کد مقاله | کد نشریه | سال انتشار | مقاله انگلیسی | نسخه تمام متن |
---|---|---|---|---|
4946962 | 1439561 | 2017 | 44 صفحه PDF | دانلود رایگان |
عنوان انگلیسی مقاله ISI
A new efficient training strategy for deep neural networks by hybridization of artificial bee colony and limited-memory BFGS optimization algorithms
دانلود مقاله + سفارش ترجمه
دانلود مقاله ISI انگلیسی
رایگان برای ایرانیان
کلمات کلیدی
موضوعات مرتبط
مهندسی و علوم پایه
مهندسی کامپیوتر
هوش مصنوعی
پیش نمایش صفحه اول مقاله
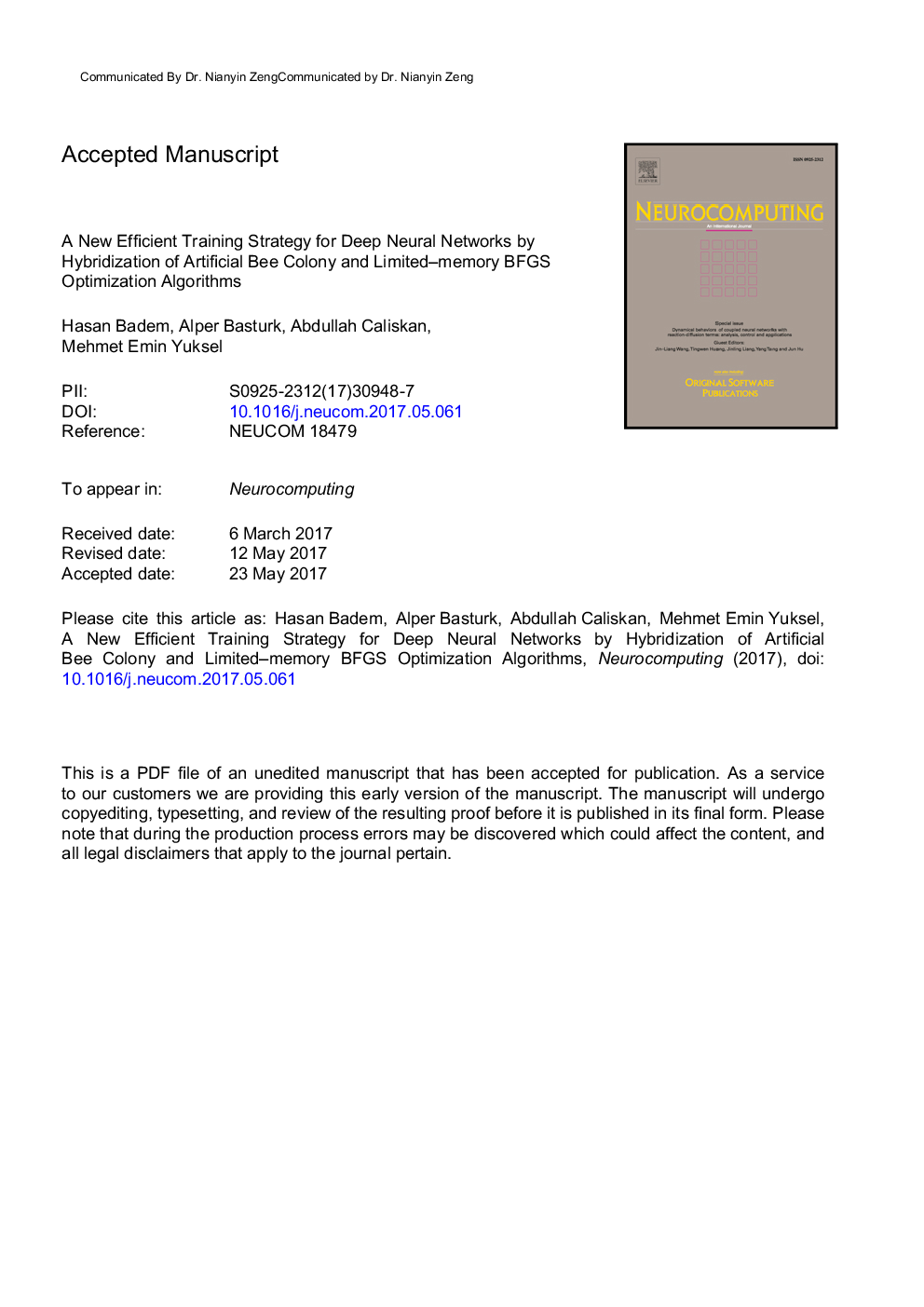
چکیده انگلیسی
Working up with deep learning techniques requires profound understanding of the mechanisms underlying the optimization of the internal parameters of complex structures. The major factor limiting this understanding is that there exist only a few optimization methods such as gradient descent and Limited-memory Broyden-Fletcher-Goldfarb-Shannon (L-BFGS) to find the best local minima of the problem space for these complex structures such as deep neural network (DNN). Therefore, in this paper, we represent a new training approach named hybrid artificial bee colony based training strategy (HABCbTS) to tune the parameters of a DNN structure, which includes one or more autoencoder layers cascaded to a softmax classification layer. In this strategy, a derivative-free optimization algorithm “ABC” is combined with a derivative-based algorithm “L-BFGS” to construct “HABC”, which is used in the HABCbTS. Detailed simulation results supported by statistical analysis show that the proposed training strategy results in better classification performance compared to the DNN classifier trained with the L-BFGS, ABC and modified ABC. The obtained classification results are also compared with the state-of-the-art classifiers, including MLP, SVM, KNN, DT and NB on 15 data sets with different dimensions and sizes.
ناشر
Database: Elsevier - ScienceDirect (ساینس دایرکت)
Journal: Neurocomputing - Volume 266, 29 November 2017, Pages 506-526
Journal: Neurocomputing - Volume 266, 29 November 2017, Pages 506-526
نویسندگان
Hasan Badem, Alper Basturk, Abdullah Caliskan, Mehmet Emin Yuksel,