کد مقاله | کد نشریه | سال انتشار | مقاله انگلیسی | نسخه تمام متن |
---|---|---|---|---|
4951054 | 1441167 | 2017 | 11 صفحه PDF | دانلود رایگان |
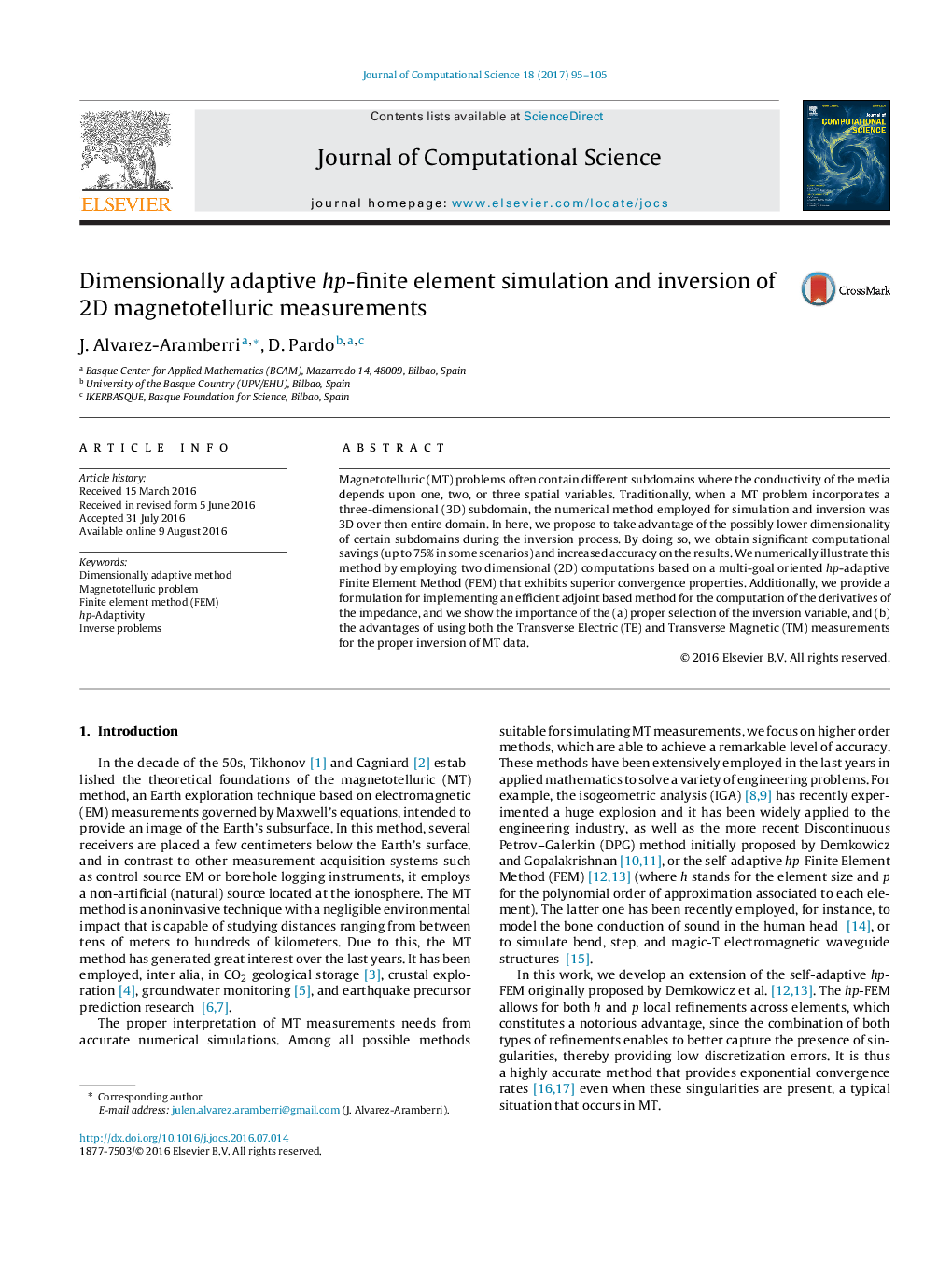
- We propose a dimensionally adaptive method for the inversion and we show the gains of this approach.
- We illustrate the fast convergence of the multi-goal oriented self-adaptive hp-FEM in terms of the error vs. the problem size, as expected from the theory.
- We provide a formulation for implementing an efficient adjoint based method for the computation of the derivatives of the impedance (a non-linear Quantity of Interest (QoI)) in terms of linear QoI.
Magnetotelluric (MT) problems often contain different subdomains where the conductivity of the media depends upon one, two, or three spatial variables. Traditionally, when a MT problem incorporates a three-dimensional (3D) subdomain, the numerical method employed for simulation and inversion was 3D over then entire domain. In here, we propose to take advantage of the possibly lower dimensionality of certain subdomains during the inversion process. By doing so, we obtain significant computational savings (up to 75% in some scenarios) and increased accuracy on the results. We numerically illustrate this method by employing two dimensional (2D) computations based on a multi-goal oriented hp-adaptive Finite Element Method (FEM) that exhibits superior convergence properties. Additionally, we provide a formulation for implementing an efficient adjoint based method for the computation of the derivatives of the impedance, and we show the importance of the (a) proper selection of the inversion variable, and (b) the advantages of using both the Transverse Electric (TE) and Transverse Magnetic (TM) measurements for the proper inversion of MT data.
Journal: Journal of Computational Science - Volume 18, January 2017, Pages 95-105