کد مقاله | کد نشریه | سال انتشار | مقاله انگلیسی | نسخه تمام متن |
---|---|---|---|---|
476786 | 1446056 | 2013 | 14 صفحه PDF | دانلود رایگان |
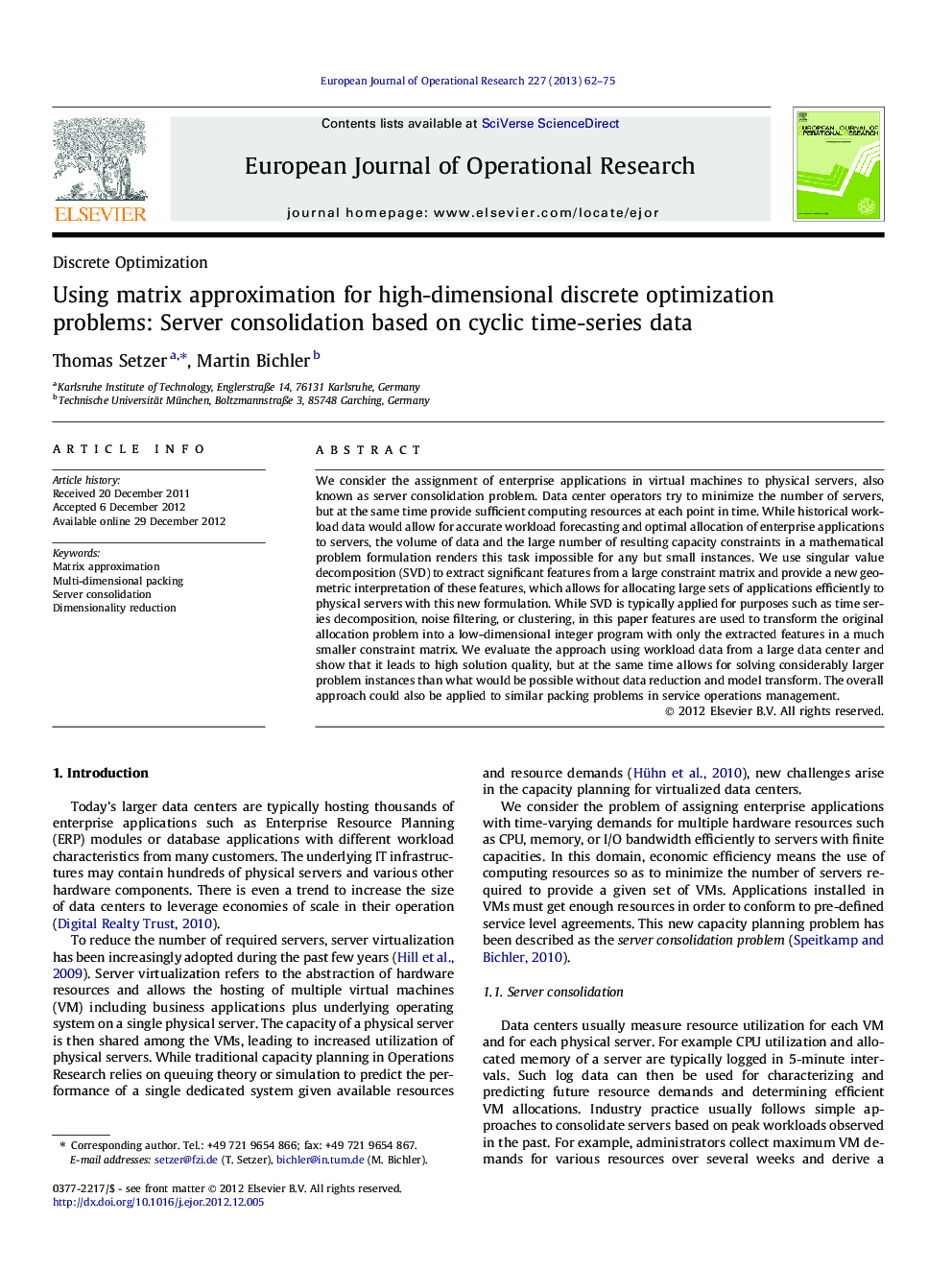
We consider the assignment of enterprise applications in virtual machines to physical servers, also known as server consolidation problem. Data center operators try to minimize the number of servers, but at the same time provide sufficient computing resources at each point in time. While historical workload data would allow for accurate workload forecasting and optimal allocation of enterprise applications to servers, the volume of data and the large number of resulting capacity constraints in a mathematical problem formulation renders this task impossible for any but small instances. We use singular value decomposition (SVD) to extract significant features from a large constraint matrix and provide a new geometric interpretation of these features, which allows for allocating large sets of applications efficiently to physical servers with this new formulation. While SVD is typically applied for purposes such as time series decomposition, noise filtering, or clustering, in this paper features are used to transform the original allocation problem into a low-dimensional integer program with only the extracted features in a much smaller constraint matrix. We evaluate the approach using workload data from a large data center and show that it leads to high solution quality, but at the same time allows for solving considerably larger problem instances than what would be possible without data reduction and model transform. The overall approach could also be applied to similar packing problems in service operations management.
► Interpretation of singular vectors derived from constraint matrices.
► Packing problem transformation into a low-dimensional feature space.
► High scalability and near-optimal solutions in server consolidation experiments.
Journal: European Journal of Operational Research - Volume 227, Issue 1, 16 May 2013, Pages 62–75