کد مقاله | کد نشریه | سال انتشار | مقاله انگلیسی | نسخه تمام متن |
---|---|---|---|---|
495180 | 862817 | 2015 | 12 صفحه PDF | دانلود رایگان |
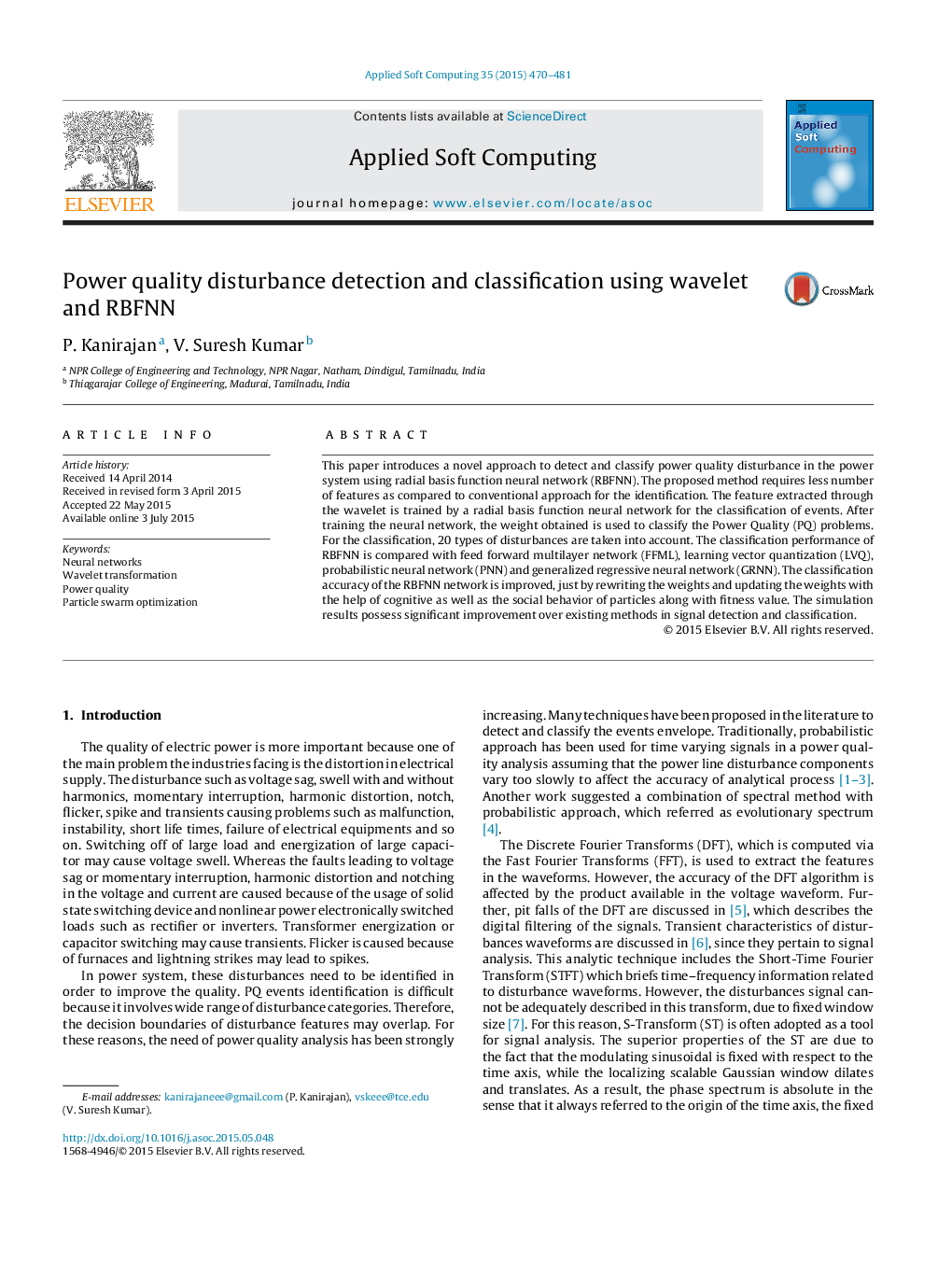
• The power quality disturbance detection and classification is important for improving the power quality.
• Various disturbances were taken in to account, over 20 events.
• Wavelet is used to extract features from a MATLAB simulated disturbance waveforms.
• Radial Basis Function Neural Network has been used to detect and classify the disturbance and compared with other considered approach.
• The entire work has been presented with particle swarm optimization.
This paper introduces a novel approach to detect and classify power quality disturbance in the power system using radial basis function neural network (RBFNN). The proposed method requires less number of features as compared to conventional approach for the identification. The feature extracted through the wavelet is trained by a radial basis function neural network for the classification of events. After training the neural network, the weight obtained is used to classify the Power Quality (PQ) problems. For the classification, 20 types of disturbances are taken into account. The classification performance of RBFNN is compared with feed forward multilayer network (FFML), learning vector quantization (LVQ), probabilistic neural network (PNN) and generalized regressive neural network (GRNN). The classification accuracy of the RBFNN network is improved, just by rewriting the weights and updating the weights with the help of cognitive as well as the social behavior of particles along with fitness value. The simulation results possess significant improvement over existing methods in signal detection and classification.
Figure optionsDownload as PowerPoint slide
Journal: Applied Soft Computing - Volume 35, October 2015, Pages 470–481