کد مقاله | کد نشریه | سال انتشار | مقاله انگلیسی | نسخه تمام متن |
---|---|---|---|---|
4924347 | 1430836 | 2017 | 19 صفحه PDF | دانلود رایگان |
عنوان انگلیسی مقاله ISI
An offline approach for output-only Bayesian identification of stochastic nonlinear systems using unscented Kalman filtering
دانلود مقاله + سفارش ترجمه
دانلود مقاله ISI انگلیسی
رایگان برای ایرانیان
کلمات کلیدی
موضوعات مرتبط
مهندسی و علوم پایه
سایر رشته های مهندسی
مهندسی عمران و سازه
پیش نمایش صفحه اول مقاله
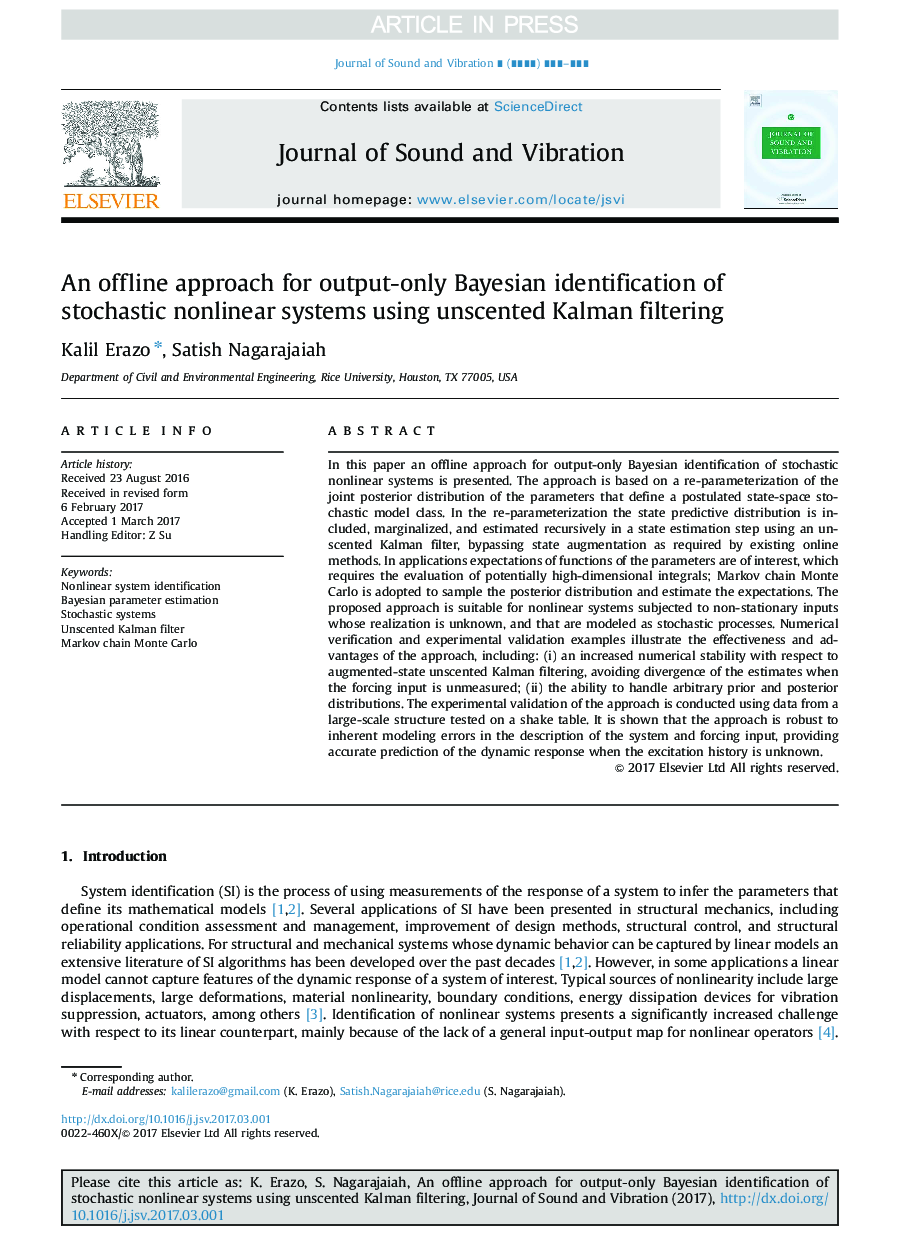
چکیده انگلیسی
In this paper an offline approach for output-only Bayesian identification of stochastic nonlinear systems is presented. The approach is based on a re-parameterization of the joint posterior distribution of the parameters that define a postulated state-space stochastic model class. In the re-parameterization the state predictive distribution is included, marginalized, and estimated recursively in a state estimation step using an unscented Kalman filter, bypassing state augmentation as required by existing online methods. In applications expectations of functions of the parameters are of interest, which requires the evaluation of potentially high-dimensional integrals; Markov chain Monte Carlo is adopted to sample the posterior distribution and estimate the expectations. The proposed approach is suitable for nonlinear systems subjected to non-stationary inputs whose realization is unknown, and that are modeled as stochastic processes. Numerical verification and experimental validation examples illustrate the effectiveness and advantages of the approach, including: (i) an increased numerical stability with respect to augmented-state unscented Kalman filtering, avoiding divergence of the estimates when the forcing input is unmeasured; (ii) the ability to handle arbitrary prior and posterior distributions. The experimental validation of the approach is conducted using data from a large-scale structure tested on a shake table. It is shown that the approach is robust to inherent modeling errors in the description of the system and forcing input, providing accurate prediction of the dynamic response when the excitation history is unknown.
ناشر
Database: Elsevier - ScienceDirect (ساینس دایرکت)
Journal: Journal of Sound and Vibration - Volume 397, 9 June 2017, Pages 222-240
Journal: Journal of Sound and Vibration - Volume 397, 9 June 2017, Pages 222-240
نویسندگان
Kalil Erazo, Satish Nagarajaiah,