کد مقاله | کد نشریه | سال انتشار | مقاله انگلیسی | نسخه تمام متن |
---|---|---|---|---|
4948717 | 1439850 | 2017 | 34 صفحه PDF | دانلود رایگان |
عنوان انگلیسی مقاله ISI
Improving the speed of convergence of multi-agent Q-learning for cooperative task-planning by a robot-team
دانلود مقاله + سفارش ترجمه
دانلود مقاله ISI انگلیسی
رایگان برای ایرانیان
کلمات کلیدی
موضوعات مرتبط
مهندسی و علوم پایه
مهندسی کامپیوتر
هوش مصنوعی
پیش نمایش صفحه اول مقاله
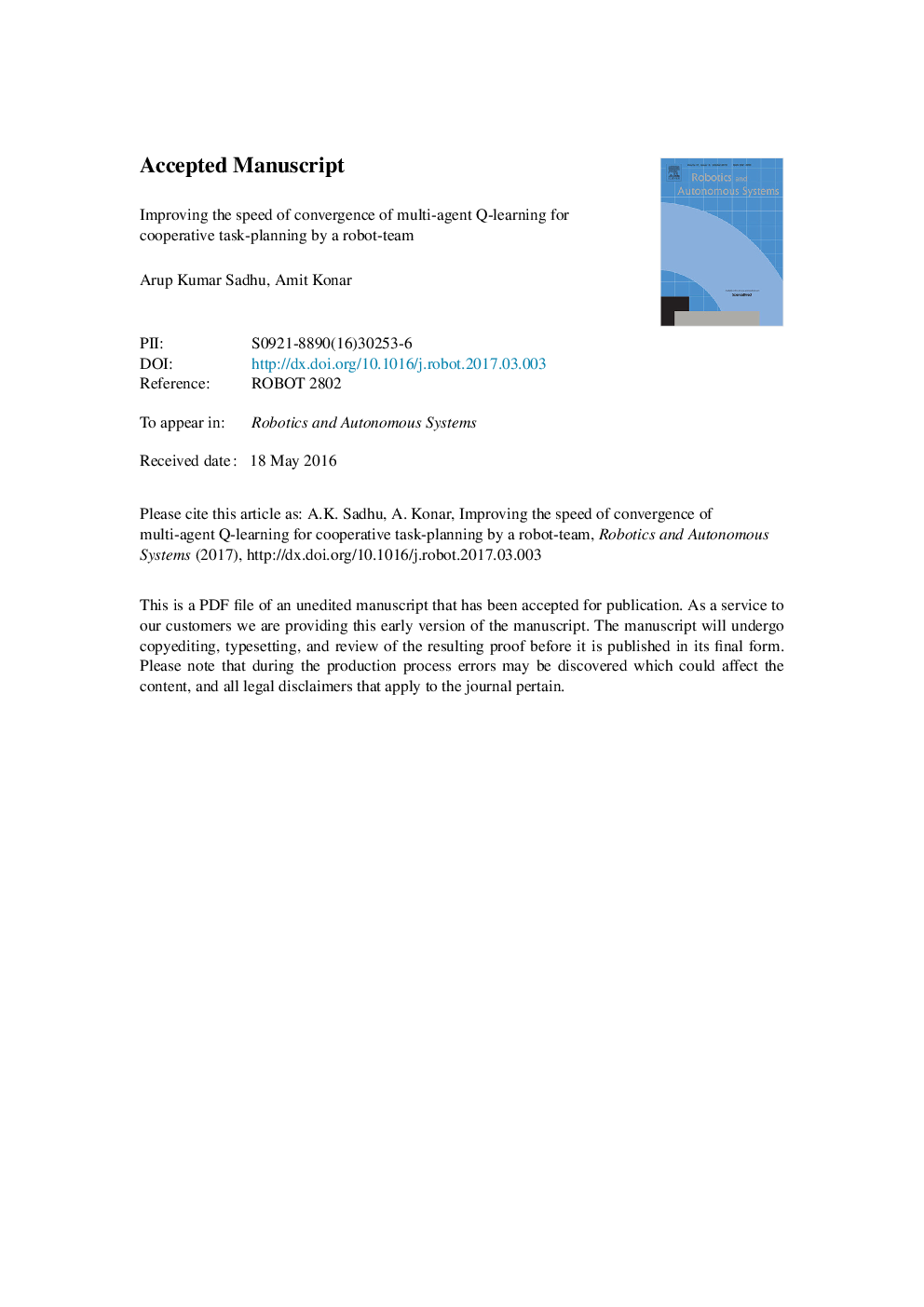
چکیده انگلیسی
Learning-based planning algorithms are currently gaining popularity for their increasing applications in real-time planning and cooperation of robots. The paper aims at extending traditional multi-agent Q-learning algorithms to improve their speed of convergence by incorporating two interesting properties, concerning (i) exploration of the team-goal and (ii) selection of joint action at a given joint state. The exploration of team-goal is realized by allowing the agents, capable of reaching their goals, to wait at their individual goal states, until remaining agents explore their individual goals synchronously or asynchronously. To avoid unwanted never-ending wait-loops, an upper bound to wait-interval, obtained empirically for the waiting team members, is introduced. Selection of joint action, which is a crucial problem in traditional multi-agent Q-learning, is performed here by taking the intersection of individual preferred joint actions of all the agents. In case the resulting intersection is a null set, the individual actions are selected randomly or otherwise following classical multi-agent Q-learning. It is shown both theoretically and experimentally that the extended algorithms outperform its traditional counterpart with respect to speed of convergence. To ensure selection of right joint action at each step of planning, we offer high rewards to exploration of the team-goal and zero rewards to exploration of individual goals during the learning phase. The introduction of the above strategy results in an enriched joint Q-table, the consultation of which during the multi-agent planning yields significant improvement in the performance of cooperative planning of robots. Hardwired realization of the proposed learning based planning algorithm, designed for object-transportation application, confirms the relative merits of the proposed technique over contestant algorithms.
ناشر
Database: Elsevier - ScienceDirect (ساینس دایرکت)
Journal: Robotics and Autonomous Systems - Volume 92, June 2017, Pages 66-80
Journal: Robotics and Autonomous Systems - Volume 92, June 2017, Pages 66-80
نویسندگان
Arup Kumar Sadhu, Amit Konar,