کد مقاله | کد نشریه | سال انتشار | مقاله انگلیسی | نسخه تمام متن |
---|---|---|---|---|
4946951 | 1439561 | 2017 | 18 صفحه PDF | دانلود رایگان |
عنوان انگلیسی مقاله ISI
Addressing class-imbalance in multi-label learning via two-stage multi-label hypernetwork
دانلود مقاله + سفارش ترجمه
دانلود مقاله ISI انگلیسی
رایگان برای ایرانیان
کلمات کلیدی
موضوعات مرتبط
مهندسی و علوم پایه
مهندسی کامپیوتر
هوش مصنوعی
پیش نمایش صفحه اول مقاله
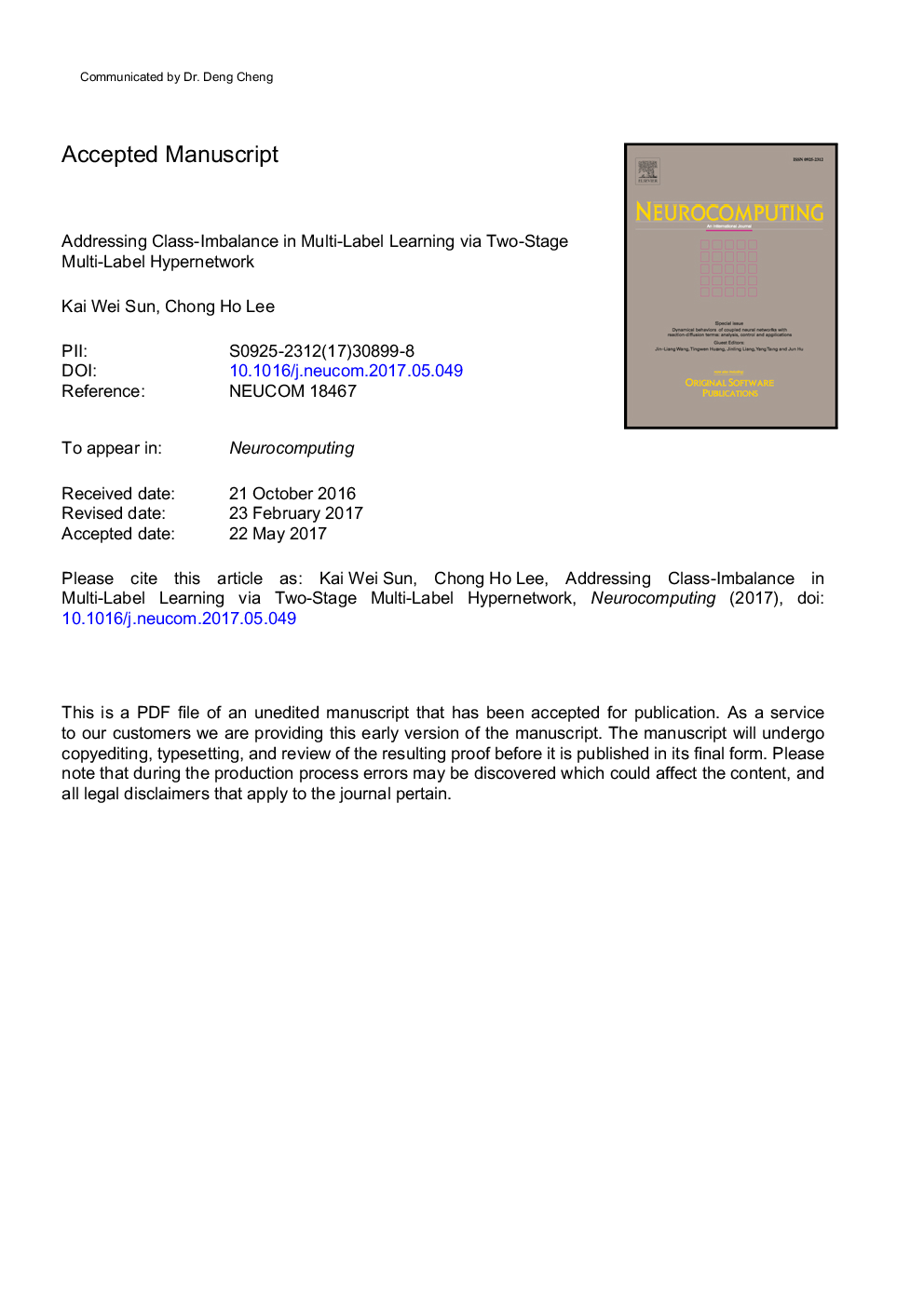
چکیده انگلیسی
Multi-label learning is concerned with learning from data examples that are represented by a single feature vector while associated with multiple labels simultaneously. Existing multi-label learning approaches mainly focus on exploiting label correlations to facilitate the learning process. However, an intrinsic characteristic of multi-label learning, i.e., class-imbalance has not been well studied. In this paper, we propose a two-stage multi-label hypernetwork (TSMLHN) to exploit label correlations and to make use of them to address the class-imbalance problem in multi-label learning. In TSMLHN, labels of a multi-label data set are divided into two groups, i.e., imbalanced labels and common labels based on their imbalance ratios. In the first stage of TSMLHN, we train a multi-label hypernetwork (MLHN) which generates basic predictions for all labels. In the second stage of TSMLHN, we train TSMLHN based on the predictions obtained from MLHN and utilize the correlations between common labels and imbalanced labels to improve the learning performance of imbalanced labels. Our proposed TSMLHN is conceptually simple, yet it is effective in addressing the class-imbalance problem in multi-label learning. Empirical studies on a broad range of multi-label data sets demonstrate that TSMLHN achieves competitive performance against state-of-the-art multi-label learning algorithms.
ناشر
Database: Elsevier - ScienceDirect (ساینس دایرکت)
Journal: Neurocomputing - Volume 266, 29 November 2017, Pages 375-389
Journal: Neurocomputing - Volume 266, 29 November 2017, Pages 375-389
نویسندگان
Kai Wei Sun, Chong Ho Lee,