کد مقاله | کد نشریه | سال انتشار | مقاله انگلیسی | نسخه تمام متن |
---|---|---|---|---|
496833 | 862872 | 2009 | 6 صفحه PDF | دانلود رایگان |
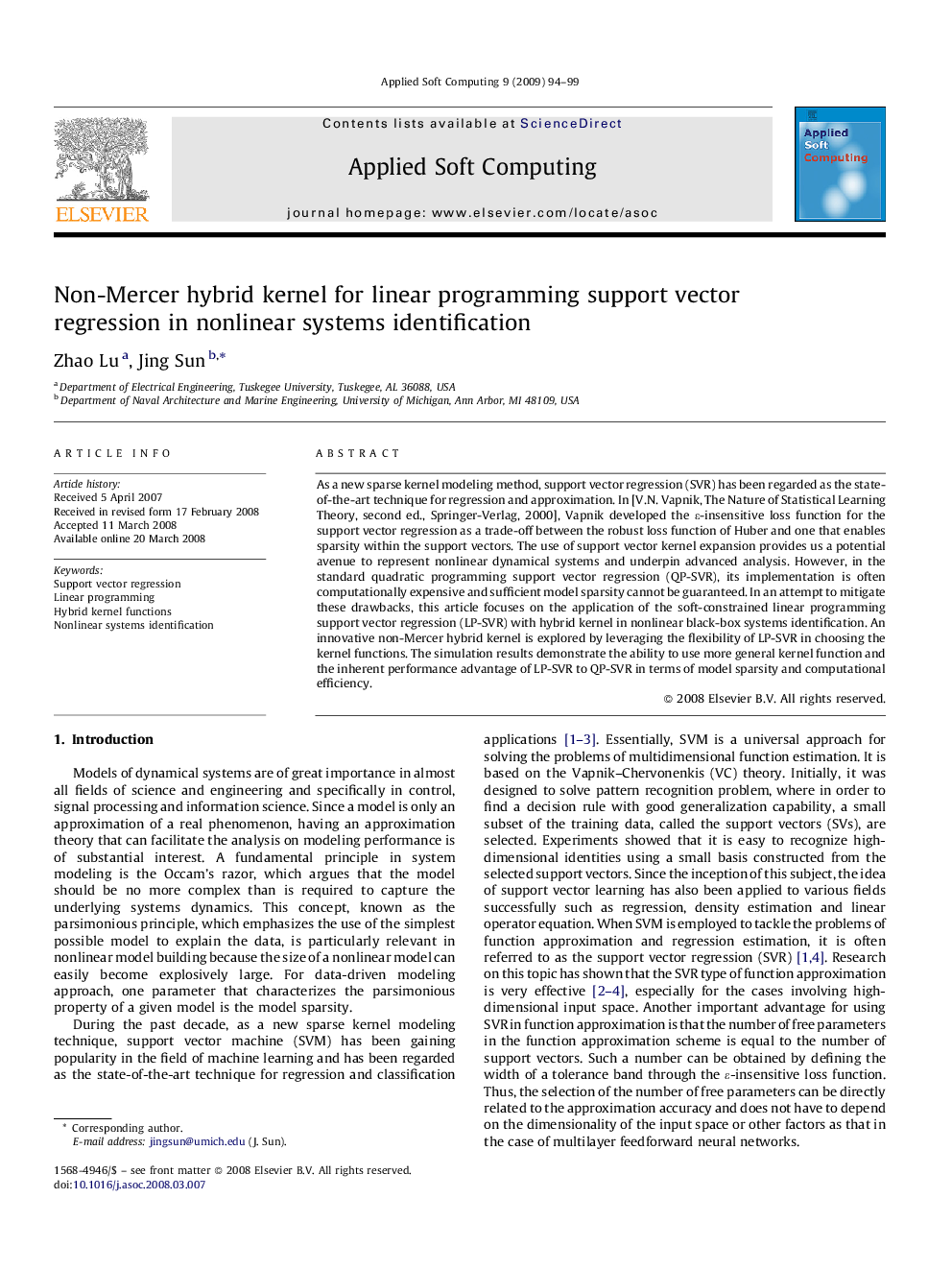
As a new sparse kernel modeling method, support vector regression (SVR) has been regarded as the state-of-the-art technique for regression and approximation. In [V.N. Vapnik, The Nature of Statistical Learning Theory, second ed., Springer-Verlag, 2000], Vapnik developed the ɛ-insensitive loss function for the support vector regression as a trade-off between the robust loss function of Huber and one that enables sparsity within the support vectors. The use of support vector kernel expansion provides us a potential avenue to represent nonlinear dynamical systems and underpin advanced analysis. However, in the standard quadratic programming support vector regression (QP-SVR), its implementation is often computationally expensive and sufficient model sparsity cannot be guaranteed. In an attempt to mitigate these drawbacks, this article focuses on the application of the soft-constrained linear programming support vector regression (LP-SVR) with hybrid kernel in nonlinear black-box systems identification. An innovative non-Mercer hybrid kernel is explored by leveraging the flexibility of LP-SVR in choosing the kernel functions. The simulation results demonstrate the ability to use more general kernel function and the inherent performance advantage of LP-SVR to QP-SVR in terms of model sparsity and computational efficiency.
Journal: Applied Soft Computing - Volume 9, Issue 1, January 2009, Pages 94–99