کد مقاله | کد نشریه | سال انتشار | مقاله انگلیسی | نسخه تمام متن |
---|---|---|---|---|
4969518 | 1449977 | 2017 | 34 صفحه PDF | دانلود رایگان |
عنوان انگلیسی مقاله ISI
Higher order spectral regression discriminant analysis (HOSRDA): A tensor feature reduction method for ERP detection
دانلود مقاله + سفارش ترجمه
دانلود مقاله ISI انگلیسی
رایگان برای ایرانیان
کلمات کلیدی
موضوعات مرتبط
مهندسی و علوم پایه
مهندسی کامپیوتر
چشم انداز کامپیوتر و تشخیص الگو
پیش نمایش صفحه اول مقاله
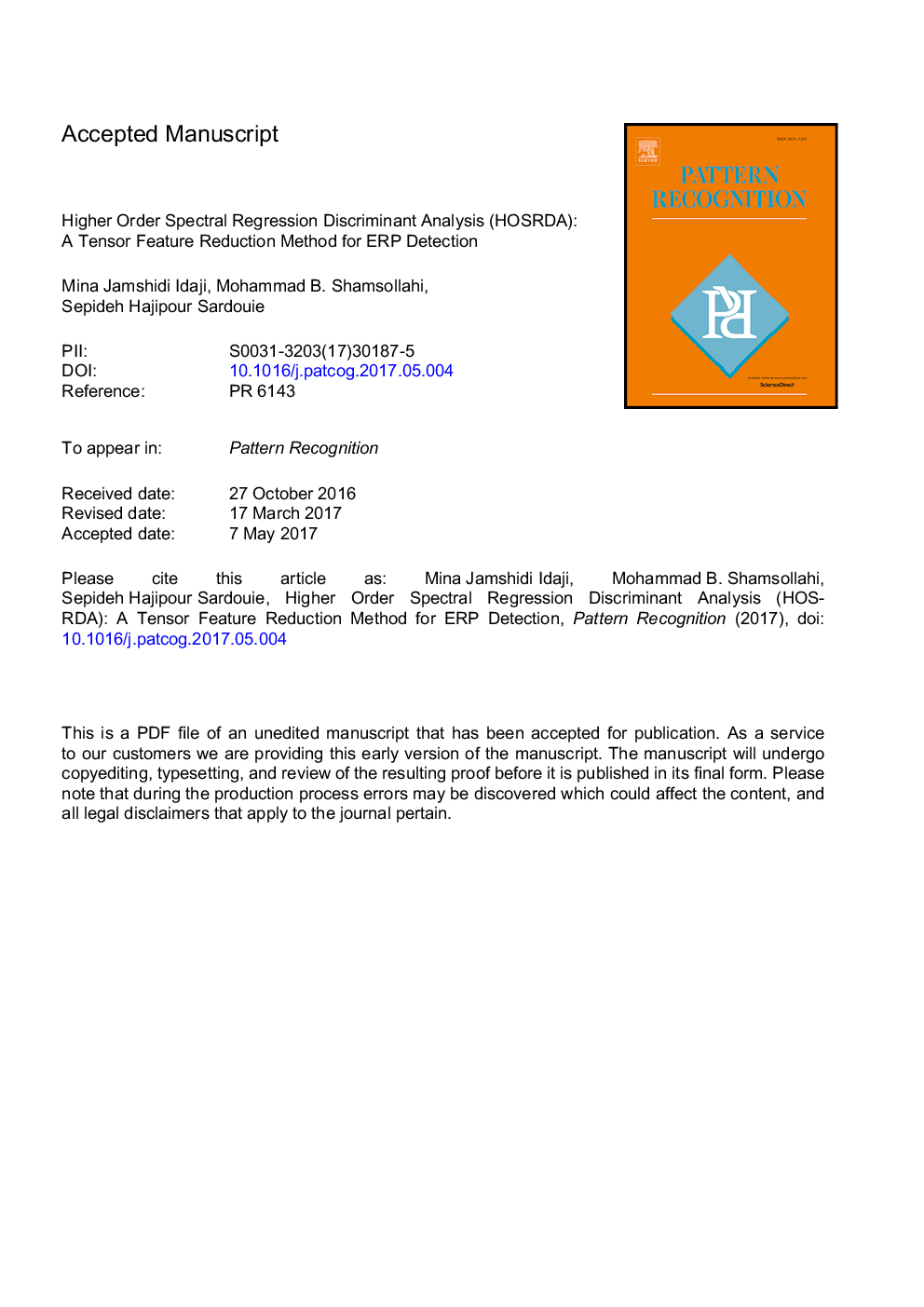
چکیده انگلیسی
Tensors are valuable tools to represent Electroencephalogram (EEG) data. Tucker decomposition is the most used tensor decomposition in multidimensional discriminant analysis and tensor extension of Linear Discriminant Analysis (LDA), called Higher Order Discriminant Analysis (HODA), is a popular tensor discriminant method used for analyzing Event Related Potentials (ERP). In this paper, we introduce a new tensor-based feature reduction technique, named Higher Order Spectral Regression Discriminant Analysis (HOSRDA), for use in a classification framework for ERP detection. The proposed method (HOSRDA) is a tensor extension of Spectral Regression Discriminant Analysis (SRDA) and casts the eigenproblem of HODA to a regression problem. The formulation of HOSRDA can open a new framework for adding different regularization constraints in higher order feature reduction problem. Additionally, when the dimension and number of samples is very large, the regression problem can be solved via efficient iterative algorithms. We applied HOSRDA on data of a P300 speller from BCI competition III and reached average character detection accuracy of 96.5% for the two subjects. HOSRDA outperforms almost all of other reported methods on this dataset. Additionally, the results of our method are fairly comparable with those of other methods when 5 and 10 repetitions are used in the P300 speller paradigm.
ناشر
Database: Elsevier - ScienceDirect (ساینس دایرکت)
Journal: Pattern Recognition - Volume 70, October 2017, Pages 152-162
Journal: Pattern Recognition - Volume 70, October 2017, Pages 152-162
نویسندگان
Mina Jamshidi Idaji, Mohammad B. Shamsollahi, Sepideh Hajipour Sardouie,