کد مقاله | کد نشریه | سال انتشار | مقاله انگلیسی | نسخه تمام متن |
---|---|---|---|---|
4948394 | 1439611 | 2016 | 48 صفحه PDF | دانلود رایگان |
عنوان انگلیسی مقاله ISI
LWCR: multi-Layered Wikipedia representation for Computing word Relatedness
دانلود مقاله + سفارش ترجمه
دانلود مقاله ISI انگلیسی
رایگان برای ایرانیان
کلمات کلیدی
موضوعات مرتبط
مهندسی و علوم پایه
مهندسی کامپیوتر
هوش مصنوعی
پیش نمایش صفحه اول مقاله
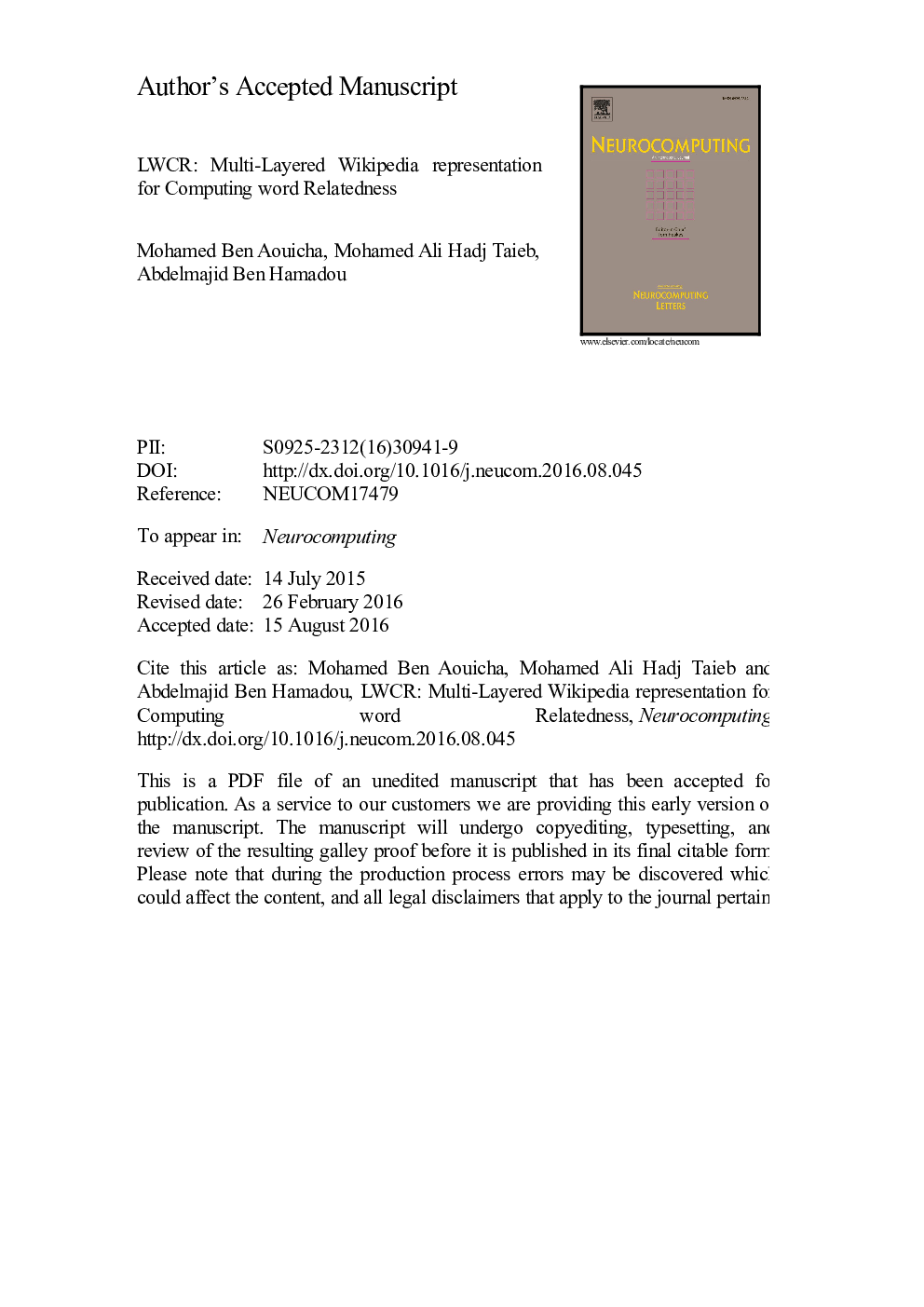
چکیده انگلیسی
The measurement of the semantic relatedness between words has gained increasing interest in several research fields, including cognitive science, artificial intelligence, biology, and linguistics. The development of efficient measures is based on knowledge resources, such as Wikipedia, a huge and living encyclopedia supplied by net surfers. In this paper, we propose a novel approach based on multi-Layered Wikipedia representation for Computing word Relatedness (LWCR) exploiting a weighting scheme based on Wikipedia Category Graph (WCG): Term Frequency-Inverse Category Frequency (tfxicf). Our proposal provides for each category pertaining to the WCG a Category Description Vector (CDV) including the weights of stems extracted from articles assigned to a category. The semantic relatedness degree is computed using the cosine measure between the CDVs assigned to the target words couple. The basic idea is followed by enhancement modules exploiting other Wikipedia features, such as article titles, redirection mechanism, and neighborhood category enrichment, to exploit semantic features and better quantify the semantic relatedness between words. To the best of our knowledge, this is the first attempt to incorporate the WCG-based term-weighting scheme (tfxicf) into computing model of semantic relatedness. It is also the first work that exploits 17 datasets in the assessment process, which are divided into two sets. The first set includes the ones designed for semantic similarity purposes: RG65, MC30, AG203, WP300, SimLexNoun666 and GeReSiD50Sim; the second includes datasets for semantic relatedness evaluation: WordSim353, GM30, Zeigler25, Zeigler30, MTurk287, MTurk771, MEN3000, Rel122, ReWord26, GeReSiD50 and SCWS1229. The found results are compared to WordNet-based measures and distributional measures cosine and PMI performed on Wikipedia articles. Experiments show that our approach provides consistent improvements over the state of the art results on multiple benchmarks.
ناشر
Database: Elsevier - ScienceDirect (ساینس دایرکت)
Journal: Neurocomputing - Volume 216, 5 December 2016, Pages 816-843
Journal: Neurocomputing - Volume 216, 5 December 2016, Pages 816-843
نویسندگان
Mohamed Ben Aouicha, Mohamed Ali Hadj Taieb, Abdelmajid Ben Hamadou,