کد مقاله | کد نشریه | سال انتشار | مقاله انگلیسی | نسخه تمام متن |
---|---|---|---|---|
479963 | 1446057 | 2013 | 10 صفحه PDF | دانلود رایگان |
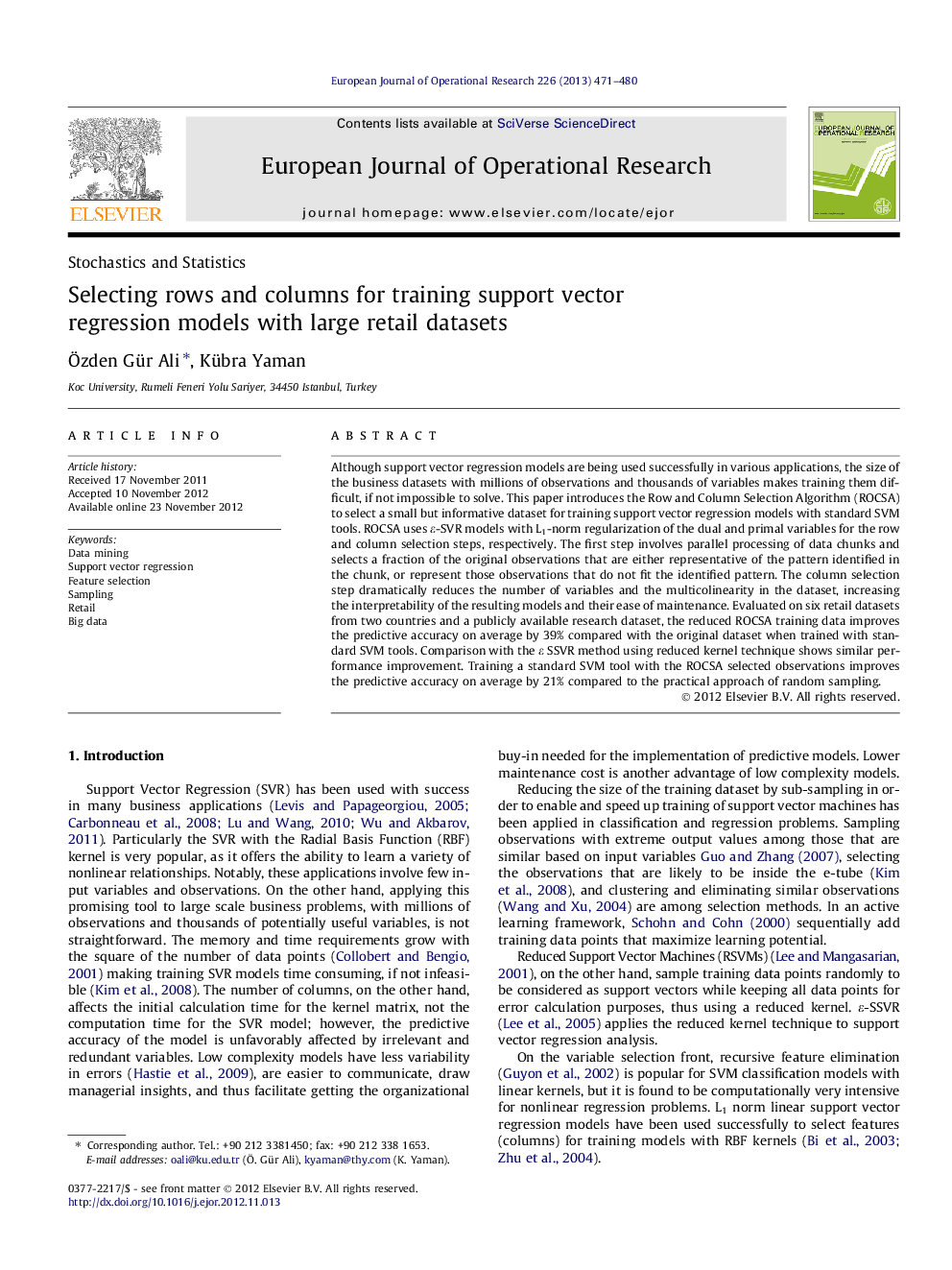
Although support vector regression models are being used successfully in various applications, the size of the business datasets with millions of observations and thousands of variables makes training them difficult, if not impossible to solve. This paper introduces the Row and Column Selection Algorithm (ROCSA) to select a small but informative dataset for training support vector regression models with standard SVM tools. ROCSA uses ε-SVR models with L1-norm regularization of the dual and primal variables for the row and column selection steps, respectively. The first step involves parallel processing of data chunks and selects a fraction of the original observations that are either representative of the pattern identified in the chunk, or represent those observations that do not fit the identified pattern. The column selection step dramatically reduces the number of variables and the multicolinearity in the dataset, increasing the interpretability of the resulting models and their ease of maintenance. Evaluated on six retail datasets from two countries and a publicly available research dataset, the reduced ROCSA training data improves the predictive accuracy on average by 39% compared with the original dataset when trained with standard SVM tools. Comparison with the ε SSVR method using reduced kernel technique shows similar performance improvement. Training a standard SVM tool with the ROCSA selected observations improves the predictive accuracy on average by 21% compared to the practical approach of random sampling.
► Method for reducing large business datasets to train nonlinear SVR models with standard SVM tools.
► ROCSA first selects rows in parallel chunks then selects columns with L1 regularized ε-SVR models.
► Accuracy evaluated on six large retail datasets from two countries and a public research dataset.
► 39% Better predictive accuracy vs. the full dataset trained with standard SVM tools and ε SSVR.
► Reduces model complexity, provides consistent variable selection in similar retail categories.
Journal: European Journal of Operational Research - Volume 226, Issue 3, 1 May 2013, Pages 471–480