کد مقاله | کد نشریه | سال انتشار | مقاله انگلیسی | نسخه تمام متن |
---|---|---|---|---|
4953445 | 1443011 | 2017 | 18 صفحه PDF | دانلود رایگان |
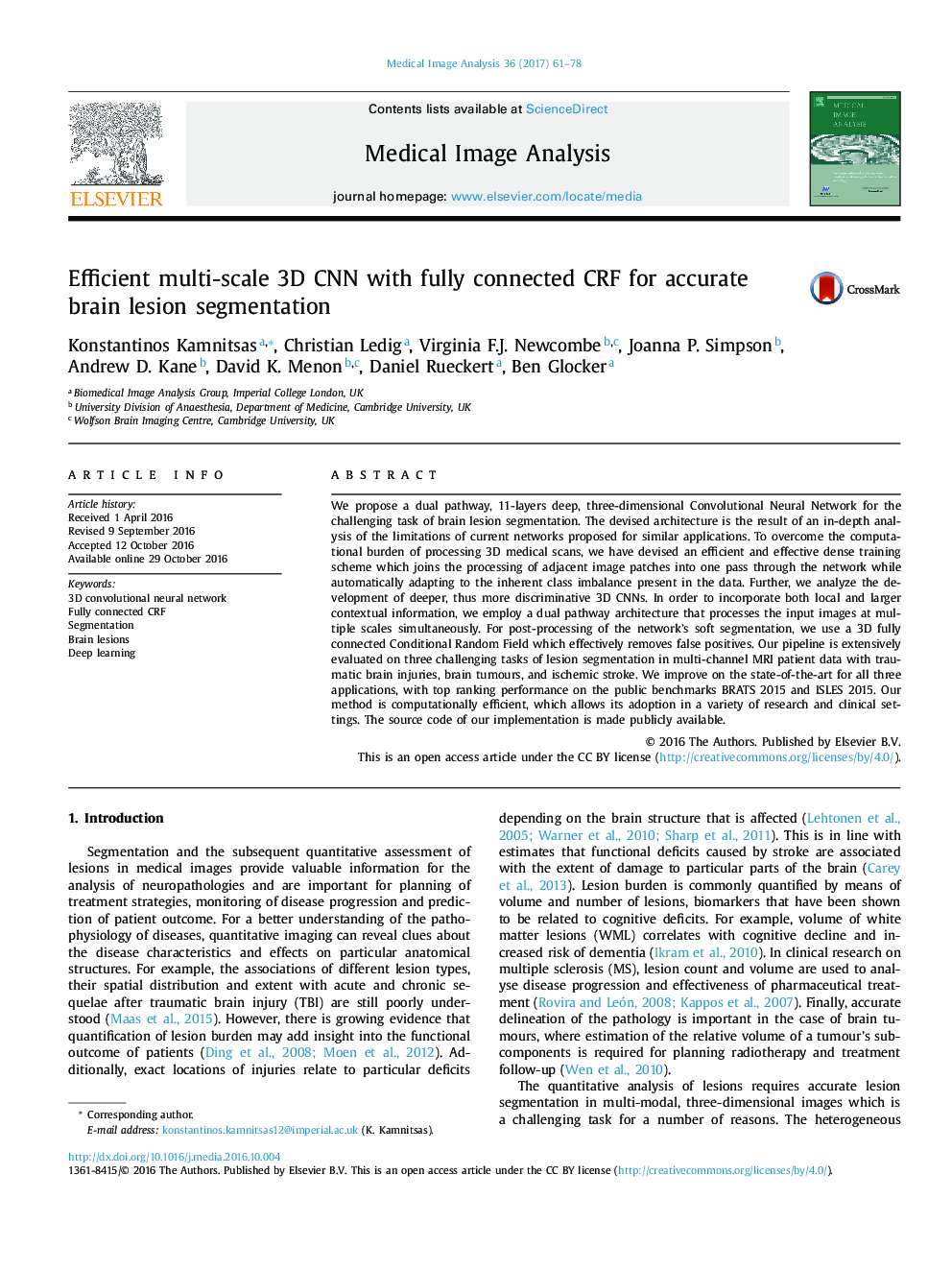
- An efficient 11-layers deep, multi-scale, 3D CNN architecture.
- A novel training strategy that significantly boosts performance.
- The first employment of a 3D fully connected CRF for post-processing.
- State-of-the-art performance on three challenging lesion segmentation tasks.
- New insights into the automatically learned intermediate representations.
We propose a dual pathway, 11-layers deep, three-dimensional Convolutional Neural Network for the challenging task of brain lesion segmentation. The devised architecture is the result of an in-depth analysis of the limitations of current networks proposed for similar applications. To overcome the computational burden of processing 3D medical scans, we have devised an efficient and effective dense training scheme which joins the processing of adjacent image patches into one pass through the network while automatically adapting to the inherent class imbalance present in the data. Further, we analyze the development of deeper, thus more discriminative 3D CNNs. In order to incorporate both local and larger contextual information, we employ a dual pathway architecture that processes the input images at multiple scales simultaneously. For post-processing of the network's soft segmentation, we use a 3D fully connected Conditional Random Field which effectively removes false positives. Our pipeline is extensively evaluated on three challenging tasks of lesion segmentation in multi-channel MRI patient data with traumatic brain injuries, brain tumours, and ischemic stroke. We improve on the state-of-the-art for all three applications, with top ranking performance on the public benchmarks BRATS 2015 and ISLES 2015. Our method is computationally efficient, which allows its adoption in a variety of research and clinical settings. The source code of our implementation is made publicly available.
155
Journal: Medical Image Analysis - Volume 36, February 2017, Pages 61-78