کد مقاله | کد نشریه | سال انتشار | مقاله انگلیسی | نسخه تمام متن |
---|---|---|---|---|
4948460 | 1439613 | 2016 | 14 صفحه PDF | دانلود رایگان |
عنوان انگلیسی مقاله ISI
Learning iterative quantization binary codes for face recognition
دانلود مقاله + سفارش ترجمه
دانلود مقاله ISI انگلیسی
رایگان برای ایرانیان
کلمات کلیدی
موضوعات مرتبط
مهندسی و علوم پایه
مهندسی کامپیوتر
هوش مصنوعی
پیش نمایش صفحه اول مقاله
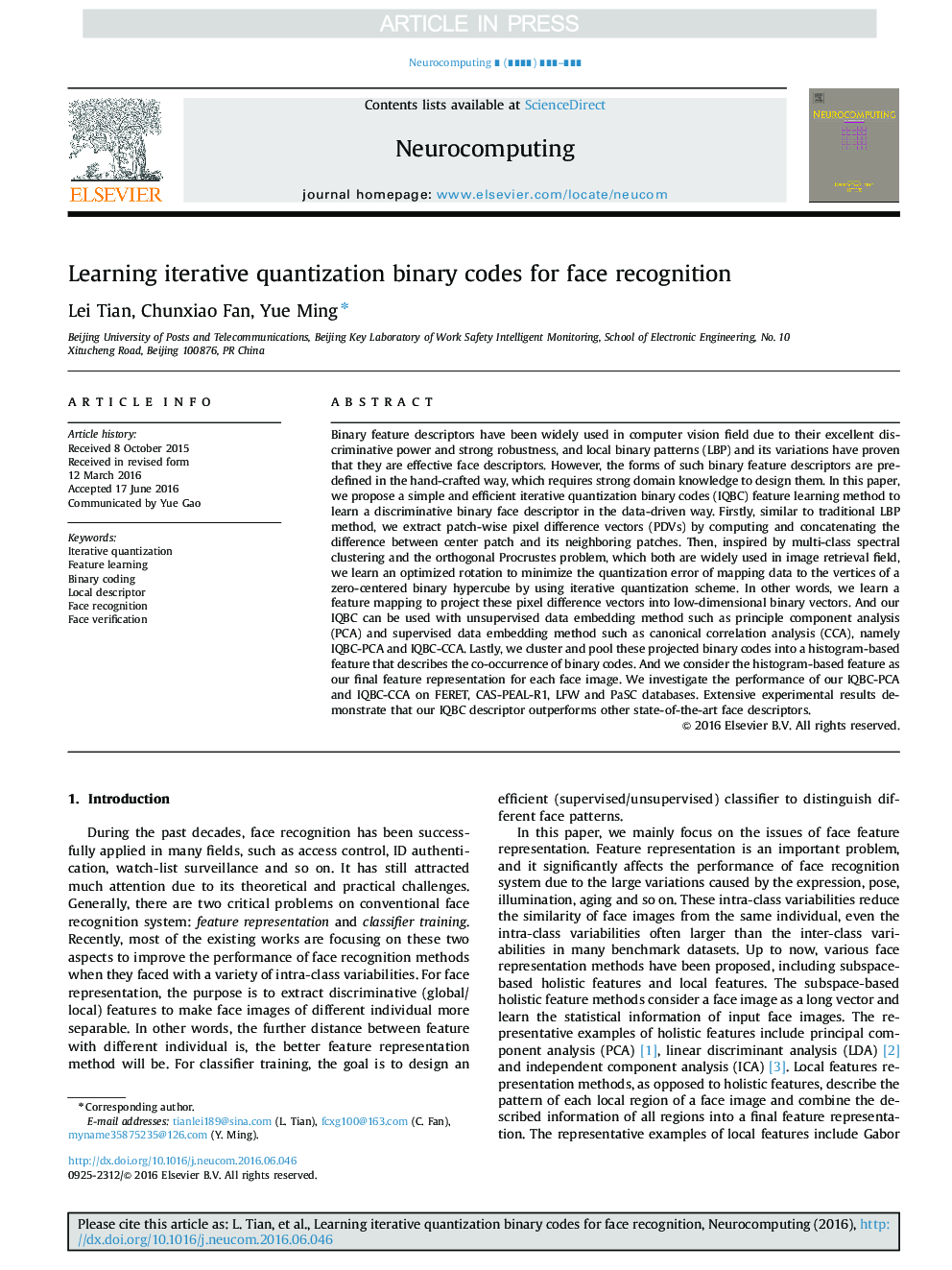
چکیده انگلیسی
Binary feature descriptors have been widely used in computer vision field due to their excellent discriminative power and strong robustness, and local binary patterns (LBP) and its variations have proven that they are effective face descriptors. However, the forms of such binary feature descriptors are predefined in the hand-crafted way, which requires strong domain knowledge to design them. In this paper, we propose a simple and efficient iterative quantization binary codes (IQBC) feature learning method to learn a discriminative binary face descriptor in the data-driven way. Firstly, similar to traditional LBP method, we extract patch-wise pixel difference vectors (PDVs) by computing and concatenating the difference between center patch and its neighboring patches. Then, inspired by multi-class spectral clustering and the orthogonal Procrustes problem, which both are widely used in image retrieval field, we learn an optimized rotation to minimize the quantization error of mapping data to the vertices of a zero-centered binary hypercube by using iterative quantization scheme. In other words, we learn a feature mapping to project these pixel difference vectors into low-dimensional binary vectors. And our IQBC can be used with unsupervised data embedding method such as principle component analysis (PCA) and supervised data embedding method such as canonical correlation analysis (CCA), namely IQBC-PCA and IQBC-CCA. Lastly, we cluster and pool these projected binary codes into a histogram-based feature that describes the co-occurrence of binary codes. And we consider the histogram-based feature as our final feature representation for each face image. We investigate the performance of our IQBC-PCA and IQBC-CCA on FERET, CAS-PEAL-R1, LFW and PaSC databases. Extensive experimental results demonstrate that our IQBC descriptor outperforms other state-of-the-art face descriptors.
ناشر
Database: Elsevier - ScienceDirect (ساینس دایرکت)
Journal: Neurocomputing - Volume 214, 19 November 2016, Pages 629-642
Journal: Neurocomputing - Volume 214, 19 November 2016, Pages 629-642
نویسندگان
Lei Tian, Chunxiao Fan, Yue Ming,