کد مقاله | کد نشریه | سال انتشار | مقاله انگلیسی | نسخه تمام متن |
---|---|---|---|---|
495700 | 862834 | 2014 | 9 صفحه PDF | دانلود رایگان |
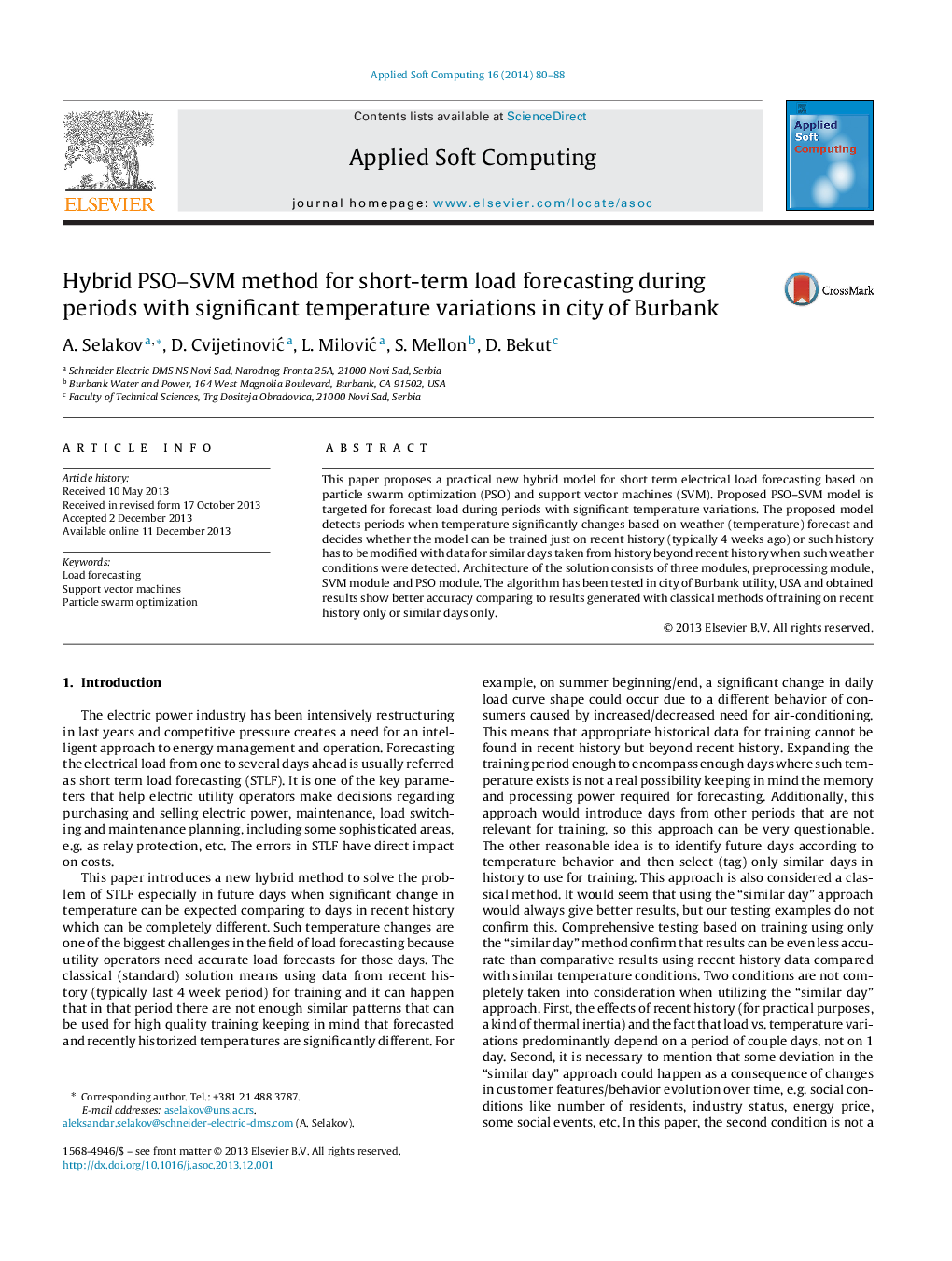
• Hybrid forecast model that includes recent history and similar day.
• Day tagging based on temperature integral.
• We detect switching events when recent history does not provide quality data for training.
• Real life data from Burbank utility.
This paper proposes a practical new hybrid model for short term electrical load forecasting based on particle swarm optimization (PSO) and support vector machines (SVM). Proposed PSO–SVM model is targeted for forecast load during periods with significant temperature variations. The proposed model detects periods when temperature significantly changes based on weather (temperature) forecast and decides whether the model can be trained just on recent history (typically 4 weeks ago) or such history has to be modified with data for similar days taken from history beyond recent history when such weather conditions were detected. Architecture of the solution consists of three modules, preprocessing module, SVM module and PSO module. The algorithm has been tested in city of Burbank utility, USA and obtained results show better accuracy comparing to results generated with classical methods of training on recent history only or similar days only.
Figure optionsDownload as PowerPoint slide
Journal: Applied Soft Computing - Volume 16, March 2014, Pages 80–88