کد مقاله | کد نشریه | سال انتشار | مقاله انگلیسی | نسخه تمام متن |
---|---|---|---|---|
4947033 | 1439560 | 2017 | 11 صفحه PDF | دانلود رایگان |
عنوان انگلیسی مقاله ISI
A kernelized non-parametric classifier based on feature ranking in anisotropic Gaussian kernel
دانلود مقاله + سفارش ترجمه
دانلود مقاله ISI انگلیسی
رایگان برای ایرانیان
کلمات کلیدی
موضوعات مرتبط
مهندسی و علوم پایه
مهندسی کامپیوتر
هوش مصنوعی
پیش نمایش صفحه اول مقاله
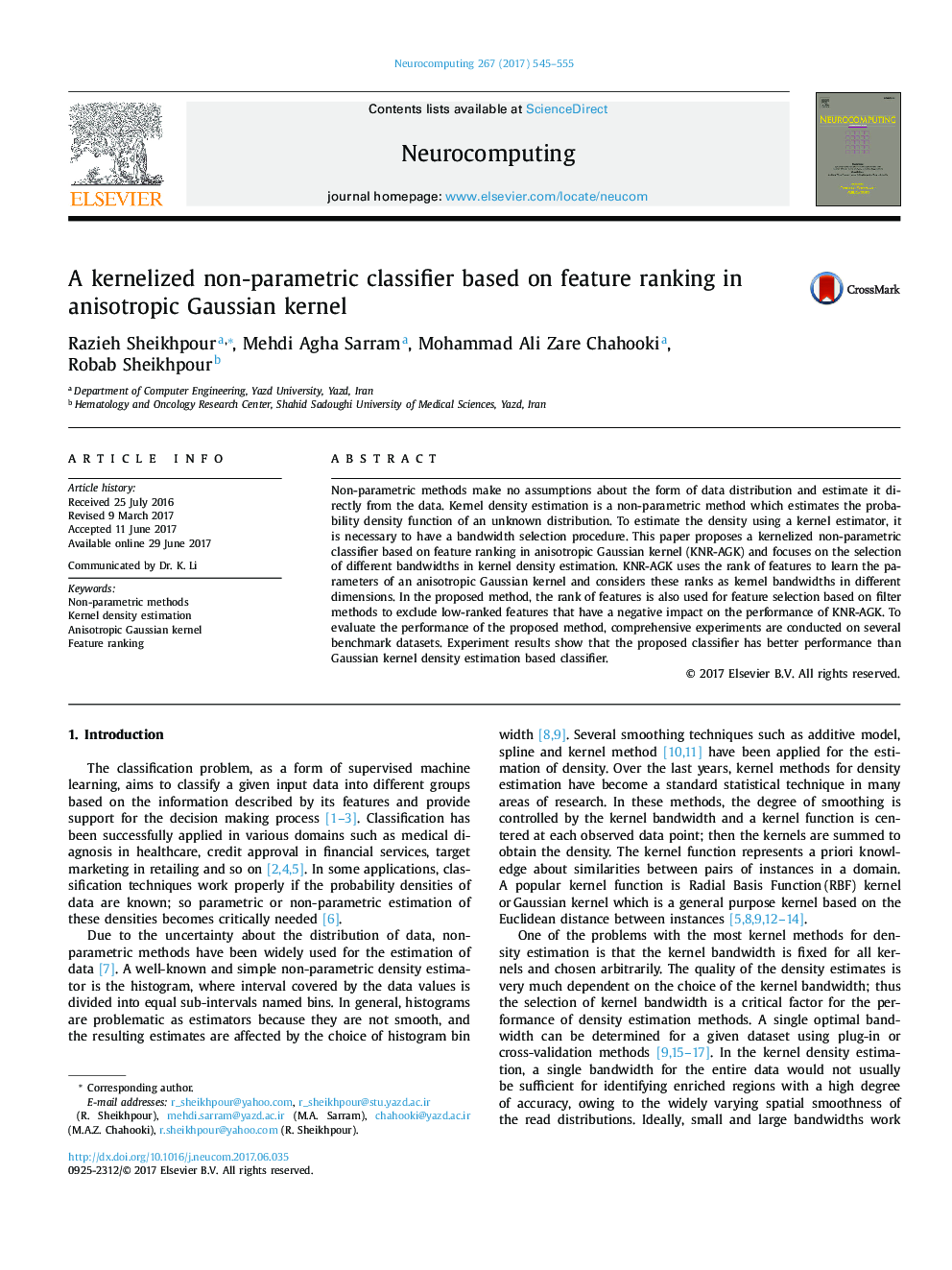
چکیده انگلیسی
Non-parametric methods make no assumptions about the form of data distribution and estimate it directly from the data. Kernel density estimation is a non-parametric method which estimates the probability density function of an unknown distribution. To estimate the density using a kernel estimator, it is necessary to have a bandwidth selection procedure. This paper proposes a kernelized non-parametric classifier based on feature ranking in anisotropic Gaussian kernel (KNR-AGK) and focuses on the selection of different bandwidths in kernel density estimation. KNR-AGK uses the rank of features to learn the parameters of an anisotropic Gaussian kernel and considers these ranks as kernel bandwidths in different dimensions. In the proposed method, the rank of features is also used for feature selection based on filter methods to exclude low-ranked features that have a negative impact on the performance of KNR-AGK. To evaluate the performance of the proposed method, comprehensive experiments are conducted on several benchmark datasets. Experiment results show that the proposed classifier has better performance than Gaussian kernel density estimation based classifier.
ناشر
Database: Elsevier - ScienceDirect (ساینس دایرکت)
Journal: Neurocomputing - Volume 267, 6 December 2017, Pages 545-555
Journal: Neurocomputing - Volume 267, 6 December 2017, Pages 545-555
نویسندگان
Razieh Sheikhpour, Mehdi Agha Sarram, Mohammad Ali Zare Chahooki, Robab Sheikhpour,