کد مقاله | کد نشریه | سال انتشار | مقاله انگلیسی | نسخه تمام متن |
---|---|---|---|---|
4948146 | 1439609 | 2016 | 10 صفحه PDF | دانلود رایگان |
عنوان انگلیسی مقاله ISI
Joint diversity regularization and graph regularization for multiple kernel k-means clustering via latent variables
دانلود مقاله + سفارش ترجمه
دانلود مقاله ISI انگلیسی
رایگان برای ایرانیان
کلمات کلیدی
موضوعات مرتبط
مهندسی و علوم پایه
مهندسی کامپیوتر
هوش مصنوعی
پیش نمایش صفحه اول مقاله
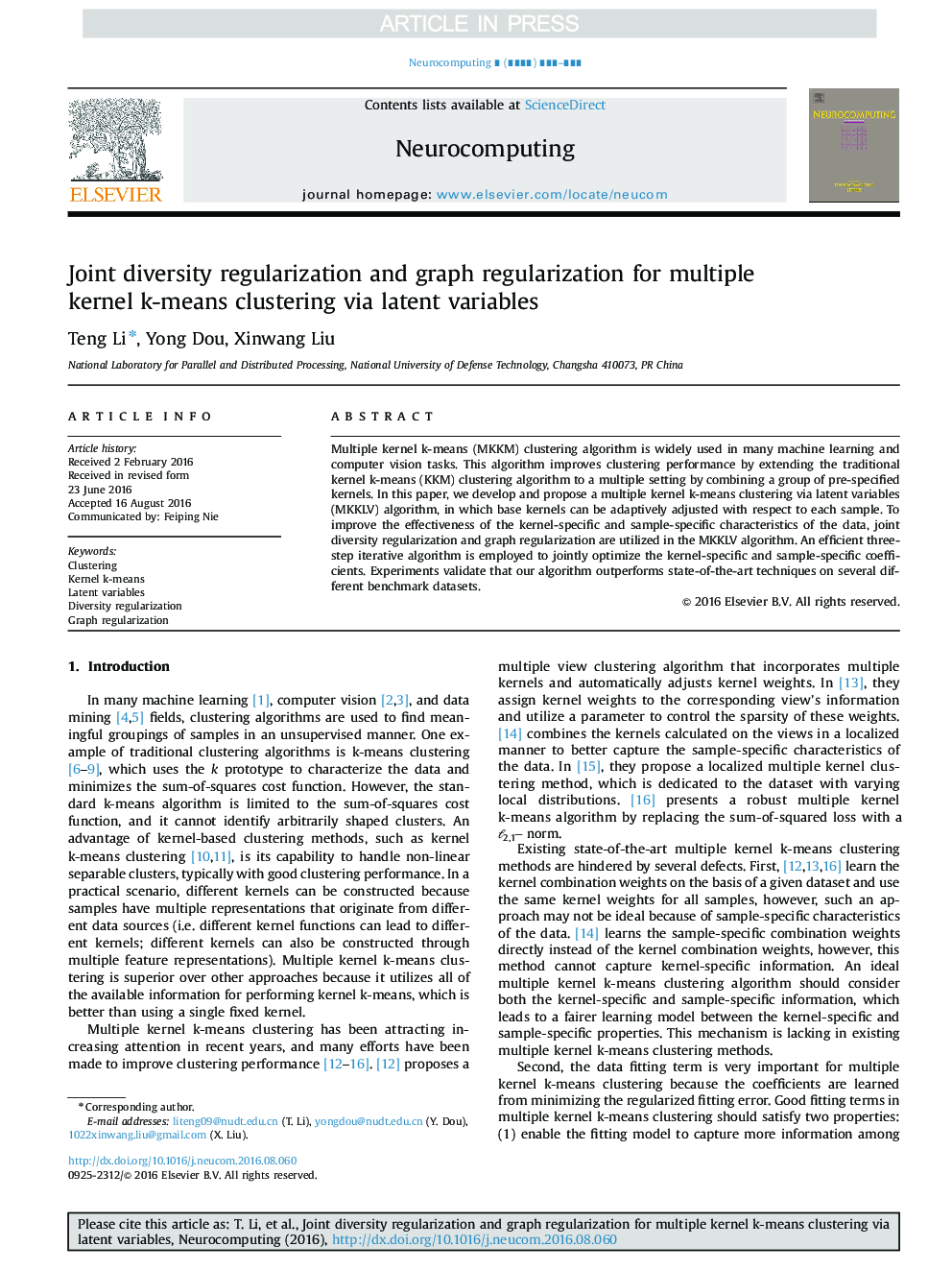
چکیده انگلیسی
Multiple kernel k-means (MKKM) clustering algorithm is widely used in many machine learning and computer vision tasks. This algorithm improves clustering performance by extending the traditional kernel k-means (KKM) clustering algorithm to a multiple setting by combining a group of pre-specified kernels. In this paper, we develop and propose a multiple kernel k-means clustering via latent variables (MKKLV) algorithm, in which base kernels can be adaptively adjusted with respect to each sample. To improve the effectiveness of the kernel-specific and sample-specific characteristics of the data, joint diversity regularization and graph regularization are utilized in the MKKLV algorithm. An efficient three-step iterative algorithm is employed to jointly optimize the kernel-specific and sample-specific coefficients. Experiments validate that our algorithm outperforms state-of-the-art techniques on several different benchmark datasets.
ناشر
Database: Elsevier - ScienceDirect (ساینس دایرکت)
Journal: Neurocomputing - Volume 218, 19 December 2016, Pages 154-163
Journal: Neurocomputing - Volume 218, 19 December 2016, Pages 154-163
نویسندگان
Teng Li, Yong Dou, Xinwang Liu,