کد مقاله | کد نشریه | سال انتشار | مقاله انگلیسی | نسخه تمام متن |
---|---|---|---|---|
495322 | 862823 | 2014 | 13 صفحه PDF | دانلود رایگان |
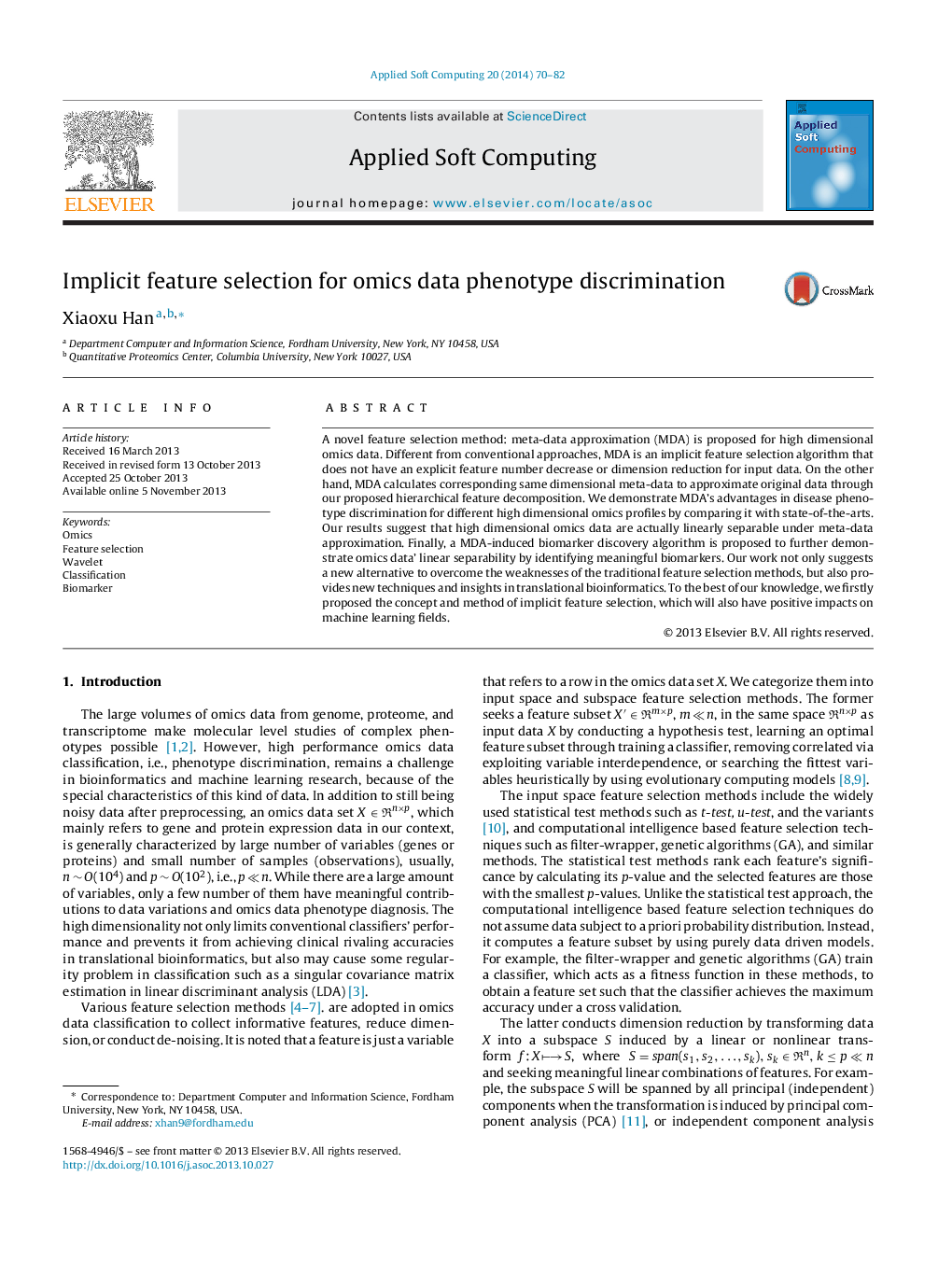
A novel feature selection method: meta-data approximation (MDA) is proposed for high dimensional omics data. Different from conventional approaches, MDA is an implicit feature selection algorithm that does not have an explicit feature number decrease or dimension reduction for input data. On the other hand, MDA calculates corresponding same dimensional meta-data to approximate original data through our proposed hierarchical feature decomposition. We demonstrate MDA's advantages in disease phenotype discrimination for different high dimensional omics profiles by comparing it with state-of-the-arts. Our results suggest that high dimensional omics data are actually linearly separable under meta-data approximation. Finally, a MDA-induced biomarker discovery algorithm is proposed to further demonstrate omics data’ linear separability by identifying meaningful biomarkers. Our work not only suggests a new alternative to overcome the weaknesses of the traditional feature selection methods, but also provides new techniques and insights in translational bioinformatics. To the best of our knowledge, we firstly proposed the concept and method of implicit feature selection, which will also have positive impacts on machine learning fields.
Separating 72 normal, 78 HCC, and 51 cirrhotic samples in Cirrhosis3 data (23,846 × 201) by using their three top-ranked biomarkers. Figure optionsDownload as PowerPoint slide
Journal: Applied Soft Computing - Volume 20, July 2014, Pages 70–82