کد مقاله | کد نشریه | سال انتشار | مقاله انگلیسی | نسخه تمام متن |
---|---|---|---|---|
496202 | 862851 | 2012 | 10 صفحه PDF | دانلود رایگان |
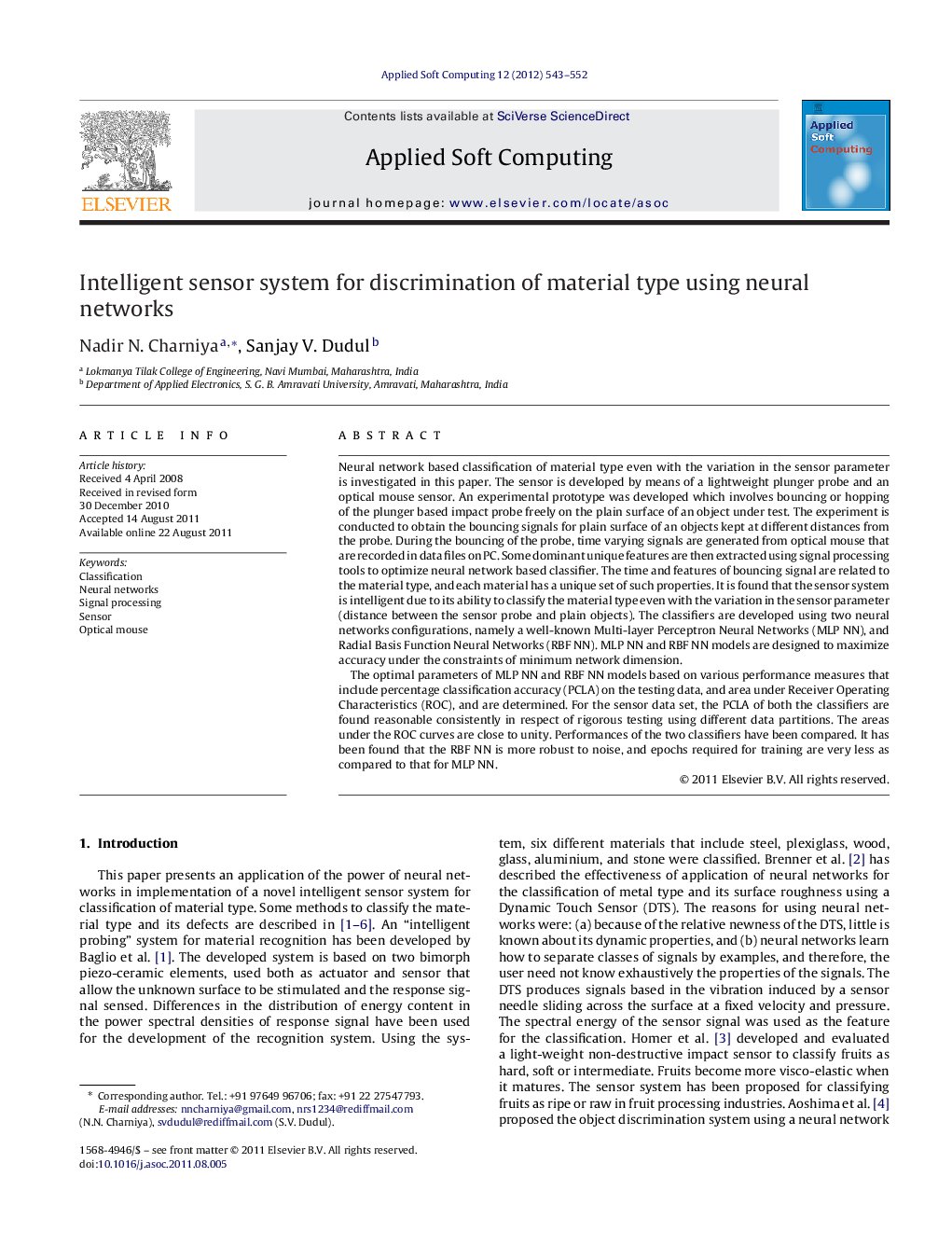
Neural network based classification of material type even with the variation in the sensor parameter is investigated in this paper. The sensor is developed by means of a lightweight plunger probe and an optical mouse sensor. An experimental prototype was developed which involves bouncing or hopping of the plunger based impact probe freely on the plain surface of an object under test. The experiment is conducted to obtain the bouncing signals for plain surface of an objects kept at different distances from the probe. During the bouncing of the probe, time varying signals are generated from optical mouse that are recorded in data files on PC. Some dominant unique features are then extracted using signal processing tools to optimize neural network based classifier. The time and features of bouncing signal are related to the material type, and each material has a unique set of such properties. It is found that the sensor system is intelligent due to its ability to classify the material type even with the variation in the sensor parameter (distance between the sensor probe and plain objects). The classifiers are developed using two neural networks configurations, namely a well-known Multi-layer Perceptron Neural Networks (MLP NN), and Radial Basis Function Neural Networks (RBF NN). MLP NN and RBF NN models are designed to maximize accuracy under the constraints of minimum network dimension.The optimal parameters of MLP NN and RBF NN models based on various performance measures that include percentage classification accuracy (PCLA) on the testing data, and area under Receiver Operating Characteristics (ROC), and are determined. For the sensor data set, the PCLA of both the classifiers are found reasonable consistently in respect of rigorous testing using different data partitions. The areas under the ROC curves are close to unity. Performances of the two classifiers have been compared. It has been found that the RBF NN is more robust to noise, and epochs required for training are very less as compared to that for MLP NN.
► Design of intelligent sensor system for classification of material type.
► Near-optimal design of neural networks based classifiers.
► Comparison of the classifiers on the basis of accuracy and robustness to noise.
► Radial basis function neural network was found more robust to noise.
Journal: Applied Soft Computing - Volume 12, Issue 1, January 2012, Pages 543–552