کد مقاله | کد نشریه | سال انتشار | مقاله انگلیسی | نسخه تمام متن |
---|---|---|---|---|
4947494 | 1439584 | 2017 | 24 صفحه PDF | دانلود رایگان |
عنوان انگلیسی مقاله ISI
Low-level structure feature extraction for image processing via stacked sparse denoising autoencoder
دانلود مقاله + سفارش ترجمه
دانلود مقاله ISI انگلیسی
رایگان برای ایرانیان
کلمات کلیدی
موضوعات مرتبط
مهندسی و علوم پایه
مهندسی کامپیوتر
هوش مصنوعی
پیش نمایش صفحه اول مقاله
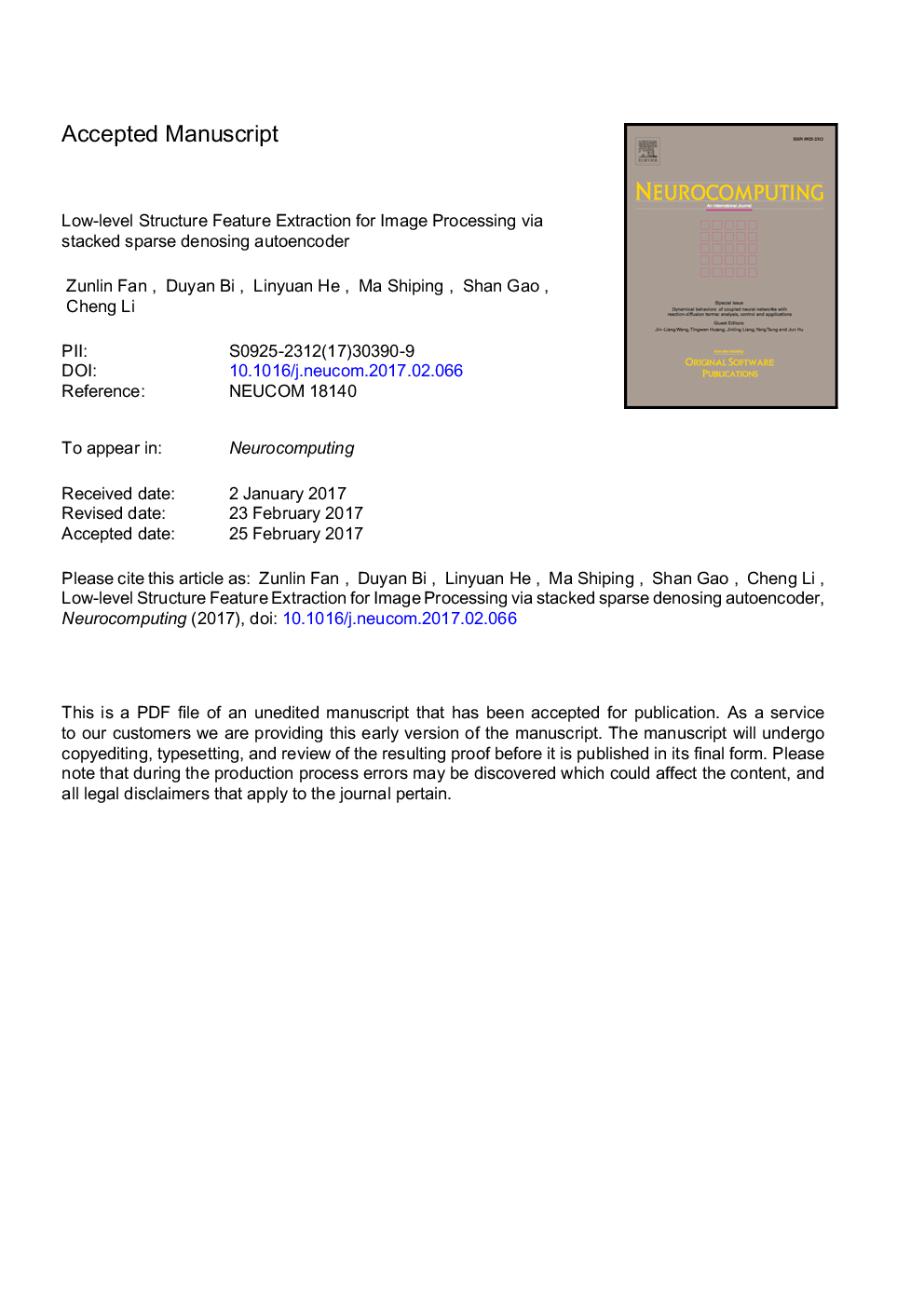
چکیده انگلیسی
In this paper, we propose a novel low-level structure feature extraction for image processing based on deep neural network, stacked sparse denoising autoencoder (SSDA). The current image processing methods via deep learning are directly building and learning the end-to-end mappings between the input/output. Instead, we advocate the analysis of the first layer learning features from input data. With the learned low-level structure features, we improve two edge-preserving filters that are key to image processing tasks such as denoising, High Dynamic Range (HDR) compression and details enhancement. Due to the validity and superiority of the proposed feature extraction, the results computed by the two improved filters do not suffer from the drawbacks including halos, edge blurring, noise amplification and over-enhancement. More importantly, we demonstrate that the features trained from natural images are not specific and can extract the structure features of infrared images. Hence, it is feasible to handle tasks by using the trained features directly.
ناشر
Database: Elsevier - ScienceDirect (ساینس دایرکت)
Journal: Neurocomputing - Volume 243, 21 June 2017, Pages 12-20
Journal: Neurocomputing - Volume 243, 21 June 2017, Pages 12-20
نویسندگان
Zunlin Fan, Duyan Bi, Linyuan He, Ma Shiping, Shan Gao, Cheng Li,