کد مقاله | کد نشریه | سال انتشار | مقاله انگلیسی | نسخه تمام متن |
---|---|---|---|---|
4948346 | 1439611 | 2016 | 9 صفحه PDF | دانلود رایگان |
عنوان انگلیسی مقاله ISI
Topic detection and tracking for conversational content by using conceptual dynamic latent Dirichlet allocation
دانلود مقاله + سفارش ترجمه
دانلود مقاله ISI انگلیسی
رایگان برای ایرانیان
کلمات کلیدی
موضوعات مرتبط
مهندسی و علوم پایه
مهندسی کامپیوتر
هوش مصنوعی
پیش نمایش صفحه اول مقاله
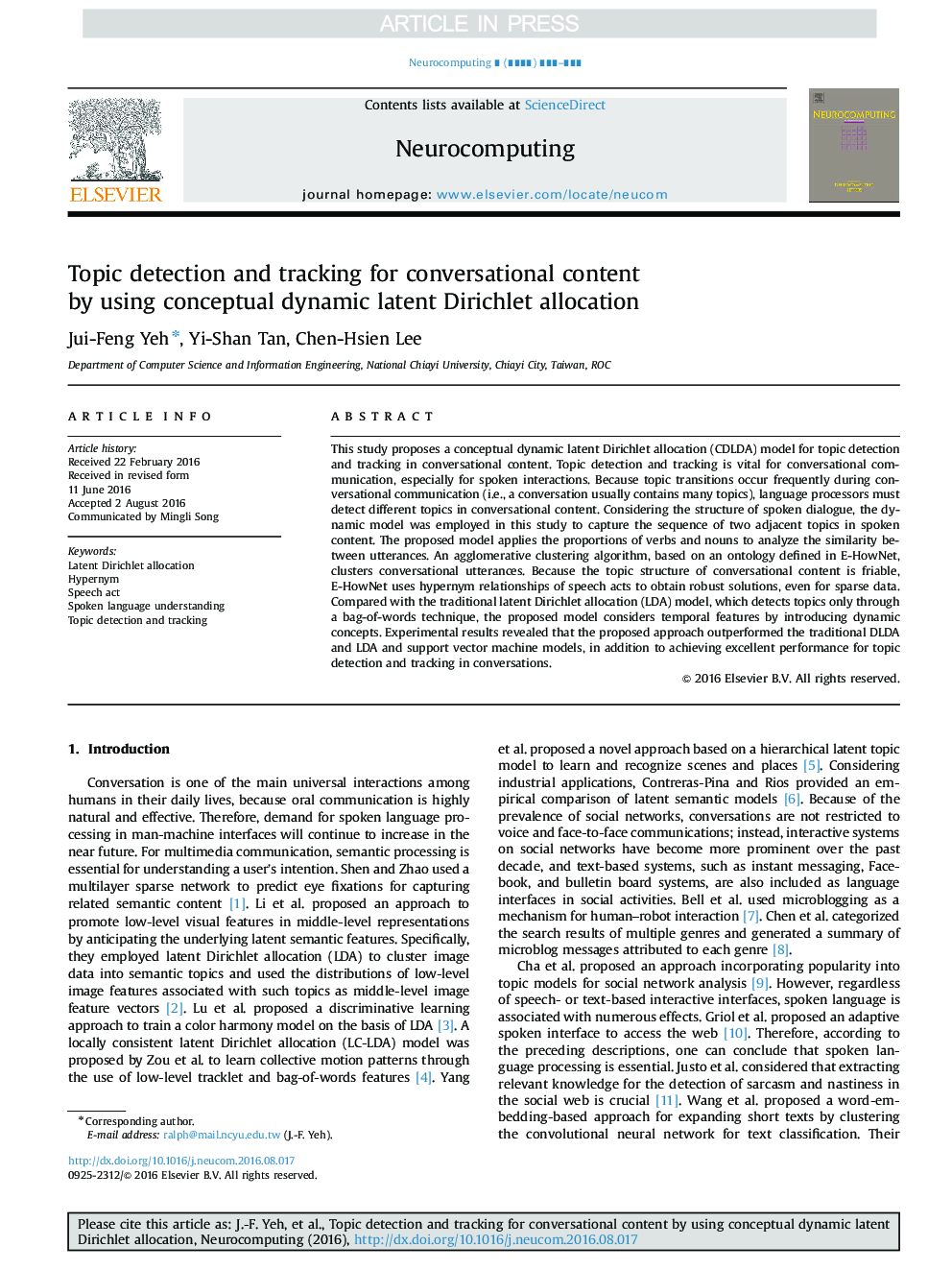
چکیده انگلیسی
This study proposes a conceptual dynamic latent Dirichlet allocation (CDLDA) model for topic detection and tracking in conversational content. Topic detection and tracking is vital for conversational communication, especially for spoken interactions. Because topic transitions occur frequently during conversational communication (i.e., a conversation usually contains many topics), language processors must detect different topics in conversational content. Considering the structure of spoken dialogue, the dynamic model was employed in this study to capture the sequence of two adjacent topics in spoken content. The proposed model applies the proportions of verbs and nouns to analyze the similarity between utterances. An agglomerative clustering algorithm, based on an ontology defined in E-HowNet, clusters conversational utterances. Because the topic structure of conversational content is friable, E-HowNet uses hypernym relationships of speech acts to obtain robust solutions, even for sparse data. Compared with the traditional latent Dirichlet allocation (LDA) model, which detects topics only through a bag-of-words technique, the proposed model considers temporal features by introducing dynamic concepts. Experimental results revealed that the proposed approach outperformed the traditional DLDA and LDA and support vector machine models, in addition to achieving excellent performance for topic detection and tracking in conversations.
ناشر
Database: Elsevier - ScienceDirect (ساینس دایرکت)
Journal: Neurocomputing - Volume 216, 5 December 2016, Pages 310-318
Journal: Neurocomputing - Volume 216, 5 December 2016, Pages 310-318
نویسندگان
Jui-Feng Yeh, Yi-Shan Tan, Chen-Hsien Lee,