کد مقاله | کد نشریه | سال انتشار | مقاله انگلیسی | نسخه تمام متن |
---|---|---|---|---|
4969754 | 1449985 | 2017 | 48 صفحه PDF | دانلود رایگان |
عنوان انگلیسی مقاله ISI
Modification of supervised OPF-based intrusion detection systems using unsupervised learning and social network concept
دانلود مقاله + سفارش ترجمه
دانلود مقاله ISI انگلیسی
رایگان برای ایرانیان
کلمات کلیدی
موضوعات مرتبط
مهندسی و علوم پایه
مهندسی کامپیوتر
چشم انداز کامپیوتر و تشخیص الگو
پیش نمایش صفحه اول مقاله
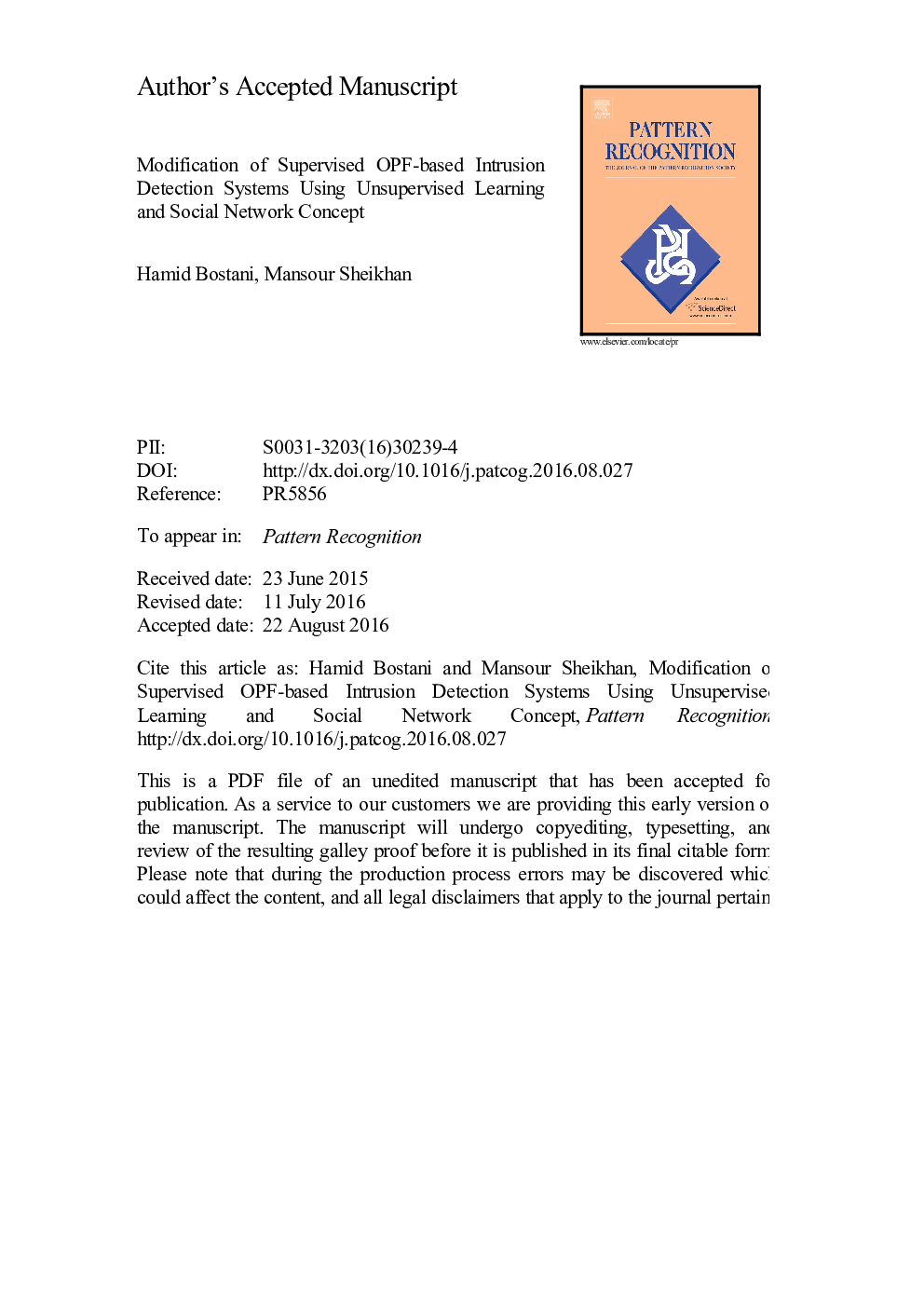
چکیده انگلیسی
Optimum-path forest (OPF) is a graph-based machine learning method that can overcome some limitations of the traditional machine learning algorithms that have been used in intrusion detection systems. This paper presents a novel approach for intrusion detection using a modified OPF (MOPF) algorithm for improving the performance of traditional OPF in terms of detection rate (DR), false alarm rate (FAR), and time of execution. To address the problem of scalability in large datasets and also for achieving high attack recognition rates, the proposed framework employs the k-means clustering algorithm, as a partitioning module, for generating different homogeneous training subsets from original heterogeneous training samples. In the proposed MOPF algorithm, the distance between unlabeled samples and the root (prototype) of every sample in OPF is also considered in classifying unlabeled samples with the aim of improving the accuracy rate of traditional OPF algorithm. Moreover, the centrality and the prestige concepts in the social network analysis are employed in a pruning module for determining the most informative samples in training subsets to speed up the traditional OPF algorithm. The experimental results on NSL-KDD dataset show that the proposed method performs better than traditional OPF in terms of accuracy rate, DR, FAR, and cost per example (CPE) evaluation metrics.
ناشر
Database: Elsevier - ScienceDirect (ساینس دایرکت)
Journal: Pattern Recognition - Volume 62, February 2017, Pages 56-72
Journal: Pattern Recognition - Volume 62, February 2017, Pages 56-72
نویسندگان
Hamid Bostani, Mansour Sheikhan,