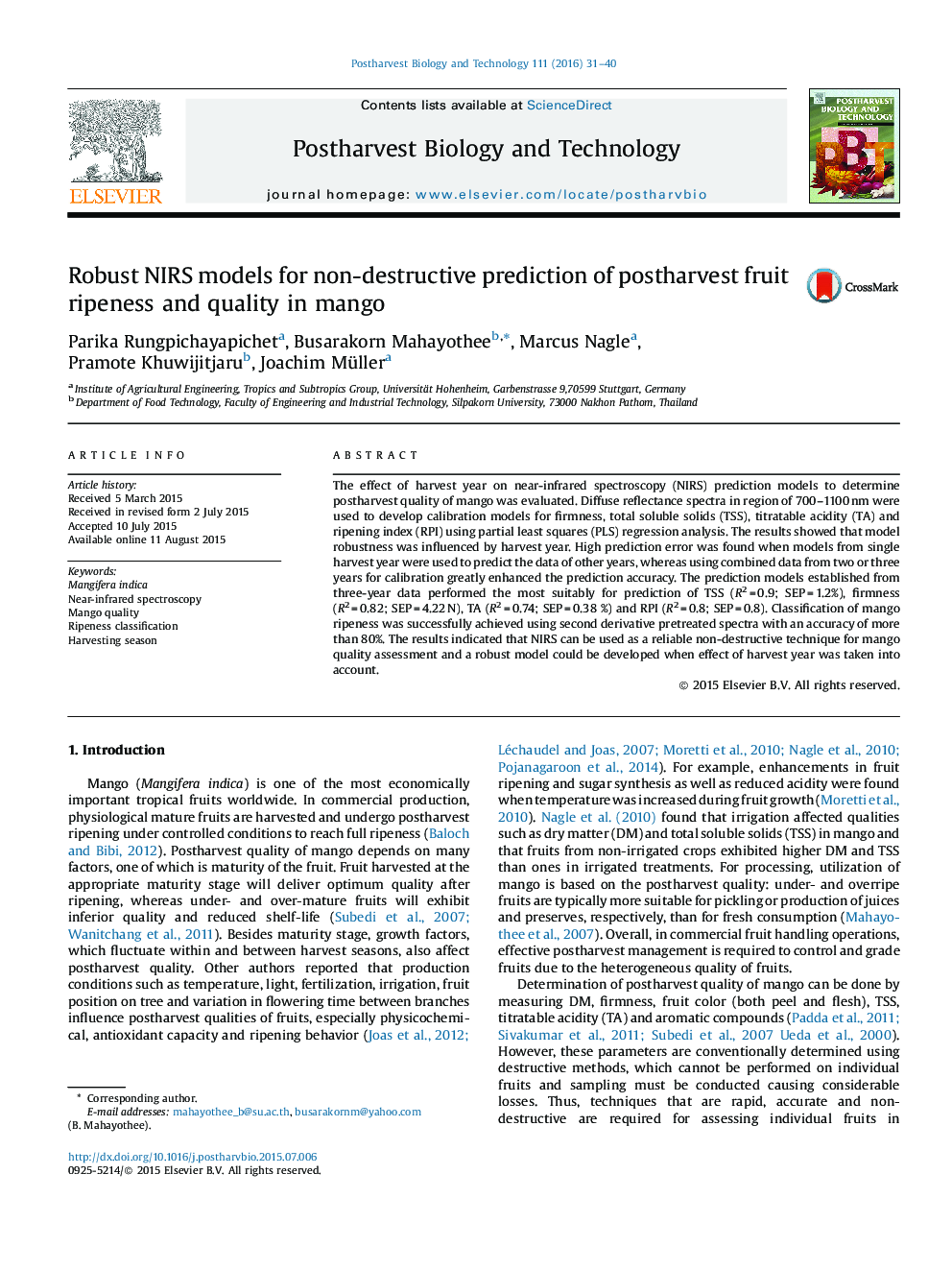
• Internal quality attributes of mango were accurately predicted by short-wave NIRS.
• Using data from multiple seasons for calibration provided robust prediction models.
• NIRS was able to directly predict a multifactorial ripening index (RPI).
• Fruit ripeness classification by NIRS calibrations showed accuracies between 59 and 88%.
The effect of harvest year on near-infrared spectroscopy (NIRS) prediction models to determine postharvest quality of mango was evaluated. Diffuse reflectance spectra in region of 700–1100 nm were used to develop calibration models for firmness, total soluble solids (TSS), titratable acidity (TA) and ripening index (RPI) using partial least squares (PLS) regression analysis. The results showed that model robustness was influenced by harvest year. High prediction error was found when models from single harvest year were used to predict the data of other years, whereas using combined data from two or three years for calibration greatly enhanced the prediction accuracy. The prediction models established from three-year data performed the most suitably for prediction of TSS (R2 = 0.9; SEP = 1.2%), firmness (R2 = 0.82; SEP = 4.22 N), TA (R2 = 0.74; SEP = 0.38 %) and RPI (R2 = 0.8; SEP = 0.8). Classification of mango ripeness was successfully achieved using second derivative pretreated spectra with an accuracy of more than 80%. The results indicated that NIRS can be used as a reliable non-destructive technique for mango quality assessment and a robust model could be developed when effect of harvest year was taken into account.
Journal: Postharvest Biology and Technology - Volume 111, January 2016, Pages 31–40