کد مقاله | کد نشریه | سال انتشار | مقاله انگلیسی | نسخه تمام متن |
---|---|---|---|---|
494663 | 862802 | 2016 | 17 صفحه PDF | دانلود رایگان |
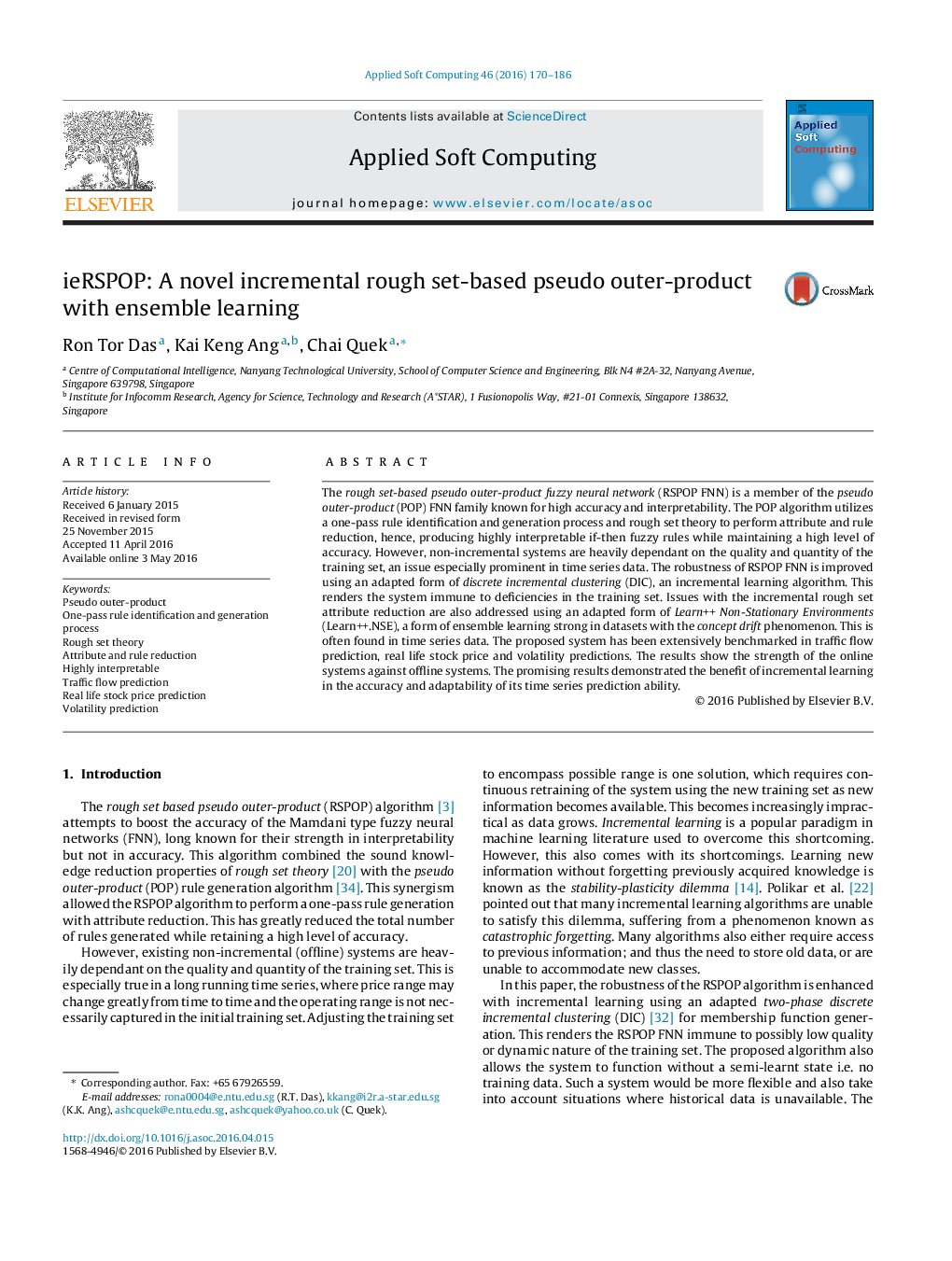
• The POP algorithm utilizes a one-pass rule identification and generation process and rough set theory to perform attribute and rule reduction.
• It produces highly interpretable if-then fuzzy rules while maintaining a high level of accuracy.
• The incremental MF generation algorithm proposed in this paper works well for time series, due to the two-phase adapted DIC.
• The robustness of RSPOP Fuzzy neural network is improved using a modified discrete incremental clustering clustering technique.
• Learn++ Non-Stationary Environments (Learn++.NSE), a form of ensemble learning good for handling datasets with the concept drift phenomenon is used.
• The proposed system has been extensively benchmarked in traffic flow prediction, real life stock price and volatility predictions.
The rough set-based pseudo outer-product fuzzy neural network (RSPOP FNN) is a member of the pseudo outer-product (POP) FNN family known for high accuracy and interpretability. The POP algorithm utilizes a one-pass rule identification and generation process and rough set theory to perform attribute and rule reduction, hence, producing highly interpretable if-then fuzzy rules while maintaining a high level of accuracy. However, non-incremental systems are heavily dependant on the quality and quantity of the training set, an issue especially prominent in time series data. The robustness of RSPOP FNN is improved using an adapted form of discrete incremental clustering (DIC), an incremental learning algorithm. This renders the system immune to deficiencies in the training set. Issues with the incremental rough set attribute reduction are also addressed using an adapted form of Learn++ Non-Stationary Environments (Learn++.NSE), a form of ensemble learning strong in datasets with the concept drift phenomenon. This is often found in time series data. The proposed system has been extensively benchmarked in traffic flow prediction, real life stock price and volatility predictions. The results show the strength of the online systems against offline systems. The promising results demonstrated the benefit of incremental learning in the accuracy and adaptability of its time series prediction ability.
Figure optionsDownload as PowerPoint slide
Journal: Applied Soft Computing - Volume 46, September 2016, Pages 170–186