کد مقاله | کد نشریه | سال انتشار | مقاله انگلیسی | نسخه تمام متن |
---|---|---|---|---|
4946093 | 1439270 | 2017 | 13 صفحه PDF | دانلود رایگان |
عنوان انگلیسی مقاله ISI
Synergy effects between grafting and subdivision in Re-RX with J48graft for the diagnosis of thyroid disease
دانلود مقاله + سفارش ترجمه
دانلود مقاله ISI انگلیسی
رایگان برای ایرانیان
کلمات کلیدی
موضوعات مرتبط
مهندسی و علوم پایه
مهندسی کامپیوتر
هوش مصنوعی
پیش نمایش صفحه اول مقاله
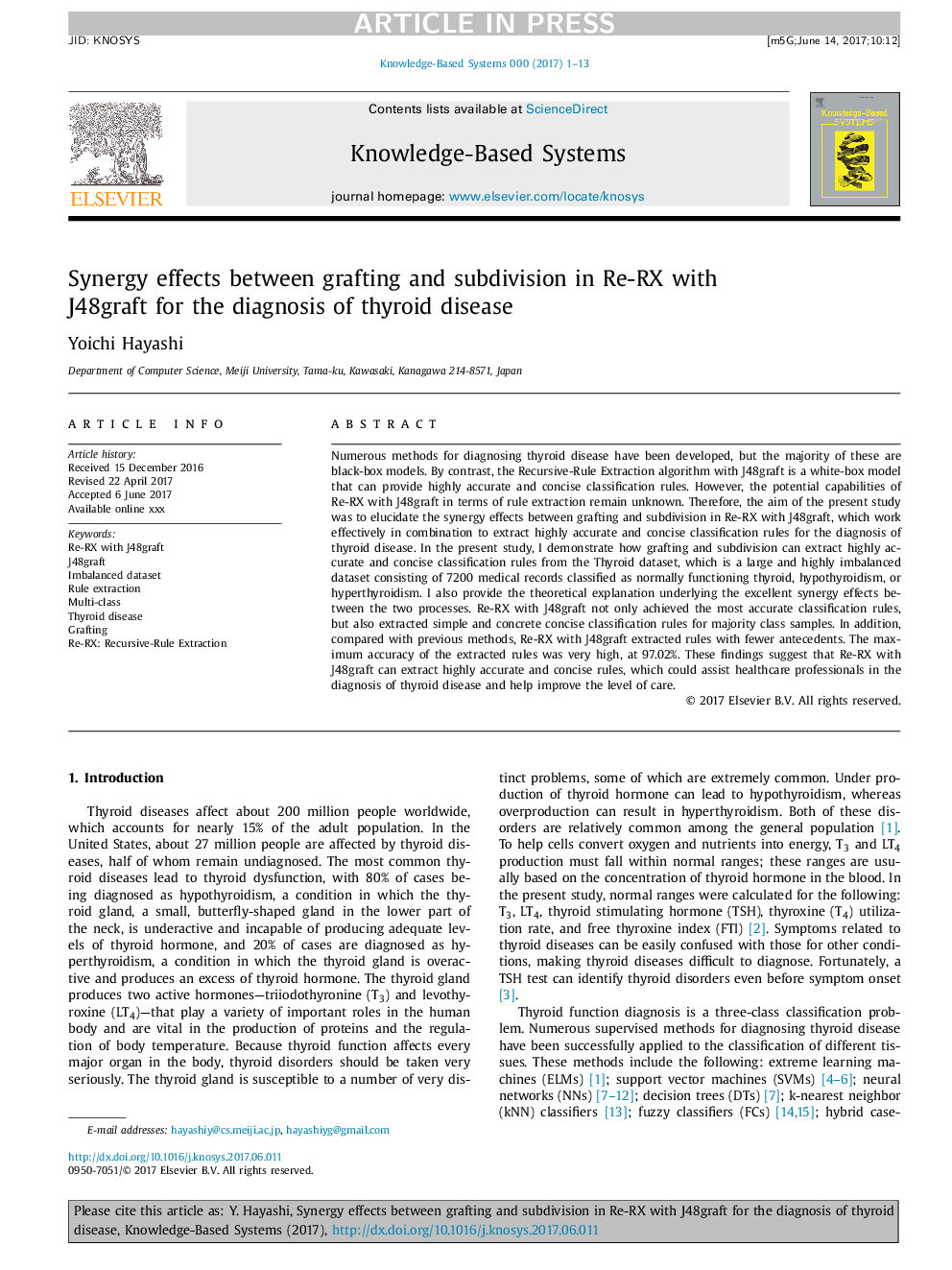
چکیده انگلیسی
Numerous methods for diagnosing thyroid disease have been developed, but the majority of these are black-box models. By contrast, the Recursive-Rule Extraction algorithm with J48graft is a white-box model that can provide highly accurate and concise classification rules. However, the potential capabilities of Re-RX with J48graft in terms of rule extraction remain unknown. Therefore, the aim of the present study was to elucidate the synergy effects between grafting and subdivision in Re-RX with J48graft, which work effectively in combination to extract highly accurate and concise classification rules for the diagnosis of thyroid disease. In the present study, I demonstrate how grafting and subdivision can extract highly accurate and concise classification rules from the Thyroid dataset, which is a large and highly imbalanced dataset consisting of 7200 medical records classified as normally functioning thyroid, hypothyroidism, or hyperthyroidism. I also provide the theoretical explanation underlying the excellent synergy effects between the two processes. Re-RX with J48graft not only achieved the most accurate classification rules, but also extracted simple and concrete concise classification rules for majority class samples. In addition, compared with previous methods, Re-RX with J48graft extracted rules with fewer antecedents. The maximum accuracy of the extracted rules was very high, at 97.02%. These findings suggest that Re-RX with J48graft can extract highly accurate and concise rules, which could assist healthcare professionals in the diagnosis of thyroid disease and help improve the level of care.
ناشر
Database: Elsevier - ScienceDirect (ساینس دایرکت)
Journal: Knowledge-Based Systems - Volume 131, 1 September 2017, Pages 170-182
Journal: Knowledge-Based Systems - Volume 131, 1 September 2017, Pages 170-182
نویسندگان
Yoichi Hayashi,